Spaces:
Running
Running
Upload 141 files
Browse filesThis view is limited to 50 files because it contains too many changes.
See raw diff
- .gitattributes +50 -0
- .gitignore +8 -0
- LICENSE +21 -0
- README.md +78 -0
- app.py +86 -0
- requirements.txt +26 -0
- src/audeo/Midi_synth.py +165 -0
- src/audeo/README.md +67 -0
- src/audeo/Roll2MidiNet.py +139 -0
- src/audeo/Roll2MidiNet_enhance.py +164 -0
- src/audeo/Roll2Midi_dataset.py +160 -0
- src/audeo/Roll2Midi_dataset_tv2a_eval.py +118 -0
- src/audeo/Roll2Midi_evaluate.py +126 -0
- src/audeo/Roll2Midi_evaluate_tv2a.py +93 -0
- src/audeo/Roll2Midi_inference.py +100 -0
- src/audeo/Roll2Midi_train.py +280 -0
- src/audeo/Video2RollNet.py +264 -0
- src/audeo/Video2Roll_dataset.py +148 -0
- src/audeo/Video2Roll_evaluate.py +90 -0
- src/audeo/Video2Roll_inference.py +151 -0
- src/audeo/Video2Roll_solver.py +204 -0
- src/audeo/Video2Roll_train.py +26 -0
- src/audeo/Video_Id.md +30 -0
- src/audeo/balance_data.py +91 -0
- src/audeo/models/Video2Roll_50_0.4/14.pth +3 -0
- src/audeo/piano_coords.py +9 -0
- src/audeo/thumbnail_image.png +3 -0
- src/audeo/videomae_fintune.ipynb +0 -0
- src/audioldm/__init__.py +8 -0
- src/audioldm/__main__.py +183 -0
- src/audioldm/audio/__init__.py +2 -0
- src/audioldm/audio/audio_processing.py +100 -0
- src/audioldm/audio/stft.py +186 -0
- src/audioldm/audio/tools.py +85 -0
- src/audioldm/clap/__init__.py +0 -0
- src/audioldm/clap/encoders.py +170 -0
- src/audioldm/clap/open_clip/__init__.py +25 -0
- src/audioldm/clap/open_clip/bert.py +40 -0
- src/audioldm/clap/open_clip/bpe_simple_vocab_16e6.txt.gz +3 -0
- src/audioldm/clap/open_clip/factory.py +279 -0
- src/audioldm/clap/open_clip/feature_fusion.py +192 -0
- src/audioldm/clap/open_clip/htsat.py +1308 -0
- src/audioldm/clap/open_clip/linear_probe.py +66 -0
- src/audioldm/clap/open_clip/loss.py +398 -0
- src/audioldm/clap/open_clip/model.py +936 -0
- src/audioldm/clap/open_clip/model_configs/HTSAT-base.json +23 -0
- src/audioldm/clap/open_clip/model_configs/HTSAT-large.json +23 -0
- src/audioldm/clap/open_clip/model_configs/HTSAT-tiny-win-1536.json +23 -0
- src/audioldm/clap/open_clip/model_configs/HTSAT-tiny.json +23 -0
- src/audioldm/clap/open_clip/model_configs/PANN-10.json +23 -0
.gitattributes
ADDED
@@ -0,0 +1,50 @@
|
|
|
|
|
|
|
|
|
|
|
|
|
|
|
|
|
|
|
|
|
|
|
|
|
|
|
|
|
|
|
|
|
|
|
|
|
|
|
|
|
|
|
|
|
|
|
|
|
|
|
|
|
|
|
|
|
|
|
|
|
|
|
|
|
|
|
|
|
|
|
|
|
|
|
|
|
|
|
|
|
|
|
|
|
|
|
|
|
|
|
|
|
|
|
|
|
|
|
|
|
|
|
1 |
+
*.7z filter=lfs diff=lfs merge=lfs -text
|
2 |
+
*.arrow filter=lfs diff=lfs merge=lfs -text
|
3 |
+
*.bin filter=lfs diff=lfs merge=lfs -text
|
4 |
+
*.bz2 filter=lfs diff=lfs merge=lfs -text
|
5 |
+
*.ckpt filter=lfs diff=lfs merge=lfs -text
|
6 |
+
*.ftz filter=lfs diff=lfs merge=lfs -text
|
7 |
+
*.gz filter=lfs diff=lfs merge=lfs -text
|
8 |
+
*.h5 filter=lfs diff=lfs merge=lfs -text
|
9 |
+
*.joblib filter=lfs diff=lfs merge=lfs -text
|
10 |
+
*.lfs.* filter=lfs diff=lfs merge=lfs -text
|
11 |
+
*.mlmodel filter=lfs diff=lfs merge=lfs -text
|
12 |
+
*.model filter=lfs diff=lfs merge=lfs -text
|
13 |
+
*.msgpack filter=lfs diff=lfs merge=lfs -text
|
14 |
+
*.npy filter=lfs diff=lfs merge=lfs -text
|
15 |
+
*.npz filter=lfs diff=lfs merge=lfs -text
|
16 |
+
*.onnx filter=lfs diff=lfs merge=lfs -text
|
17 |
+
*.ot filter=lfs diff=lfs merge=lfs -text
|
18 |
+
*.parquet filter=lfs diff=lfs merge=lfs -text
|
19 |
+
*.pb filter=lfs diff=lfs merge=lfs -text
|
20 |
+
*.pickle filter=lfs diff=lfs merge=lfs -text
|
21 |
+
*.pkl filter=lfs diff=lfs merge=lfs -text
|
22 |
+
*.pt filter=lfs diff=lfs merge=lfs -text
|
23 |
+
*.pth filter=lfs diff=lfs merge=lfs -text
|
24 |
+
*.rar filter=lfs diff=lfs merge=lfs -text
|
25 |
+
*.safetensors filter=lfs diff=lfs merge=lfs -text
|
26 |
+
saved_model/**/* filter=lfs diff=lfs merge=lfs -text
|
27 |
+
*.tar.* filter=lfs diff=lfs merge=lfs -text
|
28 |
+
*.tar filter=lfs diff=lfs merge=lfs -text
|
29 |
+
*.tflite filter=lfs diff=lfs merge=lfs -text
|
30 |
+
*.tgz filter=lfs diff=lfs merge=lfs -text
|
31 |
+
*.wasm filter=lfs diff=lfs merge=lfs -text
|
32 |
+
*.xz filter=lfs diff=lfs merge=lfs -text
|
33 |
+
*.zip filter=lfs diff=lfs merge=lfs -text
|
34 |
+
*.zst filter=lfs diff=lfs merge=lfs -text
|
35 |
+
*tfevents* filter=lfs diff=lfs merge=lfs -text
|
36 |
+
Video-to-Audio-and-Piano-HF/src/audeo/thumbnail_image.png filter=lfs diff=lfs merge=lfs -text
|
37 |
+
Video-to-Audio-and-Piano-HF/tests/piano_2h_cropped2_cuts/nwwHuxHMIpc.00000000.mp4 filter=lfs diff=lfs merge=lfs -text
|
38 |
+
Video-to-Audio-and-Piano-HF/tests/piano_2h_cropped2_cuts/nwwHuxHMIpc.00000001.mp4 filter=lfs diff=lfs merge=lfs -text
|
39 |
+
Video-to-Audio-and-Piano-HF/tests/scps/tango-master/data/audiocaps/train_audiocaps.json filter=lfs diff=lfs merge=lfs -text
|
40 |
+
Video-to-Audio-and-Piano-HF/tests/scps/tango-master/data/train_audioset_sl.json filter=lfs diff=lfs merge=lfs -text
|
41 |
+
Video-to-Audio-and-Piano-HF/tests/scps/tango-master/data/train_bbc_sound_effects.json filter=lfs diff=lfs merge=lfs -text
|
42 |
+
Video-to-Audio-and-Piano-HF/tests/scps/tango-master/data/train_val_audioset_sl.json filter=lfs diff=lfs merge=lfs -text
|
43 |
+
Video-to-Audio-and-Piano-HF/tests/scps/VGGSound/train.scp filter=lfs diff=lfs merge=lfs -text
|
44 |
+
Video-to-Audio-and-Piano-HF/tests/VGGSound/video/1u1orBeV4xI_000428.mp4 filter=lfs diff=lfs merge=lfs -text
|
45 |
+
Video-to-Audio-and-Piano-HF/tests/VGGSound/video/1uCzQCdCC1U_000170.mp4 filter=lfs diff=lfs merge=lfs -text
|
46 |
+
src/audeo/thumbnail_image.png filter=lfs diff=lfs merge=lfs -text
|
47 |
+
tests/piano_2h_cropped2_cuts/nwwHuxHMIpc.00000000.mp4 filter=lfs diff=lfs merge=lfs -text
|
48 |
+
tests/piano_2h_cropped2_cuts/nwwHuxHMIpc.00000001.mp4 filter=lfs diff=lfs merge=lfs -text
|
49 |
+
tests/VGGSound/video/1u1orBeV4xI_000428.mp4 filter=lfs diff=lfs merge=lfs -text
|
50 |
+
tests/VGGSound/video/1uCzQCdCC1U_000170.mp4 filter=lfs diff=lfs merge=lfs -text
|
.gitignore
ADDED
@@ -0,0 +1,8 @@
|
|
|
|
|
|
|
|
|
|
|
|
|
|
|
|
|
|
|
1 |
+
**/__pycache__
|
2 |
+
src/audeo/data/
|
3 |
+
ckpts/
|
4 |
+
outputs/
|
5 |
+
outputs_piano/
|
6 |
+
outputs_vgg/
|
7 |
+
src/train*
|
8 |
+
src/inference3*
|
LICENSE
ADDED
@@ -0,0 +1,21 @@
|
|
|
|
|
|
|
|
|
|
|
|
|
|
|
|
|
|
|
|
|
|
|
|
|
|
|
|
|
|
|
|
|
|
|
|
|
|
|
|
|
|
|
|
|
1 |
+
MIT License
|
2 |
+
|
3 |
+
Copyright (c) 2024 Phil Wang
|
4 |
+
|
5 |
+
Permission is hereby granted, free of charge, to any person obtaining a copy
|
6 |
+
of this software and associated documentation files (the "Software"), to deal
|
7 |
+
in the Software without restriction, including without limitation the rights
|
8 |
+
to use, copy, modify, merge, publish, distribute, sublicense, and/or sell
|
9 |
+
copies of the Software, and to permit persons to whom the Software is
|
10 |
+
furnished to do so, subject to the following conditions:
|
11 |
+
|
12 |
+
The above copyright notice and this permission notice shall be included in all
|
13 |
+
copies or substantial portions of the Software.
|
14 |
+
|
15 |
+
THE SOFTWARE IS PROVIDED "AS IS", WITHOUT WARRANTY OF ANY KIND, EXPRESS OR
|
16 |
+
IMPLIED, INCLUDING BUT NOT LIMITED TO THE WARRANTIES OF MERCHANTABILITY,
|
17 |
+
FITNESS FOR A PARTICULAR PURPOSE AND NONINFRINGEMENT. IN NO EVENT SHALL THE
|
18 |
+
AUTHORS OR COPYRIGHT HOLDERS BE LIABLE FOR ANY CLAIM, DAMAGES OR OTHER
|
19 |
+
LIABILITY, WHETHER IN AN ACTION OF CONTRACT, TORT OR OTHERWISE, ARISING FROM,
|
20 |
+
OUT OF OR IN CONNECTION WITH THE SOFTWARE OR THE USE OR OTHER DEALINGS IN THE
|
21 |
+
SOFTWARE.
|
README.md
ADDED
@@ -0,0 +1,78 @@
|
|
|
|
|
|
|
|
|
|
|
|
|
|
|
|
|
|
|
|
|
|
|
|
|
|
|
|
|
|
|
|
|
|
|
|
|
|
|
|
|
|
|
|
|
|
|
|
|
|
|
|
|
|
|
|
|
|
|
|
|
|
|
|
|
|
|
|
|
|
|
|
|
|
|
|
|
|
|
|
|
|
|
|
|
|
|
|
|
|
|
|
|
|
|
|
|
|
|
|
|
|
|
|
|
|
|
|
|
|
|
|
|
|
|
|
|
|
|
|
|
|
|
|
|
|
|
|
|
|
|
|
|
|
|
|
|
|
|
|
|
|
|
|
|
|
|
|
|
|
|
|
|
|
|
|
|
|
|
1 |
+
---
|
2 |
+
title: DeepAudio-V1
|
3 |
+
emoji: 🔊
|
4 |
+
colorFrom: blue
|
5 |
+
colorTo: indigo
|
6 |
+
sdk: gradio
|
7 |
+
app_file: app.py
|
8 |
+
pinned: false
|
9 |
+
---
|
10 |
+
|
11 |
+
|
12 |
+
## Enhance Generation Quality of Flow Matching V2A Model via Multi-Step CoT-Like Guidance and Combined Preference Optimization
|
13 |
+
## Towards Video to Piano Music Generation with Chain-of-Perform Support Benchmarks
|
14 |
+
|
15 |
+
## Results
|
16 |
+
|
17 |
+
**1. Results of Video-to-Audio Synthesis**
|
18 |
+
|
19 |
+
https://github.com/user-attachments/assets/d6761371-8fc2-427c-8b2b-6d2ac22a2db2
|
20 |
+
|
21 |
+
https://github.com/user-attachments/assets/50b33e54-8ba1-4fab-89d3-5a5cc4c22c9a
|
22 |
+
|
23 |
+
**2. Results of Video-to-Piano Synthesis**
|
24 |
+
|
25 |
+
https://github.com/user-attachments/assets/b6218b94-1d58-4dc5-873a-c3e8eef6cd67
|
26 |
+
|
27 |
+
https://github.com/user-attachments/assets/ebdd1d95-2d9e-4add-b61a-d181f0ae38d0
|
28 |
+
|
29 |
+
|
30 |
+
## Installation
|
31 |
+
|
32 |
+
**1. Create a conda environment**
|
33 |
+
|
34 |
+
```bash
|
35 |
+
conda create -n v2ap python=3.10
|
36 |
+
conda activate v2ap
|
37 |
+
```
|
38 |
+
|
39 |
+
**2. Install requirements**
|
40 |
+
|
41 |
+
```bash
|
42 |
+
pip install -r requirements.txt
|
43 |
+
```
|
44 |
+
|
45 |
+
|
46 |
+
**Pretrained models**
|
47 |
+
|
48 |
+
The models are available at https://huggingface.co/lshzhm/Video-to-Audio-and-Piano/tree/main.
|
49 |
+
|
50 |
+
|
51 |
+
## Inference
|
52 |
+
|
53 |
+
**1. Video-to-Audio inference**
|
54 |
+
|
55 |
+
```bash
|
56 |
+
python src/inference_v2a.py
|
57 |
+
```
|
58 |
+
|
59 |
+
**2. Video-to-Piano inference**
|
60 |
+
|
61 |
+
```bash
|
62 |
+
python src/inference_v2p.py
|
63 |
+
```
|
64 |
+
|
65 |
+
## Dateset is in progress
|
66 |
+
|
67 |
+
|
68 |
+
## Metrix
|
69 |
+
|
70 |
+
|
71 |
+
## Acknowledgement
|
72 |
+
|
73 |
+
- [Audeo](https://github.com/shlizee/Audeo) for video to midi prediction
|
74 |
+
- [E2TTS](https://github.com/lucidrains/e2-tts-pytorch) for CFM structure and base E2 implementation
|
75 |
+
- [FLAN-T5](https://huggingface.co/google/flan-t5-large) for FLAN-T5 text encode
|
76 |
+
- [CLIP](https://huggingface.co/laion/CLIP-ViT-bigG-14-laion2B-39B-b160k) for CLIP image encode
|
77 |
+
- [AudioLDM Eval](https://github.com/haoheliu/audioldm_eval) for audio evaluation
|
78 |
+
|
app.py
ADDED
@@ -0,0 +1,86 @@
|
|
|
|
|
|
|
|
|
|
|
|
|
|
|
|
|
|
|
|
|
|
|
|
|
|
|
|
|
|
|
|
|
|
|
|
|
|
|
|
|
|
|
|
|
|
|
|
|
|
|
|
|
|
|
|
|
|
|
|
|
|
|
|
|
|
|
|
|
|
|
|
|
|
|
|
|
|
|
|
|
|
|
|
|
|
|
|
|
|
|
|
|
|
|
|
|
|
|
|
|
|
|
|
|
|
|
|
|
|
|
|
|
|
|
|
|
|
|
|
|
|
|
|
|
|
|
|
|
|
|
|
|
|
|
|
|
|
|
|
|
|
|
|
|
|
|
|
|
|
|
|
|
|
|
|
|
|
|
|
|
|
|
|
|
|
|
|
|
|
|
|
|
|
|
1 |
+
import os
|
2 |
+
try:
|
3 |
+
import torchaudio
|
4 |
+
except ImportError:
|
5 |
+
os.system("cd ./F5-TTS; pip install -e .")
|
6 |
+
|
7 |
+
|
8 |
+
import spaces
|
9 |
+
import logging
|
10 |
+
from datetime import datetime
|
11 |
+
from pathlib import Path
|
12 |
+
|
13 |
+
import gradio as gr
|
14 |
+
import torch
|
15 |
+
import torchaudio
|
16 |
+
|
17 |
+
import tempfile
|
18 |
+
|
19 |
+
import requests
|
20 |
+
import shutil
|
21 |
+
import numpy as np
|
22 |
+
|
23 |
+
from huggingface_hub import hf_hub_download
|
24 |
+
|
25 |
+
model_path = "./ckpts/"
|
26 |
+
|
27 |
+
if not os.path.exists(model_path):
|
28 |
+
os.makedirs(model_path)
|
29 |
+
|
30 |
+
file_path = hf_hub_download(repo_id="lshzhm/Video-to-Audio-and-Piano", local_dir=model_path)
|
31 |
+
|
32 |
+
print(f"Model saved at: {file_path}")
|
33 |
+
|
34 |
+
log = logging.getLogger()
|
35 |
+
|
36 |
+
|
37 |
+
#@spaces.GPU(duration=120)
|
38 |
+
def video_to_audio(video: gr.Video, prompt: str, num_steps: int):
|
39 |
+
|
40 |
+
|
41 |
+
return video_save_path, video_gen
|
42 |
+
|
43 |
+
|
44 |
+
def video_to_piano(video: gr.Video, prompt: str, num_steps: int):
|
45 |
+
|
46 |
+
return video_save_path, video_gen
|
47 |
+
|
48 |
+
|
49 |
+
video_to_audio_and_speech_tab = gr.Interface(
|
50 |
+
fn=video_to_audio_and_speech,
|
51 |
+
description="""
|
52 |
+
Project page: <a href="https://acappemin.github.io/DeepAudio-V1.github.io">https://acappemin.github.io/DeepAudio-V1.github.io</a><br>
|
53 |
+
Code: <a href="https://github.com/acappemin/DeepAudio-V1">https://github.com/acappemin/DeepAudio-V1</a><br>
|
54 |
+
""",
|
55 |
+
inputs=[
|
56 |
+
gr.Video(label="Input Video"),
|
57 |
+
gr.Text(label='Video-to-Audio Text Prompt'),
|
58 |
+
gr.Number(label='Video-to-Audio Num Steps', value=64, precision=0, minimum=1),
|
59 |
+
gr.Text(label='Video-to-Speech Transcription'),
|
60 |
+
gr.Audio(label='Video-to-Speech Speech Prompt'),
|
61 |
+
gr.Text(label='Video-to-Speech Speech Prompt Transcription'),
|
62 |
+
gr.Number(label='Video-to-Speech Num Steps', value=64, precision=0, minimum=1),
|
63 |
+
],
|
64 |
+
outputs=[
|
65 |
+
gr.Video(label="Video-to-Audio Output"),
|
66 |
+
gr.Video(label="Video-to-Speech Output"),
|
67 |
+
],
|
68 |
+
cache_examples=False,
|
69 |
+
title='Video-to-Audio-and-Speech',
|
70 |
+
examples=[
|
71 |
+
[
|
72 |
+
'./tests/VGGSound/video/1u1orBeV4xI_000428.mp4',
|
73 |
+
'',
|
74 |
+
64,
|
75 |
+
],
|
76 |
+
[
|
77 |
+
'./tests/VGGSound/video/1uCzQCdCC1U_000170.mp4',
|
78 |
+
'',
|
79 |
+
64,
|
80 |
+
],
|
81 |
+
])
|
82 |
+
|
83 |
+
|
84 |
+
if __name__ == "__main__":
|
85 |
+
gr.TabbedInterface([video_to_audio_and_speech_tab], ['Video-to-Audio-and-Speech']).launch()
|
86 |
+
|
requirements.txt
ADDED
@@ -0,0 +1,26 @@
|
|
|
|
|
|
|
|
|
|
|
|
|
|
|
|
|
|
|
|
|
|
|
|
|
|
|
|
|
|
|
|
|
|
|
|
|
|
|
|
|
|
|
|
|
|
|
|
|
|
|
|
|
|
|
1 |
+
accelerate==0.34.2
|
2 |
+
beartype==0.18.5
|
3 |
+
einops==0.8.0
|
4 |
+
einx==0.3.0
|
5 |
+
ema-pytorch==0.6.2
|
6 |
+
g2p-en==2.1.0
|
7 |
+
jaxtyping==0.2.34
|
8 |
+
loguru==0.7.2
|
9 |
+
tensorboard==2.18.0
|
10 |
+
torch==2.4.1
|
11 |
+
torchaudio==2.4.1
|
12 |
+
torchdiffeq==0.2.4
|
13 |
+
torchlibrosa==0.1.0
|
14 |
+
torchmetrics==1.6.1
|
15 |
+
torchvision==0.19.1
|
16 |
+
numpy==1.23.5
|
17 |
+
tqdm==4.66.5
|
18 |
+
vocos==0.1.0
|
19 |
+
x-transformers==1.37.4
|
20 |
+
transformers==4.46.0
|
21 |
+
moviepy==1.0.3
|
22 |
+
jieba==0.42.1
|
23 |
+
pypinyin==0.44.0
|
24 |
+
progressbar==2.5
|
25 |
+
datasets==3.0.1
|
26 |
+
matplotlib==3.9.2
|
src/audeo/Midi_synth.py
ADDED
@@ -0,0 +1,165 @@
|
|
|
|
|
|
|
|
|
|
|
|
|
|
|
|
|
|
|
|
|
|
|
|
|
|
|
|
|
|
|
|
|
|
|
|
|
|
|
|
|
|
|
|
|
|
|
|
|
|
|
|
|
|
|
|
|
|
|
|
|
|
|
|
|
|
|
|
|
|
|
|
|
|
|
|
|
|
|
|
|
|
|
|
|
|
|
|
|
|
|
|
|
|
|
|
|
|
|
|
|
|
|
|
|
|
|
|
|
|
|
|
|
|
|
|
|
|
|
|
|
|
|
|
|
|
|
|
|
|
|
|
|
|
|
|
|
|
|
|
|
|
|
|
|
|
|
|
|
|
|
|
|
|
|
|
|
|
|
|
|
|
|
|
|
|
|
|
|
|
|
|
|
|
|
|
|
|
|
|
|
|
|
|
|
|
|
|
|
|
|
|
|
|
|
|
|
|
|
|
|
|
|
|
|
|
|
|
|
|
|
|
|
|
|
|
|
|
|
|
|
|
|
|
|
|
|
|
|
|
|
|
|
|
|
|
|
|
|
|
|
|
|
|
|
|
|
|
|
|
|
|
|
|
|
|
|
|
|
|
|
|
|
|
|
|
|
|
|
|
|
|
|
|
|
|
|
|
|
|
|
|
|
|
|
|
|
|
|
|
|
|
|
|
|
|
|
|
|
|
|
|
|
|
|
|
|
|
|
|
|
|
|
|
|
|
|
|
|
|
|
|
|
|
|
|
|
|
|
|
|
|
|
1 |
+
import os
|
2 |
+
import numpy as np
|
3 |
+
os.environ["LD_PRELOAD"] = "/usr/lib/x86_64-linux-gnu/libffi.so.7"
|
4 |
+
import pretty_midi
|
5 |
+
import glob
|
6 |
+
import librosa
|
7 |
+
import soundfile as sf
|
8 |
+
|
9 |
+
# Synthesizing Audio using Fluid Synth
|
10 |
+
class MIDISynth():
|
11 |
+
def __init__(self, out_folder, video_name, instrument, midi=True):
|
12 |
+
self.video_name = video_name
|
13 |
+
# synthesize midi or roll
|
14 |
+
self.midi = False
|
15 |
+
# synthsized output dir, change to your own path
|
16 |
+
self.syn_dir = '/ailab-train/speech/shansizhe/audeo/data/Midi_Synth/training/'
|
17 |
+
self.min_key = 15
|
18 |
+
self.max_key = 65
|
19 |
+
self.frame = 50
|
20 |
+
self.piano_keys = 88
|
21 |
+
if self.midi:
|
22 |
+
self.midi_out_folder = out_folder + video_name
|
23 |
+
self.syn_dir = self.syn_dir + 'w_Roll2Midi/'
|
24 |
+
self.process_midi()
|
25 |
+
else:
|
26 |
+
self.est_roll_folder = out_folder + video_name
|
27 |
+
self.syn_dir = self.syn_dir + 'wo_Roll2Midi/'
|
28 |
+
self.process_roll()
|
29 |
+
self.spf = 0.04 # second per frame
|
30 |
+
self.sample_rate = 16000
|
31 |
+
self.ins = instrument
|
32 |
+
|
33 |
+
def process_roll(self):
|
34 |
+
self.wo_Roll2Midi_data = []
|
35 |
+
self.est_roll_files = glob.glob(self.est_roll_folder + '/*.npz')
|
36 |
+
self.est_roll_files.sort(key=lambda x: int(x.split('/')[-1].split('.')[0].split('-')[0]))
|
37 |
+
|
38 |
+
# Use the Roll prediction for Synthesis
|
39 |
+
print("need to process {0} files".format(len(self.est_roll_folder)))
|
40 |
+
for i in range(len(self.est_roll_files)):
|
41 |
+
with np.load(self.est_roll_files[i]) as data:
|
42 |
+
est_roll = data['roll']
|
43 |
+
if est_roll.shape[0] != self.frame:
|
44 |
+
target = np.zeros((self.frame, self.piano_keys))
|
45 |
+
target[:est_roll.shape[0], :] = est_roll
|
46 |
+
est_roll = target
|
47 |
+
est_roll = np.where(est_roll > 0, 1, 0)
|
48 |
+
self.wo_Roll2Midi_data.append(est_roll)
|
49 |
+
self.complete_wo_Roll2Midi_midi = np.concatenate(self.wo_Roll2Midi_data)
|
50 |
+
print("Without Roll2MidiNet, the Roll result has shape:", self.complete_wo_Roll2Midi_midi.shape)
|
51 |
+
# compute onsets and offsets
|
52 |
+
onset = np.zeros(self.complete_wo_Roll2Midi_midi.shape)
|
53 |
+
offset = np.zeros(self.complete_wo_Roll2Midi_midi.shape)
|
54 |
+
for j in range(self.complete_wo_Roll2Midi_midi.shape[0]):
|
55 |
+
if j != 0:
|
56 |
+
onset[j][np.setdiff1d(self.complete_wo_Roll2Midi_midi[j].nonzero(),
|
57 |
+
self.complete_wo_Roll2Midi_midi[j - 1].nonzero())] = 1
|
58 |
+
offset[j][np.setdiff1d(self.complete_wo_Roll2Midi_midi[j - 1].nonzero(),
|
59 |
+
self.complete_wo_Roll2Midi_midi[j].nonzero())] = -1
|
60 |
+
else:
|
61 |
+
onset[j][self.complete_wo_Roll2Midi_midi[j].nonzero()] = 1
|
62 |
+
onset += offset
|
63 |
+
self.complete_wo_Roll2Midi_onset = onset.T
|
64 |
+
print("Without Roll2MidiNet, the onset has shape:", self.complete_wo_Roll2Midi_onset.shape)
|
65 |
+
|
66 |
+
def process_midi(self):
|
67 |
+
self.w_Roll2Midi_data = []
|
68 |
+
self.infer_out_files = glob.glob(self.midi_out_folder + '/*.npz')
|
69 |
+
self.infer_out_files.sort(key=lambda x: int(x.split('/')[-1].split('.')[0].split('-')[0]))
|
70 |
+
|
71 |
+
# Use the Midi prediction for Synthesis
|
72 |
+
for i in range(len(self.infer_out_files)):
|
73 |
+
with np.load(self.infer_out_files[i]) as data:
|
74 |
+
est_midi = data['midi']
|
75 |
+
target = np.zeros((self.frame, self.piano_keys))
|
76 |
+
target[:est_midi.shape[0], self.min_key:self.max_key+1] = est_midi
|
77 |
+
est_midi = target
|
78 |
+
est_midi = np.where(est_midi > 0, 1, 0)
|
79 |
+
self.w_Roll2Midi_data.append(est_midi)
|
80 |
+
self.complete_w_Roll2Midi_midi = np.concatenate(self.w_Roll2Midi_data)
|
81 |
+
print("With Roll2MidiNet Midi, the Midi result has shape:", self.complete_w_Roll2Midi_midi.shape)
|
82 |
+
# compute onsets and offsets
|
83 |
+
onset = np.zeros(self.complete_w_Roll2Midi_midi.shape)
|
84 |
+
offset = np.zeros(self.complete_w_Roll2Midi_midi.shape)
|
85 |
+
for j in range(self.complete_w_Roll2Midi_midi.shape[0]):
|
86 |
+
if j != 0:
|
87 |
+
onset[j][np.setdiff1d(self.complete_w_Roll2Midi_midi[j].nonzero(),
|
88 |
+
self.complete_w_Roll2Midi_midi[j - 1].nonzero())] = 1
|
89 |
+
offset[j][np.setdiff1d(self.complete_w_Roll2Midi_midi[j - 1].nonzero(),
|
90 |
+
self.complete_w_Roll2Midi_midi[j].nonzero())] = -1
|
91 |
+
else:
|
92 |
+
onset[j][self.complete_w_Roll2Midi_midi[j].nonzero()] = 1
|
93 |
+
onset += offset
|
94 |
+
self.complete_w_Roll2Midi_onset = onset.T
|
95 |
+
print("With Roll2MidiNet, the onset has shape:", self.complete_w_Roll2Midi_onset.shape)
|
96 |
+
|
97 |
+
def GetNote(self):
|
98 |
+
if self.midi:
|
99 |
+
self.w_Roll2Midi_notes = {}
|
100 |
+
for i in range(self.complete_w_Roll2Midi_onset.shape[0]):
|
101 |
+
tmp = self.complete_w_Roll2Midi_onset[i]
|
102 |
+
start = np.where(tmp == 1)[0]
|
103 |
+
end = np.where(tmp == -1)[0]
|
104 |
+
if len(start) != len(end):
|
105 |
+
end = np.append(end, tmp.shape)
|
106 |
+
merged_list = [(start[i], end[i]) for i in range(0, len(start))]
|
107 |
+
# 21 is the lowest piano key in the Midi note number (Midi has 128 notes)
|
108 |
+
self.w_Roll2Midi_notes[21 + i] = merged_list
|
109 |
+
else:
|
110 |
+
self.wo_Roll2Midi_notes = {}
|
111 |
+
for i in range(self.complete_wo_Roll2Midi_onset.shape[0]):
|
112 |
+
tmp = self.complete_wo_Roll2Midi_onset[i]
|
113 |
+
start = np.where(tmp==1)[0]
|
114 |
+
end = np.where(tmp==-1)[0]
|
115 |
+
if len(start)!=len(end):
|
116 |
+
end = np.append(end, tmp.shape)
|
117 |
+
merged_list = [(start[i], end[i]) for i in range(0, len(start))]
|
118 |
+
self.wo_Roll2Midi_notes[21 + i] = merged_list
|
119 |
+
|
120 |
+
|
121 |
+
|
122 |
+
def Synthesize(self):
|
123 |
+
if self.midi:
|
124 |
+
wav = self.generate_midi(self.w_Roll2Midi_notes, self.ins)
|
125 |
+
path = self.create_output_dir()
|
126 |
+
out_file = path + f'/Midi-{self.video_name}-{self.ins}.wav'
|
127 |
+
#librosa.output.write_wav(out_file, wav, sr=self.sample_rate)
|
128 |
+
sf.write(out_file, wav, self.sample_rate)
|
129 |
+
else:
|
130 |
+
wav = self.generate_midi(self.wo_Roll2Midi_notes, self.ins)
|
131 |
+
path = self.create_output_dir()
|
132 |
+
out_file = path + f'/Roll-{self.video_name}-{self.ins}.wav'
|
133 |
+
#librosa.output.write_wav(out_file, wav, sr=self.sample_rate)
|
134 |
+
sf.write(out_file, wav, self.sample_rate)
|
135 |
+
|
136 |
+
def generate_midi(self, notes, ins):
|
137 |
+
pm = pretty_midi.PrettyMIDI(initial_tempo=80)
|
138 |
+
piano_program = pretty_midi.instrument_name_to_program(ins) #Acoustic Grand Piano
|
139 |
+
piano = pretty_midi.Instrument(program=piano_program)
|
140 |
+
for key in list(notes.keys()):
|
141 |
+
values = notes[key]
|
142 |
+
for i in range(len(values)):
|
143 |
+
start, end = values[i]
|
144 |
+
note = pretty_midi.Note(velocity=100, pitch=key, start=start * self.spf, end=end * self.spf)
|
145 |
+
piano.notes.append(note)
|
146 |
+
pm.instruments.append(piano)
|
147 |
+
wav = pm.fluidsynth(fs=16000)
|
148 |
+
return wav
|
149 |
+
|
150 |
+
def create_output_dir(self):
|
151 |
+
synth_out_dir = os.path.join(self.syn_dir, self.video_name)
|
152 |
+
os.makedirs(synth_out_dir, exist_ok=True)
|
153 |
+
return synth_out_dir
|
154 |
+
|
155 |
+
if __name__ == "__main__":
|
156 |
+
# could select any instrument available in Midi
|
157 |
+
instrument = 'Acoustic Grand Piano'
|
158 |
+
for i in [1,3,4,5,6,7,8,9,10,11,12,13,14,15,16,17,19,21,22,23,24,25,26,27]:
|
159 |
+
video_name = f'{i}'
|
160 |
+
#print(video_name)
|
161 |
+
Midi_out_folder = '/ailab-train/speech/shansizhe/audeo/data/estimate_Roll/training/'# Generated Midi output folder, change to your own path
|
162 |
+
Synth = MIDISynth(Midi_out_folder, video_name, instrument)
|
163 |
+
Synth.GetNote()
|
164 |
+
Synth.Synthesize()
|
165 |
+
|
src/audeo/README.md
ADDED
@@ -0,0 +1,67 @@
|
|
|
|
|
|
|
|
|
|
|
|
|
|
|
|
|
|
|
|
|
|
|
|
|
|
|
|
|
|
|
|
|
|
|
|
|
|
|
|
|
|
|
|
|
|
|
|
|
|
|
|
|
|
|
|
|
|
|
|
|
|
|
|
|
|
|
|
|
|
|
|
|
|
|
|
|
|
|
|
|
|
|
|
|
|
|
|
|
|
|
|
|
|
|
|
|
|
|
|
|
|
|
|
|
|
|
|
|
|
|
|
|
|
|
|
|
|
|
|
|
|
|
|
|
|
|
|
|
|
|
|
|
|
|
|
|
1 |
+
# Audeo
|
2 |
+
|
3 |
+
## Introduction
|
4 |
+
This repository contains the code for the paper **"Audeo: Audio Generation for a Silent Performance Video"**, which is avilable [here](https://proceedings.neurips.cc/paper/2020/file/227f6afd3b7f89b96c4bb91f95d50f6d-Paper.pdf), published in NeurIPS 2020. More samples can be found in our [project webpage](http://faculty.washington.edu/shlizee/audeo/) and [Youtube Video](https://www.youtube.com/watch?v=8rS3VgjG7_c).
|
5 |
+
|
6 |
+
[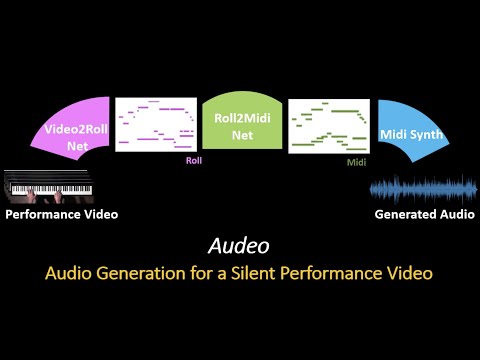](https://www.youtube.com/watch?v=8rS3VgjG7_c)
|
7 |
+
|
8 |
+
## Abstract
|
9 |
+
We present a novel system that gets as an input, video frames of a musician playing the piano, and generates the music for that video. The generation of music from
|
10 |
+
visual cues is a challenging problem and it is not clear whether it is an attainable goal at all. Our main aim in this work is to explore the plausibility of such a
|
11 |
+
transformation and to identify cues and components able to carry the association of sounds with visual events. To achieve the transformation we built a full pipeline
|
12 |
+
named ‘Audeo’ containing three components. We first translate the video frames of the keyboard and the musician hand movements into raw mechanical musical
|
13 |
+
symbolic representation Piano-Roll (Roll) for each video frame which represents the keys pressed at each time step. We then adapt the Roll to be amenable for audio
|
14 |
+
synthesis by including temporal correlations. This step turns out to be critical for meaningful audio generation. In the last step, we implement Midi synthesizers
|
15 |
+
to generate realistic music. Audeo converts video to audio smoothly and clearly with only a few setup constraints. We evaluate Audeo on piano performance videos
|
16 |
+
collected from Youtube and obtain that their generated music is of reasonable audio quality and can be successfully recognized with high precision by popular
|
17 |
+
music identification software.
|
18 |
+
|
19 |
+
## Data
|
20 |
+
We use Youtube Channel videos recorded by [Paul Barton](https://www.youtube.com/user/PaulBartonPiano) to evaluate the Audeo pipeline. For **Pseudo Midi Evaluation**, we use 24 videos of Bach Well-Tempered Clavier Book One (WTC B1). The testing set contains the first 3 Prelude and Fugue performances of Bach Well-Tempered Clavier Book Two (WTC B2) The Youtube Video Id can be found in [here](https://github.com/shlizee/Audeo/blob/master/Video_Id.md). For **Audio Evaluation**, we use 35 videos from WTC B2 (24 Prelude and Fugue pairs and their 11 variants), 8 videos from WTC B1 Variants, and 9 videos from other composers. Since we cannot host the videos due to copyright issues, you need to download the videos yourself.
|
21 |
+
|
22 |
+
All videos are set at the frame of 25 fps and the audio sampling rate of 16kHz. The **Pseudo GT Midi** are obtained via [Onsets and Frames framework (OF)](https://github.com/magenta/magenta/tree/master/magenta/models/onsets_frames_transcription). We process all videos and keep the full keyboard only and remove all frames that do not contribute to the piano performance (e.g., logos, black screens, etc). The **cropped piano coordinates** can be found in [here](https://github.com/shlizee/Audeo/blob/master/piano_coords.py) (The order is the same as in **Video_Id** file. We trim the initial silent sections up to the first frame in which the first key is being pressed, to align the video, Pseudo GT Midi, and the audio. All silent frames inside each performance are kept.
|
23 |
+
|
24 |
+
For your convenience, we provide the following folders/files in [Google Drive](https://drive.google.com/drive/folders/1w9wsZM-tPPUVqwdpsefEkrDgkN3kfg7G?usp=sharing):
|
25 |
+
- **input_images**: examples of how the images data should look like.
|
26 |
+
- **labels**: training and testing labels of for training/testing Video2Roll Net. Each folder contains a **pkl** file for one video. The labels are dictionaries where **key** is the **frame number** and **value** is a 88 dim vector. See **Video2Roll_dataset.py** for more details.
|
27 |
+
- **OF_midi_files**: the original Pseudo ground truth midi files obtained from **Onsets and Frames Framework**.
|
28 |
+
- **midi**: we process the Pseudo GT Midi files to 2D matrix (Piano keys x Time) and down-sampled to 25 fps. Then for each video, we divide them into multiple 2 seconds (50 frames) segments. For example **253-303.npz** includes the 2D matrix from frame 253 to frame 302.
|
29 |
+
- **estimate_Roll**: the **Roll** predictions obtained from **Video2Roll Net**. Same format as the **midi**. You can directly use them for training **Roll2Midi Net**.
|
30 |
+
- **Roll2Midi_results**: the **Midi** predictions obtained from **Roll2Midi Net**. Same format as the **midi** and **estimate_Roll**. Ready for **Midy Synth**.
|
31 |
+
- **Midi_Synth**: synthesized audios from **Roll2Midi_results**.
|
32 |
+
- **Video2Roll_models**: contains the pre-trained **Video2RollNet.pth**.
|
33 |
+
- **Roll2Midi_models**: contains the pre-trained **Roll2Midi Net**.
|
34 |
+
|
35 |
+
## How to Use
|
36 |
+
- Video2Roll Net
|
37 |
+
1. Please check the **Video2Roll_dataset.py** and make sure you satisfy the data formats.
|
38 |
+
2. Run **Video2Roll_train.py** for training.
|
39 |
+
3. Run **Video2Roll_evaluate.py** for evaluation.
|
40 |
+
4. Run **Video2Roll_inference.py** to generate **Roll** predictions.
|
41 |
+
- Roll2Midi Net
|
42 |
+
1. Run **Roll2Midi_train.py** for training.
|
43 |
+
2. Run **Roll2Midi_evaluate.py** for evaluation.
|
44 |
+
2. Run **Roll2Midi_inference.py** to generate **Midi** predictions.
|
45 |
+
- Midi Synth
|
46 |
+
1. Run **Midi_synth.py** to use **Fluid Synth** to synthesize audio.
|
47 |
+
|
48 |
+
## Requirements
|
49 |
+
- Pytorch >= 1.6
|
50 |
+
- Python 3
|
51 |
+
- numpy 1.19
|
52 |
+
- scikit-learn 0.22.1
|
53 |
+
- librosa 0.7.1
|
54 |
+
- pretty-midi 0.2.8
|
55 |
+
|
56 |
+
## Citation
|
57 |
+
|
58 |
+
Please cite ["Audeo: Audio Generation for a Silent Performance Video"](https://proceedings.neurips.cc/paper/2020/file/227f6afd3b7f89b96c4bb91f95d50f6d-Paper.pdf) when you use this code:
|
59 |
+
```
|
60 |
+
@article{su2020audeo,
|
61 |
+
title={Audeo: Audio generation for a silent performance video},
|
62 |
+
author={Su, Kun and Liu, Xiulong and Shlizerman, Eli},
|
63 |
+
journal={Advances in Neural Information Processing Systems},
|
64 |
+
volume={33},
|
65 |
+
year={2020}
|
66 |
+
}
|
67 |
+
```
|
src/audeo/Roll2MidiNet.py
ADDED
@@ -0,0 +1,139 @@
|
|
|
|
|
|
|
|
|
|
|
|
|
|
|
|
|
|
|
|
|
|
|
|
|
|
|
|
|
|
|
|
|
|
|
|
|
|
|
|
|
|
|
|
|
|
|
|
|
|
|
|
|
|
|
|
|
|
|
|
|
|
|
|
|
|
|
|
|
|
|
|
|
|
|
|
|
|
|
|
|
|
|
|
|
|
|
|
|
|
|
|
|
|
|
|
|
|
|
|
|
|
|
|
|
|
|
|
|
|
|
|
|
|
|
|
|
|
|
|
|
|
|
|
|
|
|
|
|
|
|
|
|
|
|
|
|
|
|
|
|
|
|
|
|
|
|
|
|
|
|
|
|
|
|
|
|
|
|
|
|
|
|
|
|
|
|
|
|
|
|
|
|
|
|
|
|
|
|
|
|
|
|
|
|
|
|
|
|
|
|
|
|
|
|
|
|
|
|
|
|
|
|
|
|
|
|
|
|
|
|
|
|
|
|
|
|
|
|
|
|
|
|
|
|
|
|
|
|
|
|
|
|
|
|
|
|
|
|
|
|
|
|
|
|
|
|
|
|
|
|
|
|
|
|
|
|
|
|
|
|
|
|
|
|
|
|
|
|
|
|
|
|
|
|
|
|
|
|
|
|
1 |
+
import torch.nn as nn
|
2 |
+
import torch.nn.functional as F
|
3 |
+
import torch
|
4 |
+
##############################
|
5 |
+
# U-NET
|
6 |
+
##############################
|
7 |
+
class UNetDown(nn.Module):
|
8 |
+
def __init__(self, in_size, out_size, normalize=True, dropout=0.0):
|
9 |
+
super(UNetDown, self).__init__()
|
10 |
+
model = [nn.Conv2d(in_size, out_size, 3, stride=1, padding=1, bias=False)]
|
11 |
+
if normalize:
|
12 |
+
model.append(nn.BatchNorm2d(out_size, 0.8))
|
13 |
+
model.append(nn.LeakyReLU(0.2))
|
14 |
+
if dropout:
|
15 |
+
model.append(nn.Dropout(dropout))
|
16 |
+
|
17 |
+
self.model = nn.Sequential(*model)
|
18 |
+
|
19 |
+
def forward(self, x):
|
20 |
+
return self.model(x)
|
21 |
+
|
22 |
+
|
23 |
+
class UNetUp(nn.Module):
|
24 |
+
def __init__(self, in_size, out_size, dropout=0.0):
|
25 |
+
super(UNetUp, self).__init__()
|
26 |
+
model = [
|
27 |
+
nn.ConvTranspose2d(in_size, out_size, 3, stride=1, padding=1, bias=False),
|
28 |
+
nn.BatchNorm2d(out_size, 0.8),
|
29 |
+
nn.ReLU(inplace=True),
|
30 |
+
]
|
31 |
+
if dropout:
|
32 |
+
model.append(nn.Dropout(dropout))
|
33 |
+
|
34 |
+
self.model = nn.Sequential(*model)
|
35 |
+
|
36 |
+
def forward(self, x, skip_input):
|
37 |
+
x = self.model(x)
|
38 |
+
out = torch.cat((x, skip_input), 1)
|
39 |
+
return out
|
40 |
+
|
41 |
+
|
42 |
+
class Generator(nn.Module):
|
43 |
+
def __init__(self, input_shape):
|
44 |
+
super(Generator, self).__init__()
|
45 |
+
channels, _ , _ = input_shape
|
46 |
+
self.down1 = UNetDown(channels, 64, normalize=False)
|
47 |
+
self.down2 = UNetDown(64, 128)
|
48 |
+
self.down3 = UNetDown(128, 256, dropout=0.5)
|
49 |
+
self.down4 = UNetDown(256, 512, dropout=0.5)
|
50 |
+
self.down5 = UNetDown(512, 1024, dropout=0.5)
|
51 |
+
self.down6 = UNetDown(1024, 1024, dropout=0.5)
|
52 |
+
|
53 |
+
self.up1 = UNetUp(1024, 512, dropout=0.5)
|
54 |
+
self.up2 = UNetUp(1024+512, 256, dropout=0.5)
|
55 |
+
self.up3 = UNetUp(512+256, 128, dropout=0.5)
|
56 |
+
self.up4 = UNetUp(256+128, 64)
|
57 |
+
self.up5 = UNetUp(128+64, 16)
|
58 |
+
self.conv1d = nn.Conv2d(80, 1, kernel_size=1)
|
59 |
+
|
60 |
+
def forward(self, x):
|
61 |
+
# U-Net generator with skip connections from encoder to decoder
|
62 |
+
d1 = self.down1(x)
|
63 |
+
|
64 |
+
d2 = self.down2(d1)
|
65 |
+
|
66 |
+
d3 = self.down3(d2)
|
67 |
+
|
68 |
+
d4 = self.down4(d3)
|
69 |
+
|
70 |
+
d5 = self.down5(d4)
|
71 |
+
|
72 |
+
d6 = self.down6(d5)
|
73 |
+
|
74 |
+
u1 = self.up1(d6, d5)
|
75 |
+
|
76 |
+
u2 = self.up2(u1, d4)
|
77 |
+
|
78 |
+
u3 = self.up3(u2, d3)
|
79 |
+
|
80 |
+
u4 = self.up4(u3, d2)
|
81 |
+
|
82 |
+
u5 = self.up5(u4, d1)
|
83 |
+
|
84 |
+
out = self.conv1d(u5)
|
85 |
+
|
86 |
+
out = F.sigmoid(out)
|
87 |
+
return out
|
88 |
+
|
89 |
+
|
90 |
+
class Discriminator(nn.Module):
|
91 |
+
def __init__(self, input_shape):
|
92 |
+
super(Discriminator, self).__init__()
|
93 |
+
|
94 |
+
channels, height, width = input_shape #1 51 50
|
95 |
+
|
96 |
+
# Calculate output of image discriminator (PatchGAN)
|
97 |
+
patch_h, patch_w = int(height / 2 ** 3)+1, int(width / 2 ** 3)+1
|
98 |
+
self.output_shape = (1, patch_h, patch_w)
|
99 |
+
|
100 |
+
def discriminator_block(in_filters, out_filters, stride, normalize):
|
101 |
+
"""Returns layers of each discriminator block"""
|
102 |
+
layers = [nn.Conv2d(in_filters, out_filters, 3, stride, 1)]
|
103 |
+
if normalize:
|
104 |
+
layers.append(nn.InstanceNorm2d(out_filters))
|
105 |
+
layers.append(nn.LeakyReLU(0.2, inplace=True))
|
106 |
+
return layers
|
107 |
+
|
108 |
+
layers = []
|
109 |
+
in_filters = channels
|
110 |
+
for out_filters, stride, normalize in [(64, 2, False), (128, 2, True), (256, 2, True), (512, 1, True)]:
|
111 |
+
layers.extend(discriminator_block(in_filters, out_filters, stride, normalize))
|
112 |
+
in_filters = out_filters
|
113 |
+
|
114 |
+
layers.append(nn.Conv2d(out_filters, 1, 3, 1, 1))
|
115 |
+
|
116 |
+
self.model = nn.Sequential(*layers)
|
117 |
+
|
118 |
+
def forward(self, img):
|
119 |
+
return self.model(img)
|
120 |
+
|
121 |
+
def weights_init_normal(m):
|
122 |
+
classname = m.__class__.__name__
|
123 |
+
if classname.find("Conv") != -1:
|
124 |
+
torch.nn.init.normal_(m.weight.data, 0.0, 0.02)
|
125 |
+
elif classname.find("BatchNorm2d") != -1:
|
126 |
+
torch.nn.init.normal_(m.weight.data, 1.0, 0.02)
|
127 |
+
torch.nn.init.constant_(m.bias.data, 0.0)
|
128 |
+
|
129 |
+
if __name__ == "__main__":
|
130 |
+
input_shape = (1,51, 100)
|
131 |
+
gnet = Generator(input_shape)
|
132 |
+
dnet = Discriminator(input_shape)
|
133 |
+
print(dnet.output_shape)
|
134 |
+
imgs = torch.rand((64,1,51,100))
|
135 |
+
gen = gnet(imgs)
|
136 |
+
print(gen.shape)
|
137 |
+
dis = dnet(gen)
|
138 |
+
print(dis.shape)
|
139 |
+
|
src/audeo/Roll2MidiNet_enhance.py
ADDED
@@ -0,0 +1,164 @@
|
|
|
|
|
|
|
|
|
|
|
|
|
|
|
|
|
|
|
|
|
|
|
|
|
|
|
|
|
|
|
|
|
|
|
|
|
|
|
|
|
|
|
|
|
|
|
|
|
|
|
|
|
|
|
|
|
|
|
|
|
|
|
|
|
|
|
|
|
|
|
|
|
|
|
|
|
|
|
|
|
|
|
|
|
|
|
|
|
|
|
|
|
|
|
|
|
|
|
|
|
|
|
|
|
|
|
|
|
|
|
|
|
|
|
|
|
|
|
|
|
|
|
|
|
|
|
|
|
|
|
|
|
|
|
|
|
|
|
|
|
|
|
|
|
|
|
|
|
|
|
|
|
|
|
|
|
|
|
|
|
|
|
|
|
|
|
|
|
|
|
|
|
|
|
|
|
|
|
|
|
|
|
|
|
|
|
|
|
|
|
|
|
|
|
|
|
|
|
|
|
|
|
|
|
|
|
|
|
|
|
|
|
|
|
|
|
|
|
|
|
|
|
|
|
|
|
|
|
|
|
|
|
|
|
|
|
|
|
|
|
|
|
|
|
|
|
|
|
|
|
|
|
|
|
|
|
|
|
|
|
|
|
|
|
|
|
|
|
|
|
|
|
|
|
|
|
|
|
|
|
|
|
|
|
|
|
|
|
|
|
|
|
|
|
|
|
|
|
|
|
|
|
|
|
|
|
|
|
|
|
|
|
|
|
|
|
|
|
|
|
|
|
|
|
|
|
|
|
|
|
1 |
+
import torch.nn as nn
|
2 |
+
import torch.nn.functional as F
|
3 |
+
import torch
|
4 |
+
##############################
|
5 |
+
# U-NET
|
6 |
+
##############################
|
7 |
+
class UNetDown(nn.Module):
|
8 |
+
def __init__(self, in_size, out_size, normalize=True, dropout=0.0):
|
9 |
+
super(UNetDown, self).__init__()
|
10 |
+
model = [nn.Conv2d(in_size, out_size, 3, stride=1, padding=1, bias=False)]
|
11 |
+
if normalize:
|
12 |
+
model.append(nn.BatchNorm2d(out_size, 0.8))
|
13 |
+
model.append(nn.LeakyReLU(0.2))
|
14 |
+
if dropout:
|
15 |
+
model.append(nn.Dropout(dropout))
|
16 |
+
|
17 |
+
self.model = nn.Sequential(*model)
|
18 |
+
|
19 |
+
def forward(self, x):
|
20 |
+
return self.model(x)
|
21 |
+
|
22 |
+
|
23 |
+
class UNetUp(nn.Module):
|
24 |
+
def __init__(self, in_size, out_size, dropout=0.0):
|
25 |
+
super(UNetUp, self).__init__()
|
26 |
+
model = [
|
27 |
+
nn.ConvTranspose2d(in_size, out_size, 3, stride=1, padding=1, bias=False),
|
28 |
+
nn.BatchNorm2d(out_size, 0.8),
|
29 |
+
nn.ReLU(inplace=True),
|
30 |
+
]
|
31 |
+
if dropout:
|
32 |
+
model.append(nn.Dropout(dropout))
|
33 |
+
|
34 |
+
self.model = nn.Sequential(*model)
|
35 |
+
|
36 |
+
def forward(self, x, skip_input):
|
37 |
+
x = self.model(x)
|
38 |
+
out = torch.cat((x, skip_input), 1)
|
39 |
+
return out
|
40 |
+
|
41 |
+
class AttentionGate(nn.Module):
|
42 |
+
def __init__(self, in_channels, g_channels, out_channels):
|
43 |
+
super(AttentionGate, self).__init__()
|
44 |
+
self.theta_x = nn.Conv2d(in_channels, out_channels, kernel_size=1)
|
45 |
+
self.phi_g = nn.Conv2d(g_channels, out_channels, kernel_size=1)
|
46 |
+
self.psi = nn.Conv2d(out_channels, 1, kernel_size=1)
|
47 |
+
self.sigmoid = nn.Sigmoid()
|
48 |
+
|
49 |
+
def forward(self, x, g):
|
50 |
+
theta_x = self.theta_x(x)
|
51 |
+
phi_g = self.phi_g(g)
|
52 |
+
f = theta_x + phi_g
|
53 |
+
f = self.psi(f)
|
54 |
+
alpha = self.sigmoid(f)
|
55 |
+
return x * alpha
|
56 |
+
|
57 |
+
class Generator(nn.Module):
|
58 |
+
def __init__(self, input_shape):
|
59 |
+
super(Generator, self).__init__()
|
60 |
+
channels, _ , _ = input_shape
|
61 |
+
self.down1 = UNetDown(channels, 64, normalize=False)
|
62 |
+
self.down2 = UNetDown(64, 128)
|
63 |
+
self.down3 = UNetDown(128, 256, dropout=0.5)
|
64 |
+
self.down4 = UNetDown(256, 512, dropout=0.5)
|
65 |
+
self.down5 = UNetDown(512, 1024, dropout=0.5)
|
66 |
+
self.down6 = UNetDown(1024, 1024, dropout=0.5)
|
67 |
+
|
68 |
+
# Attention Gates
|
69 |
+
self.att1 = AttentionGate(2048, 1024, 512)
|
70 |
+
self.att2 = AttentionGate(1024, 512, 256)
|
71 |
+
self.att3 = AttentionGate(512, 256, 128)
|
72 |
+
self.att4 = AttentionGate(256, 128, 64)
|
73 |
+
|
74 |
+
self.up1 = UNetUp(1024, 1024, dropout=0.5)
|
75 |
+
self.up2 = UNetUp(2048, 512, dropout=0.5)
|
76 |
+
self.up3 = UNetUp(1024, 256, dropout=0.5)
|
77 |
+
self.up4 = UNetUp(512, 128)
|
78 |
+
self.up5 = UNetUp(256, 64)
|
79 |
+
self.conv1d = nn.Conv2d(128, 1, kernel_size=1)
|
80 |
+
|
81 |
+
def forward(self, x):
|
82 |
+
# U-Net generator with skip connections from encoder to decoder
|
83 |
+
d1 = self.down1(x)
|
84 |
+
|
85 |
+
d2 = self.down2(d1)
|
86 |
+
|
87 |
+
d3 = self.down3(d2)
|
88 |
+
|
89 |
+
d4 = self.down4(d3)
|
90 |
+
|
91 |
+
d5 = self.down5(d4)
|
92 |
+
|
93 |
+
d6 = self.down6(d5)
|
94 |
+
|
95 |
+
u1 = self.up1(d6, d5)
|
96 |
+
u1 = self.att1(u1, d5)
|
97 |
+
|
98 |
+
u2 = self.up2(u1, d4)
|
99 |
+
u2 = self.att2(u2, d4)
|
100 |
+
|
101 |
+
u3 = self.up3(u2, d3)
|
102 |
+
u3 = self.att3(u3, d3)
|
103 |
+
|
104 |
+
u4 = self.up4(u3, d2)
|
105 |
+
u4 = self.att4(u4, d2)
|
106 |
+
|
107 |
+
u5 = self.up5(u4, d1)
|
108 |
+
|
109 |
+
out = self.conv1d(u5)
|
110 |
+
|
111 |
+
out = F.sigmoid(out)
|
112 |
+
return out
|
113 |
+
|
114 |
+
|
115 |
+
class Discriminator(nn.Module):
|
116 |
+
def __init__(self, input_shape):
|
117 |
+
super(Discriminator, self).__init__()
|
118 |
+
|
119 |
+
channels, height, width = input_shape #1 51 50
|
120 |
+
|
121 |
+
# Calculate output of image discriminator (PatchGAN)
|
122 |
+
patch_h, patch_w = int(height / 2 ** 3)+1, int(width / 2 ** 3)+1
|
123 |
+
self.output_shape = (1, patch_h, patch_w)
|
124 |
+
|
125 |
+
def discriminator_block(in_filters, out_filters, stride, normalize):
|
126 |
+
"""Returns layers of each discriminator block"""
|
127 |
+
layers = [nn.Conv2d(in_filters, out_filters, 3, stride, 1)]
|
128 |
+
if normalize:
|
129 |
+
layers.append(nn.InstanceNorm2d(out_filters))
|
130 |
+
layers.append(nn.LeakyReLU(0.2, inplace=True))
|
131 |
+
return layers
|
132 |
+
|
133 |
+
layers = []
|
134 |
+
in_filters = channels
|
135 |
+
for out_filters, stride, normalize in [(64, 2, False), (128, 2, True), (256, 2, True), (512, 1, True)]:
|
136 |
+
layers.extend(discriminator_block(in_filters, out_filters, stride, normalize))
|
137 |
+
in_filters = out_filters
|
138 |
+
|
139 |
+
layers.append(nn.Conv2d(out_filters, 1, 3, 1, 1))
|
140 |
+
|
141 |
+
self.model = nn.Sequential(*layers)
|
142 |
+
|
143 |
+
def forward(self, img):
|
144 |
+
return self.model(img)
|
145 |
+
|
146 |
+
def weights_init_normal(m):
|
147 |
+
classname = m.__class__.__name__
|
148 |
+
if classname.find("Conv") != -1:
|
149 |
+
torch.nn.init.normal_(m.weight.data, 0.0, 0.02)
|
150 |
+
elif classname.find("BatchNorm2d") != -1:
|
151 |
+
torch.nn.init.normal_(m.weight.data, 1.0, 0.02)
|
152 |
+
torch.nn.init.constant_(m.bias.data, 0.0)
|
153 |
+
|
154 |
+
if __name__ == "__main__":
|
155 |
+
input_shape = (1,51, 100)
|
156 |
+
gnet = Generator(input_shape)
|
157 |
+
dnet = Discriminator(input_shape)
|
158 |
+
print(dnet.output_shape)
|
159 |
+
imgs = torch.rand((64,1,51,100))
|
160 |
+
gen = gnet(imgs)
|
161 |
+
print(gen.shape)
|
162 |
+
dis = dnet(gen)
|
163 |
+
print(dis.shape)
|
164 |
+
|
src/audeo/Roll2Midi_dataset.py
ADDED
@@ -0,0 +1,160 @@
|
|
|
|
|
|
|
|
|
|
|
|
|
|
|
|
|
|
|
|
|
|
|
|
|
|
|
|
|
|
|
|
|
|
|
|
|
|
|
|
|
|
|
|
|
|
|
|
|
|
|
|
|
|
|
|
|
|
|
|
|
|
|
|
|
|
|
|
|
|
|
|
|
|
|
|
|
|
|
|
|
|
|
|
|
|
|
|
|
|
|
|
|
|
|
|
|
|
|
|
|
|
|
|
|
|
|
|
|
|
|
|
|
|
|
|
|
|
|
|
|
|
|
|
|
|
|
|
|
|
|
|
|
|
|
|
|
|
|
|
|
|
|
|
|
|
|
|
|
|
|
|
|
|
|
|
|
|
|
|
|
|
|
|
|
|
|
|
|
|
|
|
|
|
|
|
|
|
|
|
|
|
|
|
|
|
|
|
|
|
|
|
|
|
|
|
|
|
|
|
|
|
|
|
|
|
|
|
|
|
|
|
|
|
|
|
|
|
|
|
|
|
|
|
|
|
|
|
|
|
|
|
|
|
|
|
|
|
|
|
|
|
|
|
|
|
|
|
|
|
|
|
|
|
|
|
|
|
|
|
|
|
|
|
|
|
|
|
|
|
|
|
|
|
|
|
|
|
|
|
|
|
|
|
|
|
|
|
|
|
|
|
|
|
|
|
|
|
|
|
|
|
|
|
|
|
|
|
|
|
|
|
|
|
|
|
|
|
|
|
|
|
|
1 |
+
import matplotlib.pyplot as plt
|
2 |
+
import numpy as np
|
3 |
+
import torch
|
4 |
+
from torch.utils.data import Dataset,DataLoader
|
5 |
+
import glob
|
6 |
+
print(torch.cuda.current_device())
|
7 |
+
DEFAULT_DEVICE = 'cuda'
|
8 |
+
|
9 |
+
torch.cuda.set_device(0)
|
10 |
+
|
11 |
+
frames = 50 #2 seconds
|
12 |
+
|
13 |
+
min_key = 15
|
14 |
+
max_key = 65
|
15 |
+
|
16 |
+
class Roll2MidiDataset(Dataset):
|
17 |
+
def __init__(self, path='/ailab-train/speech/shansizhe/audeo/data/midi_npz', est_roll_path='/ailab-train/speech/shansizhe/audeo/data/estimate_Roll_exp3',
|
18 |
+
train=True, device=DEFAULT_DEVICE):
|
19 |
+
self.path = path
|
20 |
+
self.est_roll_path = est_roll_path
|
21 |
+
self.device = device
|
22 |
+
self.train = train
|
23 |
+
self.load_data()
|
24 |
+
def __getitem__(self, index):
|
25 |
+
if self.train:
|
26 |
+
gt, roll = self.final_data['train'][index]
|
27 |
+
else:
|
28 |
+
gt, roll = self.final_data['test'][index]
|
29 |
+
gt_ = gt.T.float().to(self.device)
|
30 |
+
roll_ = roll.T.float().to(self.device)
|
31 |
+
return torch.unsqueeze(gt_, dim=0), torch.unsqueeze(torch.sigmoid(roll_), dim=0)
|
32 |
+
|
33 |
+
def __len__(self):
|
34 |
+
if self.train:
|
35 |
+
return len(self.final_data['train'])
|
36 |
+
else:
|
37 |
+
return len(self.final_data['test'])
|
38 |
+
|
39 |
+
def load_data(self):
|
40 |
+
self.files = []
|
41 |
+
self.labels = []
|
42 |
+
|
43 |
+
# ground truth midi dir
|
44 |
+
path = self.path
|
45 |
+
#print(path)
|
46 |
+
train_gt_folders = glob.glob(path + '/training/*')
|
47 |
+
train_gt_folders.sort(key=lambda x: int(x.split('/')[-1]))
|
48 |
+
print(train_gt_folders)
|
49 |
+
test_gt_folders = glob.glob(path + '/testing/*')
|
50 |
+
test_gt_folders.sort(key=lambda x: int(x.split('/')[-1]))
|
51 |
+
print(test_gt_folders)
|
52 |
+
|
53 |
+
# Roll predictions dir
|
54 |
+
train_roll_folder = glob.glob(self.est_roll_path + '/training/*')
|
55 |
+
train_roll_folder.sort(key=lambda x: int(x.split('/')[-1]))
|
56 |
+
print(train_roll_folder)
|
57 |
+
test_roll_folder = glob.glob(self.est_roll_path + '/testing/*')
|
58 |
+
test_roll_folder.sort(key=lambda x: int(x.split('/')[-1]))
|
59 |
+
print(test_roll_folder)
|
60 |
+
|
61 |
+
# self.folders: dictionary
|
62 |
+
# key: train/test, values: list of tuples [(ground truth midi folder name, roll prediction folder name)]
|
63 |
+
self.folders = {}
|
64 |
+
self.folders['train'] = [(train_gt_folders[i], train_roll_folder[i]) for i in range(len(train_gt_folders))]
|
65 |
+
print(self.folders['train'])
|
66 |
+
self.folders['test'] = [(test_gt_folders[i], test_roll_folder[i]) for i in range(len(test_gt_folders))]
|
67 |
+
print(self.folders['test'])
|
68 |
+
|
69 |
+
# self.data: dictionary
|
70 |
+
# key: train/test, value:list of tuples [(2 sec ground truth Midi, 2 sec Roll prediction logits)]
|
71 |
+
self.data = {}
|
72 |
+
self.data['train'] = []
|
73 |
+
self.data['test'] = []
|
74 |
+
|
75 |
+
# self.final_data: similar to the data, but concat two continuous 2 sec Roll prediction (4 seconds, 100 frames)
|
76 |
+
self.final_data = {}
|
77 |
+
self.final_data['train'] = []
|
78 |
+
self.final_data['test'] = []
|
79 |
+
|
80 |
+
# load training data
|
81 |
+
for train_gt_folder, est_roll_folder in self.folders['train']:
|
82 |
+
gt_files = glob.glob(train_gt_folder + '/*.npz')
|
83 |
+
gt_files.sort(key=lambda x: int(x.split('/')[-1].split('.')[0].split('-')[0].split('_')[1]))
|
84 |
+
est_roll_files = glob.glob(est_roll_folder + '/*.npz')
|
85 |
+
est_roll_files.sort(key=lambda x: int(x.split('/')[-1].split('.')[0].split('-')[0]))
|
86 |
+
print("have the same files of training gt and est roll:", len(gt_files) == len(est_roll_files))
|
87 |
+
for i in range(len(gt_files)):
|
88 |
+
with np.load(gt_files[i]) as data:
|
89 |
+
gt = data['midi'][:, min_key:max_key + 1]
|
90 |
+
if gt.shape[0] != frames:
|
91 |
+
target = np.zeros((frames, max_key-min_key+1))
|
92 |
+
target[:gt.shape[0], :] = gt
|
93 |
+
gt = target
|
94 |
+
gt = np.where(gt > 0, 1, 0)
|
95 |
+
with np.load(est_roll_files[i]) as data:
|
96 |
+
est_roll_logit = data['logit'][:, min_key:max_key + 1]
|
97 |
+
if est_roll_logit.shape[0] != frames:
|
98 |
+
target = np.zeros((frames, max_key-min_key+1))
|
99 |
+
target[:est_roll_logit.shape[0], :] = est_roll_logit
|
100 |
+
est_roll_logit = target
|
101 |
+
self.data['train'].append((torch.from_numpy(gt), torch.from_numpy(est_roll_logit)))
|
102 |
+
# make 4 sec data
|
103 |
+
for i in range(len(self.data['train'])):
|
104 |
+
if i + 1 < len(self.data['train']):
|
105 |
+
one_gt, one_roll = self.data['train'][i]
|
106 |
+
two_gt, two_roll = self.data['train'][i + 1]
|
107 |
+
final_gt = torch.cat([one_gt, two_gt], dim=0)
|
108 |
+
final_roll = torch.cat([one_roll, two_roll], dim=0)
|
109 |
+
self.final_data['train'].append((final_gt, final_roll))
|
110 |
+
|
111 |
+
print("total number of training data:", len(self.final_data['train']))
|
112 |
+
|
113 |
+
# load testing data
|
114 |
+
for test_gt_folder, est_roll_folder in self.folders['test']:
|
115 |
+
gt_files = glob.glob(test_gt_folder + '/*.npz')
|
116 |
+
gt_files.sort(key=lambda x: int(x.split('/')[-1].split('.')[0].split('-')[0].split('_')[1]))
|
117 |
+
est_roll_files = glob.glob(est_roll_folder + '/*.npz')
|
118 |
+
est_roll_files.sort(key=lambda x: int(x.split('/')[-1].split('.')[0].split('-')[0]))
|
119 |
+
print("have the same files of testing midi and roll:", len(gt_files) == len(est_roll_files))
|
120 |
+
for i in range(len(gt_files)):
|
121 |
+
with np.load(gt_files[i]) as data:
|
122 |
+
gt = data['midi'][:, min_key:max_key + 1]
|
123 |
+
if gt.shape[0] != frames:
|
124 |
+
target = np.zeros((frames, max_key-min_key+1))
|
125 |
+
target[:gt.shape[0], :] = gt
|
126 |
+
gt = target
|
127 |
+
gt = np.where(gt > 0, 1, 0)
|
128 |
+
with np.load(est_roll_files[i]) as data:
|
129 |
+
est_roll = data['logit'][:, min_key:max_key + 1] # data['midi']
|
130 |
+
if est_roll.shape[0] != frames:
|
131 |
+
target = np.zeros((frames, max_key-min_key+1))
|
132 |
+
target[:est_roll.shape[0], :] = est_roll
|
133 |
+
est_roll = target
|
134 |
+
self.data['test'].append((torch.from_numpy(gt), torch.from_numpy(est_roll)))
|
135 |
+
for i in range(0, len(self.data['test']), 2):
|
136 |
+
if i + 1 < len(self.data['test']):
|
137 |
+
one_gt, one_roll = self.data['test'][i]
|
138 |
+
two_gt, two_roll = self.data['test'][i + 1]
|
139 |
+
final_gt = torch.cat([one_gt, two_gt], dim=0)
|
140 |
+
final_roll = torch.cat([one_roll, two_roll], dim=0)
|
141 |
+
self.final_data['test'].append((final_gt, final_roll))
|
142 |
+
|
143 |
+
print("total number of testing data:", len(self.final_data['test']))
|
144 |
+
|
145 |
+
|
146 |
+
|
147 |
+
if __name__ == "__main__":
|
148 |
+
dataset = Roll2MidiDataset()
|
149 |
+
gt,midi = dataset.__getitem__(0)
|
150 |
+
print(gt.shape)
|
151 |
+
print(midi.shape)
|
152 |
+
fig, (ax1,ax2,ax3) = plt.subplots(1, 3)
|
153 |
+
ax1.imshow(gt.cpu().numpy().squeeze(), plt.cm.gray)
|
154 |
+
ax2.imshow(midi.cpu().numpy().squeeze(), plt.cm.gray)
|
155 |
+
plt.show()
|
156 |
+
data_loader = DataLoader(dataset, batch_size=64)
|
157 |
+
for i,data in enumerate(data_loader):
|
158 |
+
gts,midis = data
|
159 |
+
break
|
160 |
+
|
src/audeo/Roll2Midi_dataset_tv2a_eval.py
ADDED
@@ -0,0 +1,118 @@
|
|
|
|
|
|
|
|
|
|
|
|
|
|
|
|
|
|
|
|
|
|
|
|
|
|
|
|
|
|
|
|
|
|
|
|
|
|
|
|
|
|
|
|
|
|
|
|
|
|
|
|
|
|
|
|
|
|
|
|
|
|
|
|
|
|
|
|
|
|
|
|
|
|
|
|
|
|
|
|
|
|
|
|
|
|
|
|
|
|
|
|
|
|
|
|
|
|
|
|
|
|
|
|
|
|
|
|
|
|
|
|
|
|
|
|
|
|
|
|
|
|
|
|
|
|
|
|
|
|
|
|
|
|
|
|
|
|
|
|
|
|
|
|
|
|
|
|
|
|
|
|
|
|
|
|
|
|
|
|
|
|
|
|
|
|
|
|
|
|
|
|
|
|
|
|
|
|
|
|
|
|
|
|
|
|
|
|
|
|
|
|
|
|
|
|
|
|
|
|
|
|
|
|
|
|
|
|
|
|
|
|
|
|
|
|
|
|
|
|
|
|
|
|
|
|
|
|
|
|
|
|
|
|
|
|
|
|
|
1 |
+
import matplotlib.pyplot as plt
|
2 |
+
import numpy as np
|
3 |
+
import torch
|
4 |
+
from torch.utils.data import Dataset,DataLoader
|
5 |
+
import glob
|
6 |
+
print(torch.cuda.current_device())
|
7 |
+
DEFAULT_DEVICE = 'cuda'
|
8 |
+
|
9 |
+
torch.cuda.set_device(0)
|
10 |
+
|
11 |
+
frames = 50 #2 seconds
|
12 |
+
|
13 |
+
min_key = 15
|
14 |
+
max_key = 65
|
15 |
+
|
16 |
+
class Roll2MidiDataset(Dataset):
|
17 |
+
def __init__(self, path='/ailab-train/speech/shansizhe/audeo/data/tv2a_piano3_4000_pkl_npz/gt/npz/', est_roll_path='/ailab-train/speech/shansizhe/audeo/data/tv2a_piano3_4000_pkl_npz/v2a/npz/',
|
18 |
+
train=True, device=DEFAULT_DEVICE):
|
19 |
+
self.path = path
|
20 |
+
self.est_roll_path = est_roll_path
|
21 |
+
self.device = device
|
22 |
+
self.train = train
|
23 |
+
self.load_data()
|
24 |
+
def __getitem__(self, index):
|
25 |
+
if self.train:
|
26 |
+
gt, roll = self.final_data['train'][index]
|
27 |
+
else:
|
28 |
+
gt, roll = self.final_data['test'][index]
|
29 |
+
gt_ = gt.T.float().to(self.device)
|
30 |
+
roll_ = roll.T.float().to(self.device)
|
31 |
+
return torch.unsqueeze(gt_, dim=0), torch.unsqueeze(roll_, dim=0)
|
32 |
+
|
33 |
+
def __len__(self):
|
34 |
+
if self.train:
|
35 |
+
return len(self.final_data['train'])
|
36 |
+
else:
|
37 |
+
return len(self.final_data['test'])
|
38 |
+
|
39 |
+
def load_data(self):
|
40 |
+
self.files = []
|
41 |
+
self.labels = []
|
42 |
+
|
43 |
+
# ground truth midi dir
|
44 |
+
path = self.path
|
45 |
+
#print(path)
|
46 |
+
train_gt_folders = glob.glob(path + '/*')
|
47 |
+
train_gt_folders.sort(key=lambda x: x.split('/')[-1].split('__')[-1])
|
48 |
+
print(train_gt_folders)
|
49 |
+
|
50 |
+
|
51 |
+
# Roll predictions dir
|
52 |
+
train_roll_folder = glob.glob(self.est_roll_path + '/*')
|
53 |
+
train_roll_folder.sort(key=lambda x: x.split('/')[-1].split('__')[-1])
|
54 |
+
print(train_roll_folder)
|
55 |
+
|
56 |
+
# self.folders: dictionary
|
57 |
+
# key: train/test, values: list of tuples [(ground truth midi folder name, roll prediction folder name)]
|
58 |
+
self.folders = {}
|
59 |
+
self.folders['train'] = [(train_gt_folders[i], train_roll_folder[i]) for i in range(len(train_gt_folders))]
|
60 |
+
print(self.folders['train'])
|
61 |
+
|
62 |
+
# self.data: dictionary
|
63 |
+
# key: train/test, value:list of tuples [(2 sec ground truth Midi, 2 sec Roll prediction logits)]
|
64 |
+
self.data = {}
|
65 |
+
self.data['train'] = []
|
66 |
+
|
67 |
+
# self.final_data: similar to the data, but concat two continuous 2 sec Roll prediction (4 seconds, 100 frames)
|
68 |
+
self.final_data = {}
|
69 |
+
self.final_data['train'] = []
|
70 |
+
|
71 |
+
# load training data
|
72 |
+
for train_gt_folder, est_roll_folder in self.folders['train']:
|
73 |
+
gt_files = glob.glob(train_gt_folder + '/*.npz')
|
74 |
+
gt_files.sort(key=lambda x: int(x.split('/')[-1].split('.')[0].split('-')[0]))
|
75 |
+
est_roll_files = glob.glob(est_roll_folder + '/*.npz')
|
76 |
+
est_roll_files.sort(key=lambda x: int(x.split('/')[-1].split('.')[0].split('-')[0]))
|
77 |
+
print("have the same files of training gt and est roll:", len(gt_files) == len(est_roll_files))
|
78 |
+
for i in range(len(gt_files)):
|
79 |
+
with np.load(gt_files[i]) as data:
|
80 |
+
gt = data['midi'][:, min_key:max_key + 1]
|
81 |
+
if gt.shape[0] != frames:
|
82 |
+
target = np.zeros((frames, max_key-min_key+1))
|
83 |
+
target[:gt.shape[0], :] = gt
|
84 |
+
gt = target
|
85 |
+
gt = np.where(gt > 0, 1, 0)
|
86 |
+
with np.load(est_roll_files[i]) as data:
|
87 |
+
est_roll_logit = data['midi'][:, min_key:max_key + 1]
|
88 |
+
if est_roll_logit.shape[0] != frames:
|
89 |
+
target = np.zeros((frames, max_key-min_key+1))
|
90 |
+
target[:est_roll_logit.shape[0], :] = est_roll_logit
|
91 |
+
est_roll_logit = target
|
92 |
+
est_roll_logit = np.where(est_roll_logit > 0, 1, 0)
|
93 |
+
self.data['train'].append((torch.from_numpy(gt), torch.from_numpy(est_roll_logit)))
|
94 |
+
# make 4 sec data
|
95 |
+
for i in range(len(self.data['train'])):
|
96 |
+
if i + 1 < len(self.data['train']):
|
97 |
+
one_gt, one_roll = self.data['train'][i]
|
98 |
+
two_gt, two_roll = self.data['train'][i + 1]
|
99 |
+
final_gt = torch.cat([one_gt, two_gt], dim=0)
|
100 |
+
final_roll = torch.cat([one_roll, two_roll], dim=0)
|
101 |
+
self.final_data['train'].append((final_gt, final_roll))
|
102 |
+
|
103 |
+
print("total number of training data:", len(self.final_data['train']))
|
104 |
+
|
105 |
+
if __name__ == "__main__":
|
106 |
+
dataset = Roll2MidiDataset()
|
107 |
+
gt,midi = dataset.__getitem__(0)
|
108 |
+
print(gt.shape)
|
109 |
+
print(midi.shape)
|
110 |
+
fig, (ax1,ax2,ax3) = plt.subplots(1, 3)
|
111 |
+
ax1.imshow(gt.cpu().numpy().squeeze(), plt.cm.gray)
|
112 |
+
ax2.imshow(midi.cpu().numpy().squeeze(), plt.cm.gray)
|
113 |
+
plt.show()
|
114 |
+
data_loader = DataLoader(dataset, batch_size=64)
|
115 |
+
for i,data in enumerate(data_loader):
|
116 |
+
gts,midis = data
|
117 |
+
break
|
118 |
+
|
src/audeo/Roll2Midi_evaluate.py
ADDED
@@ -0,0 +1,126 @@
|
|
|
|
|
|
|
|
|
|
|
|
|
|
|
|
|
|
|
|
|
|
|
|
|
|
|
|
|
|
|
|
|
|
|
|
|
|
|
|
|
|
|
|
|
|
|
|
|
|
|
|
|
|
|
|
|
|
|
|
|
|
|
|
|
|
|
|
|
|
|
|
|
|
|
|
|
|
|
|
|
|
|
|
|
|
|
|
|
|
|
|
|
|
|
|
|
|
|
|
|
|
|
|
|
|
|
|
|
|
|
|
|
|
|
|
|
|
|
|
|
|
|
|
|
|
|
|
|
|
|
|
|
|
|
|
|
|
|
|
|
|
|
|
|
|
|
|
|
|
|
|
|
|
|
|
|
|
|
|
|
|
|
|
|
|
|
|
|
|
|
|
|
|
|
|
|
|
|
|
|
|
|
|
|
|
|
|
|
|
|
|
|
|
|
|
|
|
|
|
|
|
|
|
|
|
|
|
|
|
|
|
|
|
|
|
|
|
|
|
|
|
|
|
|
|
|
|
|
|
|
|
|
|
|
|
|
|
|
|
|
|
|
|
|
|
|
|
|
|
|
|
|
|
|
1 |
+
import os
|
2 |
+
import json
|
3 |
+
from Roll2Midi_dataset import Roll2MidiDataset
|
4 |
+
from sklearn import metrics
|
5 |
+
import torch.utils.data as utils
|
6 |
+
import torch
|
7 |
+
from Roll2MidiNet_enhance import Generator
|
8 |
+
from torch.autograd import Variable
|
9 |
+
import numpy as np
|
10 |
+
from sklearn.metrics import _classification
|
11 |
+
cuda = torch.device("cuda")
|
12 |
+
Tensor = torch.cuda.FloatTensor
|
13 |
+
def process_data():
|
14 |
+
test_dataset = Roll2MidiDataset(train=False)
|
15 |
+
test_loader = utils.DataLoader(test_dataset, batch_size=16)
|
16 |
+
return test_loader
|
17 |
+
|
18 |
+
def test(generator, test_loader):
|
19 |
+
all_label = []
|
20 |
+
all_pred_label = []
|
21 |
+
all_pred_label_ = []
|
22 |
+
with torch.no_grad():
|
23 |
+
generator.eval()
|
24 |
+
for idx, data in enumerate(test_loader):
|
25 |
+
gt, roll = data
|
26 |
+
# Adversarial ground truths
|
27 |
+
gt = gt.type(Tensor)
|
28 |
+
roll = roll.type(Tensor)
|
29 |
+
|
30 |
+
real = Variable(gt)
|
31 |
+
roll_ = Variable(roll)
|
32 |
+
gen_imgs = generator(roll_)
|
33 |
+
|
34 |
+
pred_label = gen_imgs >= 0.4
|
35 |
+
numpy_label = gt.cpu().detach().numpy().astype(int) # B,1, 51, 50
|
36 |
+
numpy_label = np.transpose(numpy_label.squeeze(), (0, 2, 1)) # B,50,51
|
37 |
+
numpy_label = np.reshape(numpy_label, (-1, 51))
|
38 |
+
numpy_pre_label = pred_label.cpu().detach().numpy().astype(int)
|
39 |
+
numpy_pre_label = np.transpose(numpy_pre_label.squeeze(), (0, 2, 1)) #B,50,51
|
40 |
+
numpy_pre_label = np.reshape(numpy_pre_label, (-1, 51))
|
41 |
+
all_label.append(numpy_label)
|
42 |
+
all_pred_label.append(numpy_pre_label)
|
43 |
+
|
44 |
+
pred_label_ = gen_imgs >= 0.5
|
45 |
+
numpy_pre_label_ = pred_label_.cpu().detach().numpy().astype(int)
|
46 |
+
numpy_pre_label_ = np.transpose(numpy_pre_label_.squeeze(), (0, 2, 1)) # B,50,51
|
47 |
+
numpy_pre_label_ = np.reshape(numpy_pre_label_, (-1, 51))
|
48 |
+
all_pred_label_.append(numpy_pre_label_)
|
49 |
+
|
50 |
+
all_label = np.vstack(all_label)
|
51 |
+
all_pred_label = np.vstack(all_pred_label)
|
52 |
+
labels = _classification._check_set_wise_labels(all_label, all_pred_label, labels=None, pos_label=1,
|
53 |
+
average='samples')
|
54 |
+
MCM = metrics.multilabel_confusion_matrix(all_label, all_pred_label, sample_weight=None, labels=labels,
|
55 |
+
samplewise=True)
|
56 |
+
tp_sum = MCM[:, 1, 1]
|
57 |
+
fp_sum = MCM[:, 0, 1]
|
58 |
+
fn_sum = MCM[:, 1, 0]
|
59 |
+
# tn_sum = MCM[:, 0, 0]
|
60 |
+
accuracy = _prf_divide(tp_sum, tp_sum + fp_sum + fn_sum, zero_division=1)
|
61 |
+
accuracy = np.average(accuracy)
|
62 |
+
all_precision = metrics.precision_score(all_label, all_pred_label, average='samples', zero_division=1)
|
63 |
+
all_recall = metrics.recall_score(all_label, all_pred_label, average='samples', zero_division=1)
|
64 |
+
all_f1_score = metrics.f1_score(all_label, all_pred_label, average='samples', zero_division=1)
|
65 |
+
print(
|
66 |
+
"Threshold 0.4, avg precision:{0:.3f} | avg recall:{1:.3f} | avg acc:{2:.3f} | f1 score:{3:.3f}".format(
|
67 |
+
all_precision, all_recall, accuracy, all_f1_score))
|
68 |
+
|
69 |
+
all_pred_label_ = np.vstack(all_pred_label_)
|
70 |
+
labels = _classification._check_set_wise_labels(all_label, all_pred_label_, labels=None, pos_label=1,
|
71 |
+
average='samples')
|
72 |
+
MCM = metrics.multilabel_confusion_matrix(all_label, all_pred_label_, sample_weight=None, labels=labels,
|
73 |
+
samplewise=True)
|
74 |
+
tp_sum = MCM[:, 1, 1]
|
75 |
+
fp_sum = MCM[:, 0, 1]
|
76 |
+
fn_sum = MCM[:, 1, 0]
|
77 |
+
# tn_sum = MCM[:, 0, 0]
|
78 |
+
accuracy = _prf_divide(tp_sum, tp_sum + fp_sum + fn_sum, zero_division=1)
|
79 |
+
accuracy = np.average(accuracy)
|
80 |
+
all_precision = metrics.precision_score(all_label, all_pred_label_, average='samples', zero_division=1)
|
81 |
+
all_recall = metrics.recall_score(all_label, all_pred_label_, average='samples', zero_division=1)
|
82 |
+
all_f1_score = metrics.f1_score(all_label, all_pred_label_, average='samples', zero_division=1)
|
83 |
+
print(
|
84 |
+
"Threshold 0.5, avg precision:{0:.3f} | avg recall:{1:.3f} | avg acc:{2:.3f} | f1 score:{3:.3f}".format(
|
85 |
+
all_precision, all_recall,accuracy, all_f1_score))
|
86 |
+
return
|
87 |
+
|
88 |
+
def _prf_divide(numerator, denominator, zero_division="warn"):
|
89 |
+
"""Performs division and handles divide-by-zero.
|
90 |
+
On zero-division, sets the corresponding result elements equal to
|
91 |
+
0 or 1 (according to ``zero_division``). Plus, if
|
92 |
+
``zero_division != "warn"`` raises a warning.
|
93 |
+
The metric, modifier and average arguments are used only for determining
|
94 |
+
an appropriate warning.
|
95 |
+
"""
|
96 |
+
mask = denominator == 0.0
|
97 |
+
denominator = denominator.copy()
|
98 |
+
denominator[mask] = 1 # avoid infs/nans
|
99 |
+
result = numerator / denominator
|
100 |
+
|
101 |
+
if not np.any(mask):
|
102 |
+
return result
|
103 |
+
|
104 |
+
# if ``zero_division=1``, set those with denominator == 0 equal to 1
|
105 |
+
result[mask] = 0.0 if zero_division in ["warn", 0] else 1.0
|
106 |
+
|
107 |
+
# the user will be removing warnings if zero_division is set to something
|
108 |
+
# different than its default value. If we are computing only f-score
|
109 |
+
# the warning will be raised only if precision and recall are ill-defined
|
110 |
+
if zero_division != "warn":
|
111 |
+
return result
|
112 |
+
|
113 |
+
if __name__ == "__main__":
|
114 |
+
est_midi_folder = '/ailab-train/speech/shansizhe/audeo/data/estimate_Roll_exp3/testing'
|
115 |
+
exp_dir = "/ailab-train/speech/shansizhe/audeo/Correct_Roll2Midi_experiments/Roll2MidiNet_4_ep14_enhance"
|
116 |
+
with open(os.path.join(exp_dir,'hyperparams.json'), 'r') as hpfile:
|
117 |
+
hp = json.load(hpfile)
|
118 |
+
print(hp['best_loss'])
|
119 |
+
print(hp['best_epoch'])
|
120 |
+
checkpoints = 'checkpoint-best.tar'
|
121 |
+
checkpoint = torch.load(os.path.join(exp_dir, checkpoints))
|
122 |
+
test_loader = process_data()
|
123 |
+
input_shape = (1, 51, 100)
|
124 |
+
model = Generator(input_shape).cuda()
|
125 |
+
model.load_state_dict(checkpoint['state_dict_G'])
|
126 |
+
test(model, test_loader)
|
src/audeo/Roll2Midi_evaluate_tv2a.py
ADDED
@@ -0,0 +1,93 @@
|
|
|
|
|
|
|
|
|
|
|
|
|
|
|
|
|
|
|
|
|
|
|
|
|
|
|
|
|
|
|
|
|
|
|
|
|
|
|
|
|
|
|
|
|
|
|
|
|
|
|
|
|
|
|
|
|
|
|
|
|
|
|
|
|
|
|
|
|
|
|
|
|
|
|
|
|
|
|
|
|
|
|
|
|
|
|
|
|
|
|
|
|
|
|
|
|
|
|
|
|
|
|
|
|
|
|
|
|
|
|
|
|
|
|
|
|
|
|
|
|
|
|
|
|
|
|
|
|
|
|
|
|
|
|
|
|
|
|
|
|
|
|
|
|
|
|
|
|
|
|
|
|
|
|
|
|
|
|
|
|
|
|
|
|
|
|
|
|
|
|
|
|
|
|
|
|
|
|
|
|
|
|
|
|
|
|
|
|
1 |
+
import os
|
2 |
+
import json
|
3 |
+
from Roll2Midi_dataset_tv2a_eval import Roll2MidiDataset
|
4 |
+
from sklearn import metrics
|
5 |
+
import torch.utils.data as utils
|
6 |
+
import torch
|
7 |
+
from Roll2MidiNet import Generator
|
8 |
+
from torch.autograd import Variable
|
9 |
+
import numpy as np
|
10 |
+
from sklearn.metrics import _classification
|
11 |
+
cuda = torch.device("cuda")
|
12 |
+
Tensor = torch.cuda.FloatTensor
|
13 |
+
def process_data():
|
14 |
+
test_dataset = Roll2MidiDataset(train=True)
|
15 |
+
test_loader = utils.DataLoader(test_dataset, batch_size=16)
|
16 |
+
return test_loader
|
17 |
+
|
18 |
+
def test(test_loader):
|
19 |
+
all_label = []
|
20 |
+
all_pred_label = []
|
21 |
+
all_pred_label_ = []
|
22 |
+
with torch.no_grad():
|
23 |
+
#generator.eval()
|
24 |
+
for idx, data in enumerate(test_loader):
|
25 |
+
gt, roll = data
|
26 |
+
# Adversarial ground truths
|
27 |
+
gt = gt.type(Tensor)
|
28 |
+
roll = roll.type(Tensor)
|
29 |
+
|
30 |
+
real = Variable(gt)
|
31 |
+
roll_ = Variable(roll)
|
32 |
+
#gen_imgs = generator(roll_)
|
33 |
+
|
34 |
+
#pred_label = gen_imgs >= 0.4
|
35 |
+
numpy_label = gt.cpu().detach().numpy().astype(int) # B,1, 51, 50
|
36 |
+
numpy_label = np.transpose(numpy_label.squeeze(), (0, 2, 1)) # B,50,51
|
37 |
+
numpy_label = np.reshape(numpy_label, (-1, 51))
|
38 |
+
numpy_pre_label = roll.cpu().detach().numpy().astype(int)
|
39 |
+
numpy_pre_label = np.transpose(numpy_pre_label.squeeze(), (0, 2, 1)) #B,50,51
|
40 |
+
numpy_pre_label = np.reshape(numpy_pre_label, (-1, 51))
|
41 |
+
all_label.append(numpy_label)
|
42 |
+
all_pred_label.append(numpy_pre_label)
|
43 |
+
|
44 |
+
all_label = np.vstack(all_label)
|
45 |
+
all_pred_label = np.vstack(all_pred_label)
|
46 |
+
labels = _classification._check_set_wise_labels(all_label, all_pred_label, labels=None, pos_label=1,
|
47 |
+
average='samples')
|
48 |
+
MCM = metrics.multilabel_confusion_matrix(all_label, all_pred_label, sample_weight=None, labels=labels,
|
49 |
+
samplewise=True)
|
50 |
+
tp_sum = MCM[:, 1, 1]
|
51 |
+
fp_sum = MCM[:, 0, 1]
|
52 |
+
fn_sum = MCM[:, 1, 0]
|
53 |
+
# tn_sum = MCM[:, 0, 0]
|
54 |
+
accuracy = _prf_divide(tp_sum, tp_sum + fp_sum + fn_sum, zero_division=1)
|
55 |
+
accuracy = np.average(accuracy)
|
56 |
+
all_precision = metrics.precision_score(all_label, all_pred_label, average='weighted', zero_division=1)
|
57 |
+
all_recall = metrics.recall_score(all_label, all_pred_label, average='weighted', zero_division=1)
|
58 |
+
all_f1_score = metrics.f1_score(all_label, all_pred_label, average='weighted', zero_division=1)
|
59 |
+
print(
|
60 |
+
"avg precision:{0:.3f} | avg recall:{1:.3f} | avg acc:{2:.3f} | f1 score:{3:.3f}".format(
|
61 |
+
all_precision, all_recall, accuracy, all_f1_score))
|
62 |
+
|
63 |
+
return
|
64 |
+
|
65 |
+
def _prf_divide(numerator, denominator, zero_division="warn"):
|
66 |
+
"""Performs division and handles divide-by-zero.
|
67 |
+
On zero-division, sets the corresponding result elements equal to
|
68 |
+
0 or 1 (according to ``zero_division``). Plus, if
|
69 |
+
``zero_division != "warn"`` raises a warning.
|
70 |
+
The metric, modifier and average arguments are used only for determining
|
71 |
+
an appropriate warning.
|
72 |
+
"""
|
73 |
+
mask = denominator == 0.0
|
74 |
+
denominator = denominator.copy()
|
75 |
+
denominator[mask] = 1 # avoid infs/nans
|
76 |
+
result = numerator / denominator
|
77 |
+
|
78 |
+
if not np.any(mask):
|
79 |
+
return result
|
80 |
+
|
81 |
+
# if ``zero_division=1``, set those with denominator == 0 equal to 1
|
82 |
+
result[mask] = 0.0 if zero_division in ["warn", 0] else 1.0
|
83 |
+
|
84 |
+
# the user will be removing warnings if zero_division is set to something
|
85 |
+
# different than its default value. If we are computing only f-score
|
86 |
+
# the warning will be raised only if precision and recall are ill-defined
|
87 |
+
if zero_division != "warn":
|
88 |
+
return result
|
89 |
+
|
90 |
+
if __name__ == "__main__":
|
91 |
+
#est_midi_folder = '/ailab-train/speech/shansizhe/audeo/data/estimate_Roll/testing'
|
92 |
+
test_loader = process_data()
|
93 |
+
test(test_loader)
|
src/audeo/Roll2Midi_inference.py
ADDED
@@ -0,0 +1,100 @@
|
|
|
|
|
|
|
|
|
|
|
|
|
|
|
|
|
|
|
|
|
|
|
|
|
|
|
|
|
|
|
|
|
|
|
|
|
|
|
|
|
|
|
|
|
|
|
|
|
|
|
|
|
|
|
|
|
|
|
|
|
|
|
|
|
|
|
|
|
|
|
|
|
|
|
|
|
|
|
|
|
|
|
|
|
|
|
|
|
|
|
|
|
|
|
|
|
|
|
|
|
|
|
|
|
|
|
|
|
|
|
|
|
|
|
|
|
|
|
|
|
|
|
|
|
|
|
|
|
|
|
|
|
|
|
|
|
|
|
|
|
|
|
|
|
|
|
|
|
|
|
|
|
|
|
|
|
|
|
|
|
|
|
|
|
|
|
|
|
|
|
|
|
|
|
|
|
|
|
|
|
|
|
|
|
|
|
|
|
|
|
|
|
|
|
|
|
|
|
|
|
|
|
1 |
+
import os
|
2 |
+
import json
|
3 |
+
import numpy as np
|
4 |
+
import torch
|
5 |
+
import glob
|
6 |
+
from Roll2MidiNet import Generator
|
7 |
+
from torch.autograd import Variable
|
8 |
+
torch.cuda.set_device(0)
|
9 |
+
cuda = torch.device("cuda")
|
10 |
+
print(torch.cuda.current_device())
|
11 |
+
Tensor = torch.cuda.FloatTensor
|
12 |
+
class Midi_Generation():
|
13 |
+
def __init__(self, checkpoint, exp_dir, est_roll_folder, video_name):
|
14 |
+
# model dir
|
15 |
+
self.exp_dir = exp_dir
|
16 |
+
# load model checkpoint
|
17 |
+
self.checkpoint = torch.load(os.path.join(exp_dir,checkpoint))
|
18 |
+
# the video name
|
19 |
+
self.video_name = video_name
|
20 |
+
# the Roll prediction folder
|
21 |
+
self.est_roll_folder = est_roll_folder + video_name
|
22 |
+
# Midi output dir
|
23 |
+
self.infer_out_dir = '/ailab-train/speech/shansizhe/audeo/data/Roll2Midi_results/training/'
|
24 |
+
|
25 |
+
self.min_key = 15
|
26 |
+
self.max_key = 65
|
27 |
+
self.frame = 50
|
28 |
+
self.process_est_roll(self.est_roll_folder)
|
29 |
+
|
30 |
+
def process_est_roll(self, est_roll_folder):
|
31 |
+
self.data = []
|
32 |
+
self.final_data = []
|
33 |
+
self.est_roll_files = glob.glob(est_roll_folder + '/*.npz')
|
34 |
+
self.est_roll_files.sort(key=lambda x: int(x.split('/')[-1].split('.')[0].split('-')[0]))
|
35 |
+
print("need to infer {0} files".format(len(est_roll_folder)))
|
36 |
+
for i in range(len(self.est_roll_files)):
|
37 |
+
with np.load(self.est_roll_files[i]) as data:
|
38 |
+
est_roll = data['logit'][:,self.min_key:self.max_key+1]
|
39 |
+
if est_roll.shape[0] != self.frame:
|
40 |
+
target = np.zeros((self.frame, self.max_key-self.min_key+1))
|
41 |
+
target[:est_roll.shape[0], :] = est_roll
|
42 |
+
est_roll = target
|
43 |
+
self.data.append(torch.from_numpy(est_roll))
|
44 |
+
for i in range(0,len(self.data), 2):
|
45 |
+
if i + 1 < len(self.data):
|
46 |
+
one_roll = self.data[i]
|
47 |
+
two_roll = self.data[i+1]
|
48 |
+
final_roll = torch.cat([one_roll, two_roll], dim=0)
|
49 |
+
self.final_data.append(final_roll)
|
50 |
+
|
51 |
+
def inference(self):
|
52 |
+
input_shape = (1, self.max_key-self.min_key+1, 2*self.frame)
|
53 |
+
model = Generator(input_shape).cuda()
|
54 |
+
model.load_state_dict(self.checkpoint['state_dict_G'])
|
55 |
+
test_results = []
|
56 |
+
print('Inferencing MIDI......')
|
57 |
+
for i, data in enumerate(self.final_data):
|
58 |
+
roll = torch.unsqueeze(torch.unsqueeze(torch.sigmoid(data.T.float().cuda()), dim=0), dim=0)
|
59 |
+
print("piece ", i)
|
60 |
+
with torch.no_grad():
|
61 |
+
model.eval()
|
62 |
+
roll = roll.type(Tensor)
|
63 |
+
roll_ = Variable(roll)
|
64 |
+
gen_img = model(roll_)
|
65 |
+
gen_img = gen_img >= 0.5
|
66 |
+
|
67 |
+
numpy_pre_label = gen_img.cpu().detach().numpy().astype(int) # 1,1,88,100
|
68 |
+
numpy_pre_label = np.transpose(numpy_pre_label.squeeze(), (1, 0)) # 100,88
|
69 |
+
|
70 |
+
test_results.append(numpy_pre_label[:self.frame, :])
|
71 |
+
test_results.append(numpy_pre_label[self.frame:, :])
|
72 |
+
midi_out_dir = self.create_output_dir()
|
73 |
+
for i in range(len(test_results)):
|
74 |
+
print(self.est_roll_files[i])
|
75 |
+
idx = self.est_roll_files[i].split("/")[-1].split(".")[0].split("-")
|
76 |
+
idx1 = int(idx[0])
|
77 |
+
idx2 = int(idx[1])
|
78 |
+
print(idx1, idx2)
|
79 |
+
np.savez(midi_out_dir+f'/{idx1}-{idx2}.npz', midi=test_results[i])
|
80 |
+
|
81 |
+
def create_output_dir(self):
|
82 |
+
midi_out_dir = os.path.join(self.infer_out_dir, self.video_name)
|
83 |
+
os.makedirs(midi_out_dir, exist_ok=True)
|
84 |
+
return midi_out_dir
|
85 |
+
|
86 |
+
if __name__ == "__main__":
|
87 |
+
# example for generating the Midi output from training Roll predictions
|
88 |
+
est_roll_folder = '/ailab-train/speech/shansizhe/audeo/data/estimate_Roll/training/'
|
89 |
+
exp_dir = "/ailab-train/speech/shansizhe/audeo/Correct_Roll2Midi_experiments/Roll2MidiNet_1"
|
90 |
+
with open(os.path.join(exp_dir,'hyperparams.json'), 'r') as hpfile:
|
91 |
+
hp = json.load(hpfile)
|
92 |
+
print("the best loss:", hp['best_loss'])
|
93 |
+
print("the best epoch:", hp['best_epoch'])
|
94 |
+
|
95 |
+
checkpoints = 'checkpoint-{}.tar'.format(hp['best_epoch'])
|
96 |
+
for i in [1,3,4,5,6,7,8,9,10,11,12,13,14,15,16,17,19,21,22,23,24,25,26,27]:
|
97 |
+
video_name = f'{i}'
|
98 |
+
generator = Midi_Generation(checkpoints, exp_dir, est_roll_folder, video_name)
|
99 |
+
generator.inference()
|
100 |
+
|
src/audeo/Roll2Midi_train.py
ADDED
@@ -0,0 +1,280 @@
|
|
|
|
|
|
|
|
|
|
|
|
|
|
|
|
|
|
|
|
|
|
|
|
|
|
|
|
|
|
|
|
|
|
|
|
|
|
|
|
|
|
|
|
|
|
|
|
|
|
|
|
|
|
|
|
|
|
|
|
|
|
|
|
|
|
|
|
|
|
|
|
|
|
|
|
|
|
|
|
|
|
|
|
|
|
|
|
|
|
|
|
|
|
|
|
|
|
|
|
|
|
|
|
|
|
|
|
|
|
|
|
|
|
|
|
|
|
|
|
|
|
|
|
|
|
|
|
|
|
|
|
|
|
|
|
|
|
|
|
|
|
|
|
|
|
|
|
|
|
|
|
|
|
|
|
|
|
|
|
|
|
|
|
|
|
|
|
|
|
|
|
|
|
|
|
|
|
|
|
|
|
|
|
|
|
|
|
|
|
|
|
|
|
|
|
|
|
|
|
|
|
|
|
|
|
|
|
|
|
|
|
|
|
|
|
|
|
|
|
|
|
|
|
|
|
|
|
|
|
|
|
|
|
|
|
|
|
|
|
|
|
|
|
|
|
|
|
|
|
|
|
|
|
|
|
|
|
|
|
|
|
|
|
|
|
|
|
|
|
|
|
|
|
|
|
|
|
|
|
|
|
|
|
|
|
|
|
|
|
|
|
|
|
|
|
|
|
|
|
|
|
|
|
|
|
|
|
|
|
|
|
|
|
|
|
|
|
|
|
|
|
|
|
|
|
|
|
|
|
|
|
|
|
|
|
|
|
|
|
|
|
|
|
|
|
|
|
|
|
|
|
|
|
|
|
|
|
|
|
|
|
|
|
|
|
|
|
|
|
|
|
|
|
|
|
|
|
|
|
|
|
|
|
|
|
|
|
|
|
|
|
|
|
|
|
|
|
|
|
|
|
|
|
|
|
|
|
|
|
|
|
|
|
|
|
|
|
|
|
|
|
|
|
|
|
|
|
|
|
|
|
|
|
|
|
|
|
|
|
|
|
|
|
|
|
|
|
|
|
|
|
|
|
|
|
|
|
|
|
|
|
|
|
|
|
|
|
|
|
|
|
|
|
|
|
|
|
|
|
|
|
|
|
|
|
|
|
|
|
|
|
|
|
|
|
|
|
|
|
|
|
|
|
|
|
|
|
|
|
|
|
|
|
|
|
|
|
|
|
|
|
|
|
|
|
|
|
|
|
|
|
|
|
|
|
|
|
|
|
|
|
|
|
|
|
|
|
|
|
|
|
|
|
|
|
|
|
|
|
|
|
|
1 |
+
import os
|
2 |
+
import torch
|
3 |
+
import torch.optim as optim
|
4 |
+
import numpy as np
|
5 |
+
from torchvision.utils import save_image
|
6 |
+
import json
|
7 |
+
import torch.utils.data as utils
|
8 |
+
from Roll2MidiNet_enhance import Generator, Discriminator,weights_init_normal
|
9 |
+
from Roll2Midi_dataset import Roll2MidiDataset
|
10 |
+
from torch.autograd import Variable
|
11 |
+
from sklearn import metrics
|
12 |
+
from tqdm import tqdm
|
13 |
+
from torch.utils.tensorboard import SummaryWriter
|
14 |
+
|
15 |
+
torch.cuda.set_device(0)
|
16 |
+
cuda = torch.device("cuda")
|
17 |
+
print(torch.cuda.current_device())
|
18 |
+
Tensor = torch.cuda.FloatTensor
|
19 |
+
|
20 |
+
class hyperparams(object):
|
21 |
+
def __init__(self):
|
22 |
+
self.train_epoch = 200
|
23 |
+
self.test_freq = 1
|
24 |
+
self.exp_name = 'Roll2MidiNet_4_ep14_enhance'
|
25 |
+
|
26 |
+
self.channels = 1
|
27 |
+
self.h = 51 #input Piano key ranges
|
28 |
+
self.w = 100 # 4 seconds, 100 frames predictions
|
29 |
+
|
30 |
+
self.iter_train_g_loss = []
|
31 |
+
self.iter_train_d_loss = []
|
32 |
+
|
33 |
+
self.iter_test_g_loss = []
|
34 |
+
self.iter_test_d_loss = []
|
35 |
+
|
36 |
+
self.g_loss_history = []
|
37 |
+
self.d_loss_history = []
|
38 |
+
|
39 |
+
self.test_g_loss_history = []
|
40 |
+
self.test_d_loss_history = []
|
41 |
+
self.best_loss = 1e10
|
42 |
+
self.best_epoch = 0
|
43 |
+
|
44 |
+
def process_data():
|
45 |
+
train_dataset = Roll2MidiDataset(train=True)
|
46 |
+
train_loader = utils.DataLoader(train_dataset, batch_size=16, shuffle=True)
|
47 |
+
test_dataset = Roll2MidiDataset(train=False)
|
48 |
+
test_loader = utils.DataLoader(test_dataset, batch_size=16)
|
49 |
+
return train_loader, test_loader
|
50 |
+
|
51 |
+
def train(generator, discriminator, epoch, train_loader, optimizer_G, optimizer_D,
|
52 |
+
scheduler, adversarial_loss, iter_train_g_loss, iter_train_d_loss):
|
53 |
+
generator.train()
|
54 |
+
discriminator.train()
|
55 |
+
train_g_loss = 0
|
56 |
+
train_d_loss = 0
|
57 |
+
for batch_idx, data in tqdm(enumerate(train_loader)):
|
58 |
+
gt, roll = data
|
59 |
+
# Adversarial ground truths
|
60 |
+
valid = Variable(Tensor(gt.shape[0], *discriminator.output_shape).fill_(1.0), requires_grad=False)
|
61 |
+
fake = Variable(Tensor(gt.shape[0], *discriminator.output_shape).fill_(0.0), requires_grad=False)
|
62 |
+
gt = gt.type(Tensor)
|
63 |
+
roll = roll.type(Tensor)
|
64 |
+
|
65 |
+
real = Variable(gt)
|
66 |
+
roll_ = Variable(roll)
|
67 |
+
|
68 |
+
# -----------------
|
69 |
+
# Train Generator
|
70 |
+
# -----------------
|
71 |
+
|
72 |
+
optimizer_G.zero_grad()
|
73 |
+
|
74 |
+
# Generate a batch of images
|
75 |
+
gen_imgs = generator(roll_)
|
76 |
+
|
77 |
+
# Loss measures generator's ability to fool the discriminator
|
78 |
+
g_loss = 0.001*adversarial_loss(discriminator(gen_imgs), valid) + 0.999*adversarial_loss(gen_imgs, gt)
|
79 |
+
|
80 |
+
g_loss.backward()
|
81 |
+
|
82 |
+
iter_train_g_loss.append(g_loss.item())
|
83 |
+
train_g_loss += g_loss
|
84 |
+
|
85 |
+
optimizer_G.step()
|
86 |
+
|
87 |
+
# ---------------------
|
88 |
+
# Train Discriminator
|
89 |
+
# ---------------------
|
90 |
+
|
91 |
+
optimizer_D.zero_grad()
|
92 |
+
|
93 |
+
# Measure discriminator's ability to classify real from generated samples
|
94 |
+
real_loss = adversarial_loss(discriminator(real), valid)
|
95 |
+
fake_loss = adversarial_loss(discriminator(gen_imgs.detach()), fake)
|
96 |
+
d_loss = 0.5 * (real_loss + fake_loss)
|
97 |
+
|
98 |
+
d_loss.backward()
|
99 |
+
|
100 |
+
iter_train_d_loss.append(d_loss.item())
|
101 |
+
train_d_loss += d_loss
|
102 |
+
|
103 |
+
optimizer_D.step()
|
104 |
+
|
105 |
+
if batch_idx % 2 == 0:
|
106 |
+
print('Train Epoch: {0} [{1}/{2} ({3:.0f}%)]\t g Loss: {4:.6f} | d Loss: {5:.6f}'.format(epoch, batch_idx * roll.shape[0],
|
107 |
+
len(train_loader.dataset),
|
108 |
+
100. * batch_idx / len(train_loader),
|
109 |
+
g_loss.item() / roll.shape[0], d_loss.item() / roll.shape[0]))
|
110 |
+
scheduler.step(train_g_loss / len(train_loader.dataset))
|
111 |
+
print('====> Epoch: {} Average g loss: {:.4f} | d loss: {:.4f}'.format(epoch, train_g_loss / len(train_loader.dataset), train_d_loss / len(train_loader.dataset)))
|
112 |
+
return train_g_loss / len(train_loader.dataset),train_d_loss / len(train_loader.dataset)
|
113 |
+
|
114 |
+
def test(generator, discriminator, epoch, test_loader, adversarial_loss,
|
115 |
+
iter_test_g_loss,iter_test_d_loss):
|
116 |
+
all_label = []
|
117 |
+
all_pred_label = []
|
118 |
+
all_pred_label_ = []
|
119 |
+
with torch.no_grad():
|
120 |
+
generator.eval()
|
121 |
+
discriminator.eval()
|
122 |
+
test_g_loss = 0
|
123 |
+
test_d_loss = 0
|
124 |
+
for idx, data in enumerate(test_loader):
|
125 |
+
gt, roll = data
|
126 |
+
# Adversarial ground truths
|
127 |
+
valid = Variable(Tensor(gt.shape[0], *discriminator.output_shape).fill_(1.0), requires_grad=False)
|
128 |
+
fake = Variable(Tensor(gt.shape[0], *discriminator.output_shape).fill_(0.0), requires_grad=False)
|
129 |
+
gt = gt.type(Tensor)
|
130 |
+
roll = roll.type(Tensor)
|
131 |
+
|
132 |
+
real = Variable(gt)
|
133 |
+
roll_ = Variable(roll)
|
134 |
+
gen_imgs = generator(roll_)
|
135 |
+
|
136 |
+
# Loss measures generator's ability to fool the discriminator
|
137 |
+
g_loss = adversarial_loss(gen_imgs, gt)
|
138 |
+
|
139 |
+
iter_test_g_loss.append(g_loss.item())
|
140 |
+
test_g_loss += g_loss
|
141 |
+
|
142 |
+
# Measure discriminator's ability to classify real from generated samples
|
143 |
+
real_loss = adversarial_loss(discriminator(real), valid)
|
144 |
+
fake_loss = adversarial_loss(discriminator(gen_imgs.detach()), fake)
|
145 |
+
d_loss = 0.5 * (real_loss + fake_loss)
|
146 |
+
|
147 |
+
iter_test_d_loss.append(d_loss.item())
|
148 |
+
test_d_loss += d_loss
|
149 |
+
|
150 |
+
pred_label = gen_imgs >= 0.4
|
151 |
+
numpy_label = gt.cpu().detach().numpy().astype(int) # B,1,51, 50
|
152 |
+
numpy_label = np.transpose(numpy_label.squeeze(), (0, 2, 1)) # B,50,51
|
153 |
+
|
154 |
+
numpy_label = np.reshape(numpy_label, (-1, 51))
|
155 |
+
numpy_pre_label = pred_label.cpu().detach().numpy().astype(int)
|
156 |
+
numpy_pre_label = np.transpose(numpy_pre_label.squeeze(), (0, 2, 1)) #B,50,51
|
157 |
+
numpy_pre_label = np.reshape(numpy_pre_label, (-1, 51))
|
158 |
+
all_label.append(numpy_label)
|
159 |
+
all_pred_label.append(numpy_pre_label)
|
160 |
+
|
161 |
+
pred_label_ = gen_imgs >= 0.5
|
162 |
+
numpy_pre_label_ = pred_label_.cpu().detach().numpy().astype(int)
|
163 |
+
numpy_pre_label_ = np.transpose(numpy_pre_label_.squeeze(), (0, 2, 1)) # B,50,51
|
164 |
+
numpy_pre_label_ = np.reshape(numpy_pre_label_, (-1, 51))
|
165 |
+
all_pred_label_.append(numpy_pre_label_)
|
166 |
+
|
167 |
+
|
168 |
+
test_g_loss /= len(test_loader.dataset)
|
169 |
+
test_d_loss /= len(test_loader.dataset)
|
170 |
+
|
171 |
+
writer = SummaryWriter(log_dir='/ailab-train/speech/shansizhe/audeo/log/roll2midi/exp4_enhance')
|
172 |
+
|
173 |
+
# scheduler.step(test_loss)
|
174 |
+
print('====> Test set g loss: {:.4f} | d loss: {:.4f}'.format(test_g_loss, test_d_loss))
|
175 |
+
|
176 |
+
all_label = np.vstack(all_label)
|
177 |
+
all_pred_label = np.vstack(all_pred_label)
|
178 |
+
all_precision = metrics.precision_score(all_label, all_pred_label, average='samples', zero_division=1)
|
179 |
+
all_recall = metrics.recall_score(all_label, all_pred_label, average='samples', zero_division=1)
|
180 |
+
all_f1_score = metrics.f1_score(all_label, all_pred_label, average='samples', zero_division=1)
|
181 |
+
print(
|
182 |
+
"Threshold 0.4, epoch {0} avg precision:{1:.3f} | avg recall:{2:.3f} | f1 score:{3:.3f}".format(
|
183 |
+
epoch, all_precision, all_recall, all_f1_score))
|
184 |
+
|
185 |
+
writer.add_scalar('g_loss', test_g_loss, epoch)
|
186 |
+
writer.add_scalar('d_loss', test_d_loss, epoch)
|
187 |
+
writer.add_scalar('loss', test_d_loss + test_g_loss, epoch)
|
188 |
+
writer.add_scalar('Precision/t=0.4', all_precision, epoch)
|
189 |
+
writer.add_scalar('Recall/t=0.4', all_recall, epoch)
|
190 |
+
writer.add_scalar('F1_score/t=0.4', all_f1_score, epoch)
|
191 |
+
|
192 |
+
all_pred_label_ = np.vstack(all_pred_label_)
|
193 |
+
all_precision = metrics.precision_score(all_label, all_pred_label_, average='samples', zero_division=1)
|
194 |
+
all_recall = metrics.recall_score(all_label, all_pred_label_, average='samples', zero_division=1)
|
195 |
+
all_f1_score = metrics.f1_score(all_label, all_pred_label_, average='samples', zero_division=1)
|
196 |
+
print(
|
197 |
+
"Threshold 0.5, epoch {0} avg precision:{1:.3f} | avg recall:{2:.3f} | f1 score:{3:.3f}".format(
|
198 |
+
epoch, all_precision, all_recall, all_f1_score))
|
199 |
+
|
200 |
+
writer.add_scalar('Precision/t=0.5', all_precision, epoch)
|
201 |
+
writer.add_scalar('Recall/t=0.5', all_recall, epoch)
|
202 |
+
writer.add_scalar('F1_score/t=0.5', all_f1_score, epoch)
|
203 |
+
|
204 |
+
return test_g_loss, test_d_loss
|
205 |
+
|
206 |
+
|
207 |
+
def main():
|
208 |
+
hp = hyperparams()
|
209 |
+
|
210 |
+
try:
|
211 |
+
# the dir to save the Roll2Midi model
|
212 |
+
exp_root = "/ailab-train/speech/shansizhe/audeo/Correct_Roll2Midi_experiments"
|
213 |
+
os.makedirs(exp_root, exist_ok=True)
|
214 |
+
except FileExistsError:
|
215 |
+
pass
|
216 |
+
|
217 |
+
exp_dir = os.path.join(exp_root, hp.exp_name)
|
218 |
+
os.makedirs(exp_dir, exist_ok=True)
|
219 |
+
input_shape = (hp.channels, hp.h, hp.w)
|
220 |
+
# Loss function
|
221 |
+
adversarial_loss = torch.nn.MSELoss()
|
222 |
+
|
223 |
+
generator = Generator(input_shape)
|
224 |
+
discriminator = Discriminator(input_shape)
|
225 |
+
|
226 |
+
# Initialize weights
|
227 |
+
generator.apply(weights_init_normal)
|
228 |
+
discriminator.apply(weights_init_normal)
|
229 |
+
|
230 |
+
generator.cuda()
|
231 |
+
discriminator.cuda()
|
232 |
+
optimizer_G = torch.optim.Adam(generator.parameters(), lr=0.5*1e-3, betas=(0.9, 0.999))
|
233 |
+
optimizer_D = torch.optim.Adam(discriminator.parameters(), lr=0.5*1e-3, betas=(0.9, 0.999))
|
234 |
+
|
235 |
+
scheduler = optim.lr_scheduler.ReduceLROnPlateau(optimizer_G, 'min', patience=2)
|
236 |
+
train_loader, test_loader = process_data()
|
237 |
+
print ('start training')
|
238 |
+
for epoch in tqdm(range(hp.train_epoch)):
|
239 |
+
# training loop
|
240 |
+
g_loss, d_loss = train(generator, discriminator, epoch, train_loader, optimizer_G, optimizer_D,
|
241 |
+
scheduler, adversarial_loss, hp.iter_train_g_loss, hp.iter_train_d_loss)
|
242 |
+
hp.g_loss_history.append(g_loss.item())
|
243 |
+
hp.d_loss_history.append(d_loss.item())
|
244 |
+
|
245 |
+
# test
|
246 |
+
if epoch % hp.test_freq == 0:
|
247 |
+
test_g_loss,test_d_loss = test(generator, discriminator, epoch, test_loader, adversarial_loss,
|
248 |
+
hp.iter_test_g_loss, hp.iter_test_d_loss)
|
249 |
+
hp.test_g_loss_history.append(test_g_loss.item())
|
250 |
+
hp.test_d_loss_history.append(test_d_loss.item())
|
251 |
+
|
252 |
+
max_checkpoints = 5
|
253 |
+
# 在每个 epoch 后保存 checkpoint
|
254 |
+
torch.save({'epoch': epoch + 1,
|
255 |
+
'state_dict_G': generator.state_dict(),
|
256 |
+
'optimizer_G': optimizer_G.state_dict(),
|
257 |
+
'state_dict_D': discriminator.state_dict(),
|
258 |
+
'optimizer_D': optimizer_D.state_dict()},
|
259 |
+
os.path.join(exp_dir, 'checkpoint-{}.tar'.format(str(epoch + 1))))
|
260 |
+
|
261 |
+
# 如果达到最大 checkpoint 数量,删除最旧的 checkpoint
|
262 |
+
saved_checkpoints = sorted(os.listdir(exp_dir))
|
263 |
+
saved_checkpoints = [f for f in saved_checkpoints if f != 'checkpoint-best.tar']
|
264 |
+
if len(saved_checkpoints) > max_checkpoints:
|
265 |
+
oldest_checkpoint = saved_checkpoints[0]
|
266 |
+
os.remove(os.path.join(exp_dir, oldest_checkpoint))
|
267 |
+
|
268 |
+
if test_g_loss + test_d_loss < hp.best_loss:
|
269 |
+
torch.save({'epoch': epoch + 1, 'state_dict_G': generator.state_dict(),
|
270 |
+
'optimizer_G': optimizer_G.state_dict(),
|
271 |
+
'state_dict_D': discriminator.state_dict(),
|
272 |
+
'optimizer_D': optimizer_D.state_dict()},
|
273 |
+
os.path.join(exp_dir, 'checkpoint-best.tar'))
|
274 |
+
hp.best_loss = test_g_loss.item()+test_d_loss.item()
|
275 |
+
hp.best_epoch = epoch + 1
|
276 |
+
with open(os.path.join(exp_dir, 'hyperparams.json'), 'w') as outfile:
|
277 |
+
json.dump(hp.__dict__, outfile)
|
278 |
+
|
279 |
+
if __name__ == "__main__":
|
280 |
+
main()
|
src/audeo/Video2RollNet.py
ADDED
@@ -0,0 +1,264 @@
|
|
|
|
|
|
|
|
|
|
|
|
|
|
|
|
|
|
|
|
|
|
|
|
|
|
|
|
|
|
|
|
|
|
|
|
|
|
|
|
|
|
|
|
|
|
|
|
|
|
|
|
|
|
|
|
|
|
|
|
|
|
|
|
|
|
|
|
|
|
|
|
|
|
|
|
|
|
|
|
|
|
|
|
|
|
|
|
|
|
|
|
|
|
|
|
|
|
|
|
|
|
|
|
|
|
|
|
|
|
|
|
|
|
|
|
|
|
|
|
|
|
|
|
|
|
|
|
|
|
|
|
|
|
|
|
|
|
|
|
|
|
|
|
|
|
|
|
|
|
|
|
|
|
|
|
|
|
|
|
|
|
|
|
|
|
|
|
|
|
|
|
|
|
|
|
|
|
|
|
|
|
|
|
|
|
|
|
|
|
|
|
|
|
|
|
|
|
|
|
|
|
|
|
|
|
|
|
|
|
|
|
|
|
|
|
|
|
|
|
|
|
|
|
|
|
|
|
|
|
|
|
|
|
|
|
|
|
|
|
|
|
|
|
|
|
|
|
|
|
|
|
|
|
|
|
|
|
|
|
|
|
|
|
|
|
|
|
|
|
|
|
|
|
|
|
|
|
|
|
|
|
|
|
|
|
|
|
|
|
|
|
|
|
|
|
|
|
|
|
|
|
|
|
|
|
|
|
|
|
|
|
|
|
|
|
|
|
|
|
|
|
|
|
|
|
|
|
|
|
|
|
|
|
|
|
|
|
|
|
|
|
|
|
|
|
|
|
|
|
|
|
|
|
|
|
|
|
|
|
|
|
|
|
|
|
|
|
|
|
|
|
|
|
|
|
|
|
|
|
|
|
|
|
|
|
|
|
|
|
|
|
|
|
|
|
|
|
|
|
|
|
|
|
|
|
|
|
|
|
|
|
|
|
|
|
|
|
|
|
|
|
|
|
|
|
|
|
|
|
|
|
|
|
|
|
|
|
|
|
|
|
|
|
|
|
|
|
|
|
|
|
|
|
|
|
|
|
|
|
|
|
|
|
|
|
|
|
|
|
|
|
|
|
|
|
|
|
|
|
|
|
|
|
|
|
|
|
|
|
|
|
|
|
|
|
|
|
|
|
|
|
|
|
|
|
|
|
|
|
|
|
|
|
|
|
|
|
|
|
|
|
|
|
|
|
|
|
|
|
|
1 |
+
import torch.nn as nn
|
2 |
+
import math
|
3 |
+
import torch.nn.functional as F
|
4 |
+
import torch
|
5 |
+
|
6 |
+
__all__ = ['ResNet', 'resnet18']
|
7 |
+
|
8 |
+
|
9 |
+
def conv3x3(in_planes, out_planes, stride=1):
|
10 |
+
"""3x3 convolution with padding"""
|
11 |
+
return nn.Conv2d(in_planes, out_planes, kernel_size=3, stride=stride,
|
12 |
+
padding=1, bias=False)
|
13 |
+
|
14 |
+
class FTB(nn.Module):
|
15 |
+
def __init__(self,in_planes, out_planes=512, stride=1):
|
16 |
+
super(FTB,self).__init__()
|
17 |
+
self.conv0 = nn.Conv2d(in_planes, out_planes, kernel_size=1, stride=stride, padding=1,bias=False)
|
18 |
+
self.conv1 = conv3x3(out_planes, out_planes, stride)
|
19 |
+
self.bn1 = nn.BatchNorm2d(out_planes)
|
20 |
+
self.relu = nn.ReLU(inplace=True)
|
21 |
+
self.conv2 = conv3x3(out_planes, out_planes)
|
22 |
+
self.avgpool1 = nn.AvgPool2d(kernel_size=(2, 2), stride=2)
|
23 |
+
self.avgpool2 = nn.AvgPool2d(kernel_size=(3, 3), stride=1)
|
24 |
+
def forward(self, x, avg=True):
|
25 |
+
x1 = self.conv0(x)
|
26 |
+
residual = x1
|
27 |
+
out = self.conv1(x1)
|
28 |
+
out = self.bn1(out)
|
29 |
+
out = self.relu(out)
|
30 |
+
out = self.conv2(out)
|
31 |
+
out += residual
|
32 |
+
if avg:
|
33 |
+
out = self.avgpool1(out)
|
34 |
+
else:
|
35 |
+
out = self.avgpool2(out)
|
36 |
+
return out
|
37 |
+
|
38 |
+
class FRB(nn.Module):
|
39 |
+
def __init__(self,in_planes1,in_planes2):
|
40 |
+
super(FRB,self).__init__()
|
41 |
+
self.fc1 = nn.Linear(in_planes1+in_planes2, in_planes2)
|
42 |
+
self.relu = nn.ReLU(inplace=True)
|
43 |
+
self.fc2 = nn.Linear(in_planes2, in_planes2)
|
44 |
+
def forward(self, xl, xh):
|
45 |
+
xc = torch.cat([xl,xh],dim=1)
|
46 |
+
zc = F.avg_pool2d(xc, kernel_size=xc.size()[2:]) # C x 1 x 1
|
47 |
+
zc = torch.flatten(zc, 1)
|
48 |
+
out = self.fc1(zc)
|
49 |
+
out = self.relu(out)
|
50 |
+
out = self.fc2(out)
|
51 |
+
zc_ = F.sigmoid(out)
|
52 |
+
zc_ = torch.unsqueeze(zc_,dim=2)
|
53 |
+
zc_ = zc_.repeat(1, 1, xl.shape[2] * xl.shape[3]).view(-1,xl.shape[1],xl.shape[2],xl.shape[3])
|
54 |
+
xl_ = zc_ * xl #n,c,h,w
|
55 |
+
return xl_
|
56 |
+
|
57 |
+
class BasicBlock(nn.Module):
|
58 |
+
expansion = 1
|
59 |
+
|
60 |
+
def __init__(self, inplanes, planes, stride=1, downsample=None):
|
61 |
+
super(BasicBlock, self).__init__()
|
62 |
+
self.conv1 = conv3x3(inplanes, planes, stride)
|
63 |
+
self.bn1 = nn.BatchNorm2d(planes)
|
64 |
+
self.relu = nn.ReLU(inplace=True)
|
65 |
+
self.conv2 = conv3x3(planes, planes)
|
66 |
+
self.bn2 = nn.BatchNorm2d(planes)
|
67 |
+
self.downsample = downsample
|
68 |
+
self.stride = stride
|
69 |
+
|
70 |
+
def forward(self, x):
|
71 |
+
residual = x
|
72 |
+
|
73 |
+
out = self.conv1(x)
|
74 |
+
out = self.bn1(out)
|
75 |
+
out = self.relu(out)
|
76 |
+
|
77 |
+
out = self.conv2(out)
|
78 |
+
out = self.bn2(out)
|
79 |
+
|
80 |
+
if self.downsample is not None:
|
81 |
+
residual = self.downsample(x)
|
82 |
+
|
83 |
+
out += residual
|
84 |
+
out = self.relu(out)
|
85 |
+
|
86 |
+
return out
|
87 |
+
|
88 |
+
|
89 |
+
class Bottleneck(nn.Module):
|
90 |
+
expansion = 4
|
91 |
+
|
92 |
+
def __init__(self, inplanes, planes, stride=1, downsample=None):
|
93 |
+
super(Bottleneck, self).__init__()
|
94 |
+
self.conv1 = nn.Conv2d(inplanes, planes, kernel_size=1, bias=False)
|
95 |
+
self.bn1 = nn.BatchNorm2d(planes)
|
96 |
+
self.conv2 = nn.Conv2d(planes, planes, kernel_size=3, stride=stride,padding=1, bias=False)
|
97 |
+
self.bn2 = nn.BatchNorm2d(planes)
|
98 |
+
self.conv3 = nn.Conv2d(planes, planes * 4, kernel_size=1, bias=False)
|
99 |
+
self.bn3 = nn.BatchNorm2d(planes * 4)
|
100 |
+
self.relu = nn.ReLU(inplace=True)
|
101 |
+
self.downsample = downsample
|
102 |
+
self.stride = stride
|
103 |
+
|
104 |
+
def forward(self, x):
|
105 |
+
residual = x
|
106 |
+
|
107 |
+
out = self.conv1(x)
|
108 |
+
out = self.bn1(out)
|
109 |
+
out = self.relu(out)
|
110 |
+
|
111 |
+
out = self.conv2(out)
|
112 |
+
out = self.bn2(out)
|
113 |
+
out = self.relu(out)
|
114 |
+
|
115 |
+
out = self.conv3(out)
|
116 |
+
out = self.bn3(out)
|
117 |
+
|
118 |
+
if self.downsample is not None:
|
119 |
+
residual = self.downsample(x)
|
120 |
+
|
121 |
+
out += residual
|
122 |
+
out = self.relu(out)
|
123 |
+
|
124 |
+
return out
|
125 |
+
|
126 |
+
|
127 |
+
class ResNet(nn.Module):
|
128 |
+
|
129 |
+
def __init__(self, block, layers, top_channel_nums=2048, reduced_channel_nums=256, num_classes=51, scale=1):
|
130 |
+
self.inplanes = 64
|
131 |
+
super(ResNet, self).__init__()
|
132 |
+
self.conv1 = nn.Conv2d(5, 64, kernel_size=(11, 11), stride=(2, 2), padding=(4, 4),bias=False)
|
133 |
+
self.bn1 = nn.BatchNorm2d(64)
|
134 |
+
self.relu1 = nn.ReLU(inplace=True)
|
135 |
+
self.maxpool = nn.MaxPool2d(kernel_size=3, stride=2, padding=1)
|
136 |
+
self.layer1 = self._make_layer(block, 64, layers[0])
|
137 |
+
self.layer2 = self._make_layer(block, 128, layers[1], stride=2)
|
138 |
+
|
139 |
+
self.FTB2_1 = FTB(128, 128)
|
140 |
+
self.FTB2_2 = FTB(128, 128)
|
141 |
+
self.FRB2 = FRB(128, 128)
|
142 |
+
|
143 |
+
self.layer3 = self._make_layer(block, 256, layers[2], stride=2)
|
144 |
+
|
145 |
+
self.FTB3 = FTB(256, 128)
|
146 |
+
self.FRB3 = FRB(128, 128)
|
147 |
+
|
148 |
+
self.layer4 = self._make_layer(block, 512, layers[3], stride=2)
|
149 |
+
|
150 |
+
self.FTB4 = FTB(512, 128)
|
151 |
+
self.FRB4 = FRB(64, 128)
|
152 |
+
|
153 |
+
|
154 |
+
#FPN PARTS
|
155 |
+
# Top layer
|
156 |
+
self.toplayer = nn.Conv2d(top_channel_nums, reduced_channel_nums, kernel_size=1, stride=1, padding=0) # Reduce channels,
|
157 |
+
self.toplayer_bn = nn.BatchNorm2d(reduced_channel_nums)
|
158 |
+
self.toplayer_relu = nn.ReLU(inplace=True)
|
159 |
+
|
160 |
+
self.conv2 = nn.Conv2d(128, 128, kernel_size=1)
|
161 |
+
self.fc = nn.Linear(128, num_classes)
|
162 |
+
|
163 |
+
for m in self.modules():
|
164 |
+
if isinstance(m, nn.Conv2d):
|
165 |
+
n = m.kernel_size[0] * m.kernel_size[1] * m.out_channels
|
166 |
+
m.weight.data.normal_(0, math.sqrt(2. / n))
|
167 |
+
elif isinstance(m, nn.BatchNorm2d):
|
168 |
+
m.weight.data.fill_(1)
|
169 |
+
m.bias.data.zero_()
|
170 |
+
|
171 |
+
def _make_layer(self, block, planes, blocks, stride=1):
|
172 |
+
downsample = None
|
173 |
+
if stride != 1 or self.inplanes != planes * block.expansion:
|
174 |
+
downsample = nn.Sequential(
|
175 |
+
nn.Conv2d(self.inplanes, planes * block.expansion,
|
176 |
+
kernel_size=1, stride=stride, bias=False),
|
177 |
+
nn.BatchNorm2d(planes * block.expansion),
|
178 |
+
)
|
179 |
+
|
180 |
+
layers = []
|
181 |
+
layers.append(block(self.inplanes, planes, stride, downsample))
|
182 |
+
self.inplanes = planes * block.expansion
|
183 |
+
for i in range(1, blocks):
|
184 |
+
layers.append(block(self.inplanes, planes))
|
185 |
+
|
186 |
+
return nn.Sequential(*layers)
|
187 |
+
|
188 |
+
def _upsample(self, x, y, scale=1):
|
189 |
+
_, _, H, W = y.size()
|
190 |
+
return F.upsample(x, size=(H // scale, W // scale), mode='bilinear')
|
191 |
+
|
192 |
+
def _upsample_add(self, x, y):
|
193 |
+
_, _, H, W = y.size()
|
194 |
+
return F.upsample(x, size=(H, W), mode='bilinear') + y
|
195 |
+
|
196 |
+
def forward(self, x):
|
197 |
+
h = x
|
198 |
+
h = self.conv1(h)
|
199 |
+
h = self.bn1(h)
|
200 |
+
h = self.relu1(h)
|
201 |
+
h = self.maxpool(h)
|
202 |
+
|
203 |
+
h = self.layer1(h)
|
204 |
+
x1 = h
|
205 |
+
|
206 |
+
h = self.layer2(h)
|
207 |
+
x2 = h
|
208 |
+
|
209 |
+
h = self.layer3(h)
|
210 |
+
|
211 |
+
x3 = h
|
212 |
+
|
213 |
+
h = self.layer4(h)
|
214 |
+
x4 = h
|
215 |
+
|
216 |
+
# Top-down
|
217 |
+
x5 = self.toplayer(x4)
|
218 |
+
x5 = self.toplayer_relu(self.toplayer_bn(x5))
|
219 |
+
|
220 |
+
x2_ = self.FTB2_1(x2)
|
221 |
+
|
222 |
+
x2_ = self.FTB2_2(x2_)
|
223 |
+
|
224 |
+
x3_ = self.FTB3(x3)
|
225 |
+
|
226 |
+
x4_ = self.FTB4(x4, avg=False)
|
227 |
+
|
228 |
+
p4 = self.FRB4(x4_, x5)
|
229 |
+
|
230 |
+
p3 = self.FRB3(x3_, p4)
|
231 |
+
|
232 |
+
p2 = self.FRB2(x2_, p3)
|
233 |
+
|
234 |
+
out1 = p2*p3
|
235 |
+
|
236 |
+
out1_ = F.softmax(out1.view(*out1.size()[:2], -1),dim=2).view_as(out1)
|
237 |
+
|
238 |
+
out2 = out1_*p4
|
239 |
+
|
240 |
+
out2 = self.conv2(out2)
|
241 |
+
|
242 |
+
out = out2 + p4
|
243 |
+
|
244 |
+
out = F.avg_pool2d(out, kernel_size=out.size()[2:])
|
245 |
+
|
246 |
+
out = torch.flatten(out, 1)
|
247 |
+
|
248 |
+
out = self.fc(out)
|
249 |
+
|
250 |
+
return out
|
251 |
+
|
252 |
+
|
253 |
+
def resnet18(**kwargs):
|
254 |
+
"""Constructs a ResNet-18 model.
|
255 |
+
"""
|
256 |
+
model = ResNet(BasicBlock, layers=[2, 2, 2, 2], top_channel_nums=512, reduced_channel_nums=64, **kwargs)
|
257 |
+
return model
|
258 |
+
|
259 |
+
if __name__ == "__main__":
|
260 |
+
net = resnet18()
|
261 |
+
print(net)
|
262 |
+
imgs = torch.rand((2, 5, 100,900))
|
263 |
+
logits = net(imgs)
|
264 |
+
print(logits.shape)
|
src/audeo/Video2Roll_dataset.py
ADDED
@@ -0,0 +1,148 @@
|
|
|
|
|
|
|
|
|
|
|
|
|
|
|
|
|
|
|
|
|
|
|
|
|
|
|
|
|
|
|
|
|
|
|
|
|
|
|
|
|
|
|
|
|
|
|
|
|
|
|
|
|
|
|
|
|
|
|
|
|
|
|
|
|
|
|
|
|
|
|
|
|
|
|
|
|
|
|
|
|
|
|
|
|
|
|
|
|
|
|
|
|
|
|
|
|
|
|
|
|
|
|
|
|
|
|
|
|
|
|
|
|
|
|
|
|
|
|
|
|
|
|
|
|
|
|
|
|
|
|
|
|
|
|
|
|
|
|
|
|
|
|
|
|
|
|
|
|
|
|
|
|
|
|
|
|
|
|
|
|
|
|
|
|
|
|
|
|
|
|
|
|
|
|
|
|
|
|
|
|
|
|
|
|
|
|
|
|
|
|
|
|
|
|
|
|
|
|
|
|
|
|
|
|
|
|
|
|
|
|
|
|
|
|
|
|
|
|
|
|
|
|
|
|
|
|
|
|
|
|
|
|
|
|
|
|
|
|
|
|
|
|
|
|
|
|
|
|
|
|
|
|
|
|
|
|
|
|
|
|
|
|
|
|
|
|
|
|
|
|
|
|
|
|
|
|
|
|
|
|
|
|
|
|
|
|
|
|
|
|
|
|
|
|
|
|
|
|
1 |
+
import numpy as np
|
2 |
+
import glob
|
3 |
+
import matplotlib.pyplot as plt
|
4 |
+
from PIL import Image
|
5 |
+
from torch.utils.data import Dataset, DataLoader
|
6 |
+
import torchvision.transforms as transforms
|
7 |
+
import torch
|
8 |
+
from balance_data import MultilabelBalancedRandomSampler
|
9 |
+
# Resize all input images to 1 x 100 x 900
|
10 |
+
transform = transforms.Compose([lambda x: x.resize((900,100)),
|
11 |
+
lambda x: np.reshape(x,(100,900,1)),
|
12 |
+
lambda x: np.transpose(x,[2,0,1]),
|
13 |
+
lambda x: x/255.])
|
14 |
+
|
15 |
+
class Video2RollDataset(Dataset):
|
16 |
+
def __init__(self, img_root='./data/frame',label_root='./data/label', transform = transform, subset='train', device='cuda'):
|
17 |
+
self.img_root = img_root #images root dir
|
18 |
+
self.label_root = label_root #labels root dir
|
19 |
+
self.transform = transform
|
20 |
+
self.subset = subset
|
21 |
+
# the minimum and maximum Piano Key values in the data, depending on the data stats
|
22 |
+
self.min_key = 15 #3
|
23 |
+
self.max_key = 65 #79
|
24 |
+
self.device = device
|
25 |
+
self.load_data()
|
26 |
+
|
27 |
+
def __getitem__(self,index):
|
28 |
+
if self.subset=='train':
|
29 |
+
input_file_list, label = self.data['train'][index]
|
30 |
+
else:
|
31 |
+
input_file_list, label = self.data['test'][index]
|
32 |
+
input_img_list = []
|
33 |
+
# 5 consecutive frames, set binary
|
34 |
+
for input_file in input_file_list:
|
35 |
+
input_img = Image.open(input_file).convert('L')
|
36 |
+
binarr = np.array(input_img)
|
37 |
+
input_img = Image.fromarray(binarr.astype(np.uint8))
|
38 |
+
input_img_list.append(input_img)
|
39 |
+
|
40 |
+
new_input_img_list = []
|
41 |
+
for input_img in input_img_list:
|
42 |
+
new_input_img_list.append(self.transform(input_img))
|
43 |
+
# stack 5 consecutive frames
|
44 |
+
final_input_img = np.concatenate(new_input_img_list)
|
45 |
+
torch_input_img = torch.from_numpy(final_input_img).float().to(self.device)
|
46 |
+
torch_label = torch.from_numpy(label).float().to(self.device)
|
47 |
+
|
48 |
+
return torch_input_img, torch_label
|
49 |
+
def __len__(self):
|
50 |
+
if self.subset == 'train':
|
51 |
+
# return 20000
|
52 |
+
return len(self.data['train'])
|
53 |
+
else:
|
54 |
+
return len(self.data['test'])
|
55 |
+
|
56 |
+
def load_data(self):
|
57 |
+
# self.folders: dictionary
|
58 |
+
# key: train/test, values: list of tuples [(video_i_image_folder, video_i_label_folder)]
|
59 |
+
self.folders = {}
|
60 |
+
|
61 |
+
train_img_folder = glob.glob(self.img_root+'/training/*')
|
62 |
+
train_img_folder.sort(key=lambda x:int(x.split('/')[-1]))
|
63 |
+
test_img_folder = glob.glob(self.img_root+'/testing/*')
|
64 |
+
test_img_folder.sort(key=lambda x:int(x.split('/')[-1]))
|
65 |
+
train_label_folder = glob.glob(self.label_root+'/training/*')
|
66 |
+
train_label_folder.sort(key=lambda x: int(x.split('/')[-1].split('.')[0]))
|
67 |
+
test_label_folder = glob.glob(self.label_root+'/testing/*')
|
68 |
+
test_label_folder.sort(key=lambda x: int(x.split('/')[-1].split('.')[0]))
|
69 |
+
|
70 |
+
self.folders['train'] = [(train_img_folder[i],train_label_folder[i]) for i in range(len(train_img_folder))]
|
71 |
+
print(self.folders['train'])
|
72 |
+
self.folders['test'] = [(test_img_folder[i],test_label_folder[i]) for i in range(len(test_img_folder))]
|
73 |
+
print(self.folders['test'])
|
74 |
+
|
75 |
+
# self.data: dictionary
|
76 |
+
# key: train/test, value: list of tuples [([frame_{i-2, i+2}_image_filename], frame_i_label)]
|
77 |
+
self.data = {}
|
78 |
+
self.data['train'] = []
|
79 |
+
self.data['test'] = []
|
80 |
+
self.train_labels = []
|
81 |
+
count_zero = 0
|
82 |
+
# load train data
|
83 |
+
for img_folder, label_file in self.folders['train']:
|
84 |
+
# each folder contains all image frames of one video, format: frame{number}.jpg
|
85 |
+
img_files = glob.glob(img_folder + '/*.jpg')
|
86 |
+
img_files.sort(key=lambda x: int(x.split('/')[-1].split('.')[0][5:]))
|
87 |
+
# label is a pkl file. The key is frame number, value is the label vector of 88 dim
|
88 |
+
labels = np.load(label_file, allow_pickle=True)
|
89 |
+
for i, file in enumerate(img_files):
|
90 |
+
key = int(file.split('/')[-1].split('.')[0][5:])
|
91 |
+
label = labels[key]
|
92 |
+
# count the number of frames that no key is activate
|
93 |
+
if not np.any(label):
|
94 |
+
count_zero += 1
|
95 |
+
# continue
|
96 |
+
new_label = label[self.min_key:self.max_key + 1]
|
97 |
+
if i >= 2 and i<len(img_files)-2:
|
98 |
+
file_list = [img_files[i-2], img_files[i-1], file, img_files[i+1],img_files[i+2]]
|
99 |
+
else:
|
100 |
+
continue
|
101 |
+
self.data['train'].append((file_list, new_label))
|
102 |
+
self.train_labels.append(new_label)
|
103 |
+
print("number of all zero label in training:", count_zero)
|
104 |
+
self.train_labels = np.asarray(self.train_labels)
|
105 |
+
count_zero = 0
|
106 |
+
|
107 |
+
# load test data
|
108 |
+
for img_folder, label_file in self.folders['test']:
|
109 |
+
img_files = glob.glob(img_folder + '/*.jpg')
|
110 |
+
img_files.sort(key=lambda x: int(x.split('/')[-1].split('.')[0][5:]))
|
111 |
+
labels = np.load(label_file, allow_pickle=True)
|
112 |
+
for i, file in enumerate(img_files):
|
113 |
+
key = int(file.split('/')[-1].split('.')[0][5:])
|
114 |
+
label = labels[key]
|
115 |
+
if not np.any(label):
|
116 |
+
count_zero += 1
|
117 |
+
# continue
|
118 |
+
new_label = label[self.min_key:self.max_key + 1]
|
119 |
+
if i >= 2 and i<len(img_files)-2:
|
120 |
+
file_list = [img_files[i-2], img_files[i-1], file, img_files[i+1],img_files[i+2]]
|
121 |
+
else:
|
122 |
+
continue
|
123 |
+
self.data['test'].append((file_list, new_label))
|
124 |
+
print("number of all zero label in testing:", count_zero)
|
125 |
+
|
126 |
+
|
127 |
+
print("length of training data:",len(self.data['train']))
|
128 |
+
print("length of testing data:",len(self.data['test']))
|
129 |
+
|
130 |
+
if __name__ == "__main__":
|
131 |
+
dataset = Video2RollDataset(subset='train')
|
132 |
+
|
133 |
+
# g,h = dataset.__getitem__(200)
|
134 |
+
# print(g.shape)
|
135 |
+
# print(torch.nonzero(h))
|
136 |
+
train_sampler = MultilabelBalancedRandomSampler(dataset.train_labels)
|
137 |
+
train_loader = DataLoader(dataset, batch_size=64,sampler=train_sampler)
|
138 |
+
for i, data in enumerate(train_loader):
|
139 |
+
print(i)
|
140 |
+
imgs,label = data
|
141 |
+
print(label.shape)
|
142 |
+
# fig, (ax1) = plt.subplots(1)
|
143 |
+
# ax1.imshow(label.cpu().numpy().T, plt.cm.gray)
|
144 |
+
# plt.show()
|
145 |
+
# print(torch.nonzero(label, as_tuple=True))
|
146 |
+
print(torch.unique(torch.nonzero(label)[:,1]))
|
147 |
+
if i==3:
|
148 |
+
break
|
src/audeo/Video2Roll_evaluate.py
ADDED
@@ -0,0 +1,90 @@
|
|
|
|
|
|
|
|
|
|
|
|
|
|
|
|
|
|
|
|
|
|
|
|
|
|
|
|
|
|
|
|
|
|
|
|
|
|
|
|
|
|
|
|
|
|
|
|
|
|
|
|
|
|
|
|
|
|
|
|
|
|
|
|
|
|
|
|
|
|
|
|
|
|
|
|
|
|
|
|
|
|
|
|
|
|
|
|
|
|
|
|
|
|
|
|
|
|
|
|
|
|
|
|
|
|
|
|
|
|
|
|
|
|
|
|
|
|
|
|
|
|
|
|
|
|
|
|
|
|
|
|
|
|
|
|
|
|
|
|
|
|
|
|
|
|
|
|
|
|
|
|
|
|
|
|
|
|
|
|
|
|
|
|
|
|
|
|
|
|
|
|
|
|
|
|
|
|
|
|
|
|
|
1 |
+
import Video2RollNet
|
2 |
+
import os
|
3 |
+
import glob
|
4 |
+
import numpy as np
|
5 |
+
from PIL import Image
|
6 |
+
import torchvision.transforms as transforms
|
7 |
+
from Video2Roll_dataset import Video2RollDataset
|
8 |
+
from torch.utils.data import DataLoader
|
9 |
+
import torch
|
10 |
+
import time
|
11 |
+
from sklearn import metrics
|
12 |
+
from sklearn.metrics import _classification
|
13 |
+
import torch.nn as nn
|
14 |
+
def validate(net, criterion, test_loader):
|
15 |
+
epoch_loss = 0
|
16 |
+
count = 0
|
17 |
+
all_pred_label = []
|
18 |
+
all_label = []
|
19 |
+
with torch.no_grad():
|
20 |
+
for i, data in enumerate(test_loader):
|
21 |
+
imgs, label = data
|
22 |
+
logits = net(imgs)
|
23 |
+
loss = criterion(logits, label)
|
24 |
+
pred_label = torch.sigmoid(logits) >= 0.4
|
25 |
+
numpy_label = label.cpu().detach().numpy().astype(int)
|
26 |
+
numpy_pre_label = pred_label.cpu().detach().numpy().astype(int)
|
27 |
+
all_label.append(numpy_label)
|
28 |
+
all_pred_label.append(numpy_pre_label)
|
29 |
+
epoch_loss += loss.item()
|
30 |
+
count += 1
|
31 |
+
all_label = np.vstack(all_label)
|
32 |
+
all_pred_label = np.vstack(all_pred_label)
|
33 |
+
labels = _classification._check_set_wise_labels(all_label, all_pred_label,labels=None, pos_label=1, average='samples')
|
34 |
+
MCM = metrics.multilabel_confusion_matrix(all_label, all_pred_label,sample_weight=None, labels=labels, samplewise=True)
|
35 |
+
tp_sum = MCM[:, 1, 1]
|
36 |
+
fp_sum = MCM[:, 0, 1]
|
37 |
+
fn_sum = MCM[:, 1, 0]
|
38 |
+
# tn_sum = MCM[:, 0, 0]
|
39 |
+
accuracy = _prf_divide(tp_sum, tp_sum+fp_sum+fn_sum, zero_division=1)
|
40 |
+
accuracy = np.average(accuracy)
|
41 |
+
all_precision = metrics.precision_score(all_label, all_pred_label, average='samples', zero_division=1)
|
42 |
+
all_recall = metrics.recall_score(all_label, all_pred_label, average='samples', zero_division=1)
|
43 |
+
all_f1_score = metrics.f1_score(all_label, all_pred_label, average='samples', zero_division=1)
|
44 |
+
return epoch_loss/count, all_precision, all_recall, accuracy, all_f1_score
|
45 |
+
|
46 |
+
|
47 |
+
def _prf_divide(numerator, denominator, zero_division="warn"):
|
48 |
+
"""Performs division and handles divide-by-zero.
|
49 |
+
On zero-division, sets the corresponding result elements equal to
|
50 |
+
0 or 1 (according to ``zero_division``). Plus, if
|
51 |
+
``zero_division != "warn"`` raises a warning.
|
52 |
+
The metric, modifier and average arguments are used only for determining
|
53 |
+
an appropriate warning.
|
54 |
+
"""
|
55 |
+
mask = denominator == 0.0
|
56 |
+
denominator = denominator.copy()
|
57 |
+
denominator[mask] = 1 # avoid infs/nans
|
58 |
+
result = numerator / denominator
|
59 |
+
|
60 |
+
if not np.any(mask):
|
61 |
+
return result
|
62 |
+
|
63 |
+
# if ``zero_division=1``, set those with denominator == 0 equal to 1
|
64 |
+
result[mask] = 0.0 if zero_division in ["warn", 0] else 1.0
|
65 |
+
|
66 |
+
# the user will be removing warnings if zero_division is set to something
|
67 |
+
# different than its default value. If we are computing only f-score
|
68 |
+
# the warning will be raised only if precision and recall are ill-defined
|
69 |
+
if zero_division != "warn":
|
70 |
+
return result
|
71 |
+
|
72 |
+
if __name__ == "__main__":
|
73 |
+
model_path = './models/Video2Roll_50_0.4/14.pth'
|
74 |
+
device = torch.device('cuda')
|
75 |
+
net = Video2RollNet.resnet18()
|
76 |
+
# net = torch.nn.DataParallel(net)
|
77 |
+
net.cuda()
|
78 |
+
net.load_state_dict(torch.load(model_path))
|
79 |
+
print(net)
|
80 |
+
test_dataset = Video2RollDataset(subset='test')
|
81 |
+
test_data_loader = DataLoader(test_dataset, batch_size=64)
|
82 |
+
net.eval()
|
83 |
+
criterion=nn.BCEWithLogitsLoss()
|
84 |
+
val_avg_loss, val_avg_precision, val_avg_recall, val_avg_acc, val_fscore = validate(net, criterion, test_data_loader)
|
85 |
+
epoch = 0
|
86 |
+
print('-' * 85)
|
87 |
+
print(
|
88 |
+
"epoch {0} validation loss:{1:.3f} | avg precision:{2:.3f} | avg recall:{3:.3f} | avg acc:{4:.3f} | f1 score:{5:.3f}".format(
|
89 |
+
epoch + 1, val_avg_loss, val_avg_precision, val_avg_recall, val_avg_acc, val_fscore))
|
90 |
+
print('-' * 85)
|
src/audeo/Video2Roll_inference.py
ADDED
@@ -0,0 +1,151 @@
|
|
|
|
|
|
|
|
|
|
|
|
|
|
|
|
|
|
|
|
|
|
|
|
|
|
|
|
|
|
|
|
|
|
|
|
|
|
|
|
|
|
|
|
|
|
|
|
|
|
|
|
|
|
|
|
|
|
|
|
|
|
|
|
|
|
|
|
|
|
|
|
|
|
|
|
|
|
|
|
|
|
|
|
|
|
|
|
|
|
|
|
|
|
|
|
|
|
|
|
|
|
|
|
|
|
|
|
|
|
|
|
|
|
|
|
|
|
|
|
|
|
|
|
|
|
|
|
|
|
|
|
|
|
|
|
|
|
|
|
|
|
|
|
|
|
|
|
|
|
|
|
|
|
|
|
|
|
|
|
|
|
|
|
|
|
|
|
|
|
|
|
|
|
|
|
|
|
|
|
|
|
|
|
|
|
|
|
|
|
|
|
|
|
|
|
|
|
|
|
|
|
|
|
|
|
|
|
|
|
|
|
|
|
|
|
|
|
|
|
|
|
|
|
|
|
|
|
|
|
|
|
|
|
|
|
|
|
|
|
|
|
|
|
|
|
|
|
|
|
|
|
|
|
|
|
|
|
|
|
|
|
|
|
|
|
|
|
|
|
|
|
|
|
|
|
|
|
|
|
|
|
|
|
|
|
|
|
|
|
|
|
|
|
|
|
|
|
|
|
|
|
|
|
|
1 |
+
import Video2RollNet
|
2 |
+
import os
|
3 |
+
import glob
|
4 |
+
import numpy as np
|
5 |
+
from PIL import Image
|
6 |
+
import torchvision.transforms as transforms
|
7 |
+
import torch
|
8 |
+
transform = transforms.Compose([lambda x: x.resize((900,100)),
|
9 |
+
lambda x: np.reshape(x,(100,900,1)),
|
10 |
+
lambda x: np.transpose(x,[2,0,1]),
|
11 |
+
lambda x: x/255.])
|
12 |
+
|
13 |
+
# video images root dir, change to your path
|
14 |
+
img_root='./data/frame'
|
15 |
+
# labels root dir, change to your path
|
16 |
+
label_root='./data/label'
|
17 |
+
# midi ground truth root dir, change to your path
|
18 |
+
midi_root = './data/midi_npz'
|
19 |
+
# Roll prediction output, change to your path
|
20 |
+
#est_roll_root = '/ailab-train/speech/shansizhe/audeo/data/estimate_Roll_exp3/'
|
21 |
+
|
22 |
+
# the range of Piano keys (maximum is 88), depending on your data
|
23 |
+
min_key = 15
|
24 |
+
max_key = 65
|
25 |
+
|
26 |
+
def load_data(img_folder, label_file, midi_folder):
|
27 |
+
img_files = glob.glob(img_folder + '/*.jpg')
|
28 |
+
img_files.sort(key=lambda x: int(x.split('/')[-1].split('.')[0][5:]))
|
29 |
+
labels = np.load(label_file, allow_pickle=True)
|
30 |
+
# Midi info for every video is divided into multiple npz files
|
31 |
+
# each npz contains 2 seconds (50 frames) Midi information
|
32 |
+
# format: frame_{i}-frame_{i+50}.npz
|
33 |
+
midi_files = glob.glob(midi_folder + '/*.npz')
|
34 |
+
midi_files.sort(key=lambda x: int(x.split('/')[-1].split('.')[0].split('-')[0].split('_')[1]))
|
35 |
+
intervals = []
|
36 |
+
for file in midi_files:
|
37 |
+
interval = file.split('/')[-1].split('.')[0].split('-')
|
38 |
+
start = int(interval[0].split('_')[1])
|
39 |
+
end = int(interval[1].split('_')[1])
|
40 |
+
intervals.append([start, end])
|
41 |
+
data = []
|
42 |
+
for i, file in enumerate(img_files):
|
43 |
+
key = int(file.split('/')[-1].split('.')[0][5:])
|
44 |
+
label = np.where(labels[key] > 0, 1, 0)
|
45 |
+
new_label = label[min_key:max_key + 1]
|
46 |
+
if i >= 2 and i < len(img_files) - 2:
|
47 |
+
file_list = [img_files[i - 2], img_files[i - 1], file, img_files[i + 1], img_files[i + 2]]
|
48 |
+
elif i < 2:
|
49 |
+
file_list = [file, file, file, img_files[i + 1], img_files[i + 2]]
|
50 |
+
else:
|
51 |
+
file_list = [img_files[i - 2], img_files[i - 1], file, file, file]
|
52 |
+
data.append((file_list, new_label))
|
53 |
+
print("data", i, file, file_list, new_label)
|
54 |
+
return intervals, data
|
55 |
+
|
56 |
+
# infer 2 seconds every time
|
57 |
+
def inference(net, intervals, data, est_roll_folder):
|
58 |
+
net.eval()
|
59 |
+
i = 0
|
60 |
+
for interval in intervals:
|
61 |
+
start, end = interval
|
62 |
+
print("infer interval {0} - {1}".format(start, end))
|
63 |
+
save_est_roll = []
|
64 |
+
save_est_logit = []
|
65 |
+
infer_data = data[i:i+50]
|
66 |
+
for frame in infer_data:
|
67 |
+
file_list, label = frame
|
68 |
+
torch_input_img, torch_label = torch_preprocess(file_list, label)
|
69 |
+
logits = net(torch.unsqueeze(torch_input_img,dim=0))
|
70 |
+
print("####", torch_input_img.shape, torch_label.shape, logits.shape)
|
71 |
+
pred_label = torch.sigmoid(logits) >= 0.4
|
72 |
+
numpy_pre_label = pred_label.cpu().detach().numpy().astype(int)
|
73 |
+
numpy_logit = logits.cpu().detach().numpy()
|
74 |
+
save_est_roll.append(numpy_pre_label)
|
75 |
+
save_est_logit.append(numpy_logit)
|
76 |
+
# Roll prediction
|
77 |
+
target = np.zeros((50, 88))
|
78 |
+
target[:, min_key:max_key+1] = np.asarray(save_est_roll).squeeze()
|
79 |
+
save_est_roll = target
|
80 |
+
# Logit
|
81 |
+
target_ = np.zeros((50, 88))
|
82 |
+
target_[:, min_key:max_key + 1] = np.asarray(save_est_logit).squeeze()
|
83 |
+
save_est_logit = target_
|
84 |
+
# save both Roll predictions and logits as npz files
|
85 |
+
np.savez(f'{est_roll_folder}/' + str(start) + '-' + str(end) + '.npz', logit=save_est_logit, roll=save_est_roll)
|
86 |
+
i = i+50
|
87 |
+
|
88 |
+
def torch_preprocess(input_file_list, label):
|
89 |
+
input_img_list = []
|
90 |
+
for input_file in input_file_list:
|
91 |
+
input_img = Image.open(input_file).convert('L')
|
92 |
+
binarr = np.array(input_img)
|
93 |
+
input_img = Image.fromarray(binarr.astype(np.uint8))
|
94 |
+
input_img_list.append(input_img)
|
95 |
+
new_input_img_list = []
|
96 |
+
for input_img in input_img_list:
|
97 |
+
new_input_img_list.append(transform(input_img))
|
98 |
+
final_input_img = np.concatenate(new_input_img_list)
|
99 |
+
torch_input_img = torch.from_numpy(final_input_img).float().cuda()
|
100 |
+
torch_label = torch.from_numpy(label).float().cuda()
|
101 |
+
return torch_input_img, torch_label
|
102 |
+
|
103 |
+
|
104 |
+
if __name__ == "__main__":
|
105 |
+
model_path = './models/Video2Roll_50_0.4/14.pth' # change to your path
|
106 |
+
device = torch.device('cuda')
|
107 |
+
net = Video2RollNet.resnet18()
|
108 |
+
net.cuda()
|
109 |
+
net.load_state_dict(torch.load(model_path))
|
110 |
+
|
111 |
+
#training_data = [True,False]
|
112 |
+
training_data = [False]
|
113 |
+
# infer Roll predictions
|
114 |
+
folders = {}
|
115 |
+
|
116 |
+
train_img_folder = glob.glob(img_root +'/training/*')
|
117 |
+
train_img_folder.sort(key=lambda x:int(x.split('/')[-1]))
|
118 |
+
test_img_folder = glob.glob(img_root +'/testing/*')
|
119 |
+
test_img_folder.sort(key=lambda x:int(x.split('/')[-1]))
|
120 |
+
train_label_folder = glob.glob(label_root +'/training/*')
|
121 |
+
train_label_folder.sort(key=lambda x: int(x.split('/')[-1].split('.')[0]))
|
122 |
+
test_label_folder = glob.glob(label_root +'/testing/*')
|
123 |
+
test_label_folder.sort(key=lambda x: int(x.split('/')[-1].split('.')[0]))
|
124 |
+
train_midi_folder = glob.glob(midi_root +'/training/*')
|
125 |
+
train_midi_folder.sort(key=lambda x:int(x.split('/')[-1]))
|
126 |
+
test_midi_folder = glob.glob(midi_root +'/testing/*')
|
127 |
+
test_midi_folder.sort(key=lambda x:int(x.split('/')[-1]))
|
128 |
+
|
129 |
+
folders['train'] = [(train_img_folder[i],train_label_folder[i],train_midi_folder[i]) for i in range(len(train_img_folder))]
|
130 |
+
print(folders['train'])
|
131 |
+
folders['test'] = [(test_img_folder[i],test_label_folder[i],test_midi_folder[i]) for i in range(len(test_img_folder))]
|
132 |
+
print(folders['test'])
|
133 |
+
for item in training_data:
|
134 |
+
if item:
|
135 |
+
for img_folder, label_file, midi_folder in folders['train']:
|
136 |
+
est_roll_folder = midi_folder.replace('midi_npz','estimate_Roll_exp4')
|
137 |
+
#/ailab-train/speech/shansizhe/audeo/data/midi_npz/testing/2
|
138 |
+
print("save file in:", est_roll_folder)
|
139 |
+
os.makedirs(est_roll_folder, exist_ok=True)
|
140 |
+
intervals, data = load_data(img_folder, label_file, midi_folder)
|
141 |
+
print("starting inference--------------------")
|
142 |
+
inference(net,intervals, data, est_roll_folder)
|
143 |
+
else:
|
144 |
+
for img_folder, label_file, midi_folder in folders['test']:
|
145 |
+
est_roll_folder = midi_folder.replace('midi_npz','estimate_Roll_exp4')
|
146 |
+
print("save file in:", est_roll_folder)
|
147 |
+
os.makedirs(est_roll_folder, exist_ok=True)
|
148 |
+
intervals, data = load_data(img_folder, label_file, midi_folder)
|
149 |
+
print("starting inference--------------------")
|
150 |
+
inference(net, intervals, data, est_roll_folder)
|
151 |
+
|
src/audeo/Video2Roll_solver.py
ADDED
@@ -0,0 +1,204 @@
|
|
|
|
|
|
|
|
|
|
|
|
|
|
|
|
|
|
|
|
|
|
|
|
|
|
|
|
|
|
|
|
|
|
|
|
|
|
|
|
|
|
|
|
|
|
|
|
|
|
|
|
|
|
|
|
|
|
|
|
|
|
|
|
|
|
|
|
|
|
|
|
|
|
|
|
|
|
|
|
|
|
|
|
|
|
|
|
|
|
|
|
|
|
|
|
|
|
|
|
|
|
|
|
|
|
|
|
|
|
|
|
|
|
|
|
|
|
|
|
|
|
|
|
|
|
|
|
|
|
|
|
|
|
|
|
|
|
|
|
|
|
|
|
|
|
|
|
|
|
|
|
|
|
|
|
|
|
|
|
|
|
|
|
|
|
|
|
|
|
|
|
|
|
|
|
|
|
|
|
|
|
|
|
|
|
|
|
|
|
|
|
|
|
|
|
|
|
|
|
|
|
|
|
|
|
|
|
|
|
|
|
|
|
|
|
|
|
|
|
|
|
|
|
|
|
|
|
|
|
|
|
|
|
|
|
|
|
|
|
|
|
|
|
|
|
|
|
|
|
|
|
|
|
|
|
|
|
|
|
|
|
|
|
|
|
|
|
|
|
|
|
|
|
|
|
|
|
|
|
|
|
|
|
|
|
|
|
|
|
|
|
|
|
|
|
|
|
|
|
|
|
|
|
|
|
|
|
|
|
|
|
|
|
|
|
|
|
|
|
|
|
|
|
|
|
|
|
|
|
|
|
|
|
|
|
|
|
|
|
|
|
|
|
|
|
|
|
|
|
|
|
|
|
|
|
|
|
|
|
|
|
|
|
|
|
|
|
|
|
|
|
|
|
|
|
|
|
|
|
|
|
|
|
|
|
|
|
|
|
|
|
|
|
|
|
|
|
|
|
|
|
|
|
|
|
|
|
|
|
|
1 |
+
import time
|
2 |
+
import numpy as np
|
3 |
+
import torch
|
4 |
+
from sklearn import metrics
|
5 |
+
from sklearn.metrics import _classification
|
6 |
+
from torch.utils.tensorboard import SummaryWriter
|
7 |
+
from tqdm import tqdm
|
8 |
+
import os
|
9 |
+
|
10 |
+
class Solver(object):
|
11 |
+
|
12 |
+
def __init__(self, data_loader, test_data_loader, model, criterion, optimizer, lr_scheduler, epochs):
|
13 |
+
self.save_model_path = '/ailab-train/speech/shansizhe/audeo/models/Video2Roll_50_0.4/' # change to your path
|
14 |
+
self.test_loader = test_data_loader
|
15 |
+
self.data_loader = data_loader
|
16 |
+
self.net = model
|
17 |
+
self.criterion = criterion
|
18 |
+
self.optimizer = optimizer
|
19 |
+
self.lr_scheduler = lr_scheduler
|
20 |
+
# Training config
|
21 |
+
self.epochs = epochs
|
22 |
+
# logging
|
23 |
+
self.step = 0
|
24 |
+
self.global_step = 0
|
25 |
+
self.writer = SummaryWriter(log_dir='/ailab-train/speech/shansizhe/audeo/log/50_0.4/')
|
26 |
+
# visualizing loss using visdom
|
27 |
+
self.tr_loss = torch.Tensor(self.epochs)
|
28 |
+
self.val_loss = torch.zeros(self.epochs)
|
29 |
+
self.visdom = False
|
30 |
+
self.visdom_epoch = 1
|
31 |
+
self.visdom_id = 'key classification'
|
32 |
+
if self.visdom:
|
33 |
+
from visdom import Visdom
|
34 |
+
self.vis = Visdom(env=self.visdom_id)
|
35 |
+
self.vis_opts = dict(title=self.visdom_id,
|
36 |
+
ylabel='Loss', xlabel='Epoch',
|
37 |
+
legend=['train loss', 'val loss'])
|
38 |
+
self.vis_window = None
|
39 |
+
self.vis_epochs = torch.arange(1, self.epochs + 1)
|
40 |
+
|
41 |
+
def train(self):
|
42 |
+
# Train model multi-epoches
|
43 |
+
pre_val_loss = 1e4
|
44 |
+
for epoch in tqdm(range(self.epochs)):
|
45 |
+
print("Training...")
|
46 |
+
self.net.train() # Turn on BatchNorm & Dropout
|
47 |
+
start = time.time()
|
48 |
+
# training loop
|
49 |
+
tr_avg_loss, tr_avg_precision, tr_avg_recall = self.train_loop()
|
50 |
+
|
51 |
+
# evaluate
|
52 |
+
self.net.eval()
|
53 |
+
val_avg_loss, val_avg_precision, val_avg_recall, val_avg_acc, val_fscore = self.validate()
|
54 |
+
print('-' * 85)
|
55 |
+
print('Train Summary | Epoch {0} | Time {1:.2f}s | '
|
56 |
+
'Train Loss {2:.3f}'.format(
|
57 |
+
epoch+1, time.time() - start, tr_avg_loss, tr_avg_precision, tr_avg_recall))
|
58 |
+
print("epoch {0} validation loss:{1:.3f} | avg precision:{2:.3f} | avg recall:{3:.3f} | avg acc:{4:.3f} | f1 score:{5:.3f}".format(
|
59 |
+
epoch+1, val_avg_loss, val_avg_precision, val_avg_recall, val_avg_acc, val_fscore))
|
60 |
+
print('-' * 85)
|
61 |
+
|
62 |
+
# Log metrics to TensorBoard
|
63 |
+
self.writer.add_scalar('Loss/train', tr_avg_loss, epoch)
|
64 |
+
self.writer.add_scalar('Precision/train', tr_avg_precision, epoch)
|
65 |
+
self.writer.add_scalar('Recall/train', tr_avg_recall, epoch)
|
66 |
+
self.writer.add_scalar('Loss/val', val_avg_loss, epoch)
|
67 |
+
self.writer.add_scalar('Precision/val', val_avg_precision, epoch)
|
68 |
+
self.writer.add_scalar('Recall/val', val_avg_recall, epoch)
|
69 |
+
self.writer.add_scalar('Accuracy/val', val_avg_acc, epoch)
|
70 |
+
self.writer.add_scalar('F1_score/val', val_fscore, epoch)
|
71 |
+
|
72 |
+
os.makedirs(self.save_model_path, exist_ok=True)
|
73 |
+
model_save_path = f"{self.save_model_path}{epoch}.pth"
|
74 |
+
torch.save(self.net.state_dict(), model_save_path)
|
75 |
+
if val_avg_loss < pre_val_loss:
|
76 |
+
pre_val_loss = val_avg_loss
|
77 |
+
torch.save(self.net.state_dict(), f"{self.save_model_path}best.pth")
|
78 |
+
# Save model each epoch
|
79 |
+
self.val_loss[epoch] = val_avg_loss
|
80 |
+
self.tr_loss[epoch] = tr_avg_loss
|
81 |
+
|
82 |
+
# visualizing loss using visdom
|
83 |
+
if self.visdom:
|
84 |
+
x_axis = self.vis_epochs[0:epoch + 1]
|
85 |
+
# train_y_axis = self.tr_loss[0:epoch+1]
|
86 |
+
# val_x_axis = self.vis_epochs[0:epoch+1:10]
|
87 |
+
# val_y_axis = self.val_loss[0:epoch//10+1]
|
88 |
+
y_axis = torch.stack(
|
89 |
+
(self.tr_loss[0:epoch + 1], self.val_loss[0:epoch + 1]), dim=1)
|
90 |
+
if self.vis_window is None:
|
91 |
+
self.vis_window = self.vis.line(
|
92 |
+
X=x_axis,
|
93 |
+
Y=y_axis,
|
94 |
+
opts=self.vis_opts,
|
95 |
+
)
|
96 |
+
else:
|
97 |
+
self.vis.line(
|
98 |
+
X=x_axis.unsqueeze(0).expand(y_axis.size(
|
99 |
+
1), x_axis.size(0)).transpose(0, 1), # Visdom fix
|
100 |
+
Y=y_axis,
|
101 |
+
win=self.vis_window,
|
102 |
+
update='replace',
|
103 |
+
)
|
104 |
+
|
105 |
+
def train_loop(self):
|
106 |
+
data_loader = self.data_loader
|
107 |
+
epoch_loss = 0
|
108 |
+
epoch_precision = 0
|
109 |
+
epoch_recall = 0
|
110 |
+
count = 0
|
111 |
+
start = time.time()
|
112 |
+
|
113 |
+
for i, data in tqdm(enumerate(data_loader)):
|
114 |
+
imgs, label = data
|
115 |
+
logits = self.net(imgs)
|
116 |
+
loss = self.criterion(logits,label)
|
117 |
+
# set the threshold of the logits
|
118 |
+
pred_label = torch.sigmoid(logits) >= 0.4
|
119 |
+
numpy_label = label.cpu().detach().numpy().astype(int)
|
120 |
+
numpy_pre_label = pred_label.cpu().detach().numpy().astype(int)
|
121 |
+
|
122 |
+
precision = metrics.precision_score(numpy_label,numpy_pre_label, average='samples', zero_division=1)
|
123 |
+
recall = metrics.recall_score(numpy_label,numpy_pre_label, average='samples', zero_division=1)
|
124 |
+
|
125 |
+
self.writer.add_scalar('loss/step', loss, self.global_step)
|
126 |
+
self.writer.add_scalar('precision/step', precision, self.global_step)
|
127 |
+
self.writer.add_scalar('recall/step', recall, self.global_step)
|
128 |
+
|
129 |
+
if self.global_step % 100 == 0:
|
130 |
+
end = time.time()
|
131 |
+
print(
|
132 |
+
"step {0} loss:{1:.4f} | precision:{2:.3f} | recall:{3:.3f} | time:{4:.2f}".format(self.global_step, loss.item(), precision,
|
133 |
+
recall,end - start))
|
134 |
+
start = end
|
135 |
+
|
136 |
+
epoch_precision += precision
|
137 |
+
epoch_recall += recall
|
138 |
+
epoch_loss += loss.item()
|
139 |
+
self.optimizer.zero_grad()
|
140 |
+
loss.backward()
|
141 |
+
self.optimizer.step()
|
142 |
+
count += 1
|
143 |
+
self.global_step += 1
|
144 |
+
self.lr_scheduler.step(epoch_loss / count)
|
145 |
+
return epoch_loss/count, epoch_precision/count, epoch_recall/count
|
146 |
+
|
147 |
+
def validate(self):
|
148 |
+
epoch_loss = 0
|
149 |
+
count = 0
|
150 |
+
all_pred_label = []
|
151 |
+
all_label = []
|
152 |
+
with torch.no_grad():
|
153 |
+
for i, data in enumerate(self.test_loader):
|
154 |
+
imgs, label = data
|
155 |
+
logits = self.net(imgs)
|
156 |
+
loss = self.criterion(logits, label)
|
157 |
+
pred_label = torch.sigmoid(logits) >= 0.4
|
158 |
+
numpy_label = label.cpu().detach().numpy().astype(int)
|
159 |
+
numpy_pre_label = pred_label.cpu().detach().numpy().astype(int)
|
160 |
+
all_label.append(numpy_label)
|
161 |
+
all_pred_label.append(numpy_pre_label)
|
162 |
+
epoch_loss += loss.item()
|
163 |
+
count += 1
|
164 |
+
all_label = np.vstack(all_label)
|
165 |
+
all_pred_label = np.vstack(all_pred_label)
|
166 |
+
labels = _classification._check_set_wise_labels(all_label, all_pred_label,labels=None, pos_label=1, average='samples')
|
167 |
+
MCM = metrics.multilabel_confusion_matrix(all_label, all_pred_label,sample_weight=None, labels=labels, samplewise=True)
|
168 |
+
tp_sum = MCM[:, 1, 1]
|
169 |
+
fp_sum = MCM[:, 0, 1]
|
170 |
+
fn_sum = MCM[:, 1, 0]
|
171 |
+
# tn_sum = MCM[:, 0, 0]
|
172 |
+
accuracy = _prf_divide(tp_sum, tp_sum+fp_sum+fn_sum, zero_division=1)
|
173 |
+
accuracy = np.average(accuracy)
|
174 |
+
all_precision = metrics.precision_score(all_label, all_pred_label, average='samples', zero_division=1)
|
175 |
+
all_recall = metrics.recall_score(all_label, all_pred_label, average='samples', zero_division=1)
|
176 |
+
all_f1_score = metrics.f1_score(all_label, all_pred_label, average='samples', zero_division=1)
|
177 |
+
return epoch_loss/count, all_precision, all_recall, accuracy, all_f1_score
|
178 |
+
|
179 |
+
|
180 |
+
def _prf_divide(numerator, denominator, zero_division="warn"):
|
181 |
+
"""Performs division and handles divide-by-zero.
|
182 |
+
On zero-division, sets the corresponding result elements equal to
|
183 |
+
0 or 1 (according to ``zero_division``). Plus, if
|
184 |
+
``zero_division != "warn"`` raises a warning.
|
185 |
+
The metric, modifier and average arguments are used only for determining
|
186 |
+
an appropriate warning.
|
187 |
+
"""
|
188 |
+
mask = denominator == 0.0
|
189 |
+
denominator = denominator.copy()
|
190 |
+
denominator[mask] = 1 # avoid infs/nans
|
191 |
+
result = numerator / denominator
|
192 |
+
|
193 |
+
if not np.any(mask):
|
194 |
+
return result
|
195 |
+
|
196 |
+
# if ``zero_division=1``, set those with denominator == 0 equal to 1
|
197 |
+
result[mask] = 0.0 if zero_division in ["warn", 0] else 1.0
|
198 |
+
|
199 |
+
# the user will be removing warnings if zero_division is set to something
|
200 |
+
# different than its default value. If we are computing only f-score
|
201 |
+
# the warning will be raised only if precision and recall are ill-defined
|
202 |
+
if zero_division != "warn":
|
203 |
+
return result
|
204 |
+
|
src/audeo/Video2Roll_train.py
ADDED
@@ -0,0 +1,26 @@
|
|
|
|
|
|
|
|
|
|
|
|
|
|
|
|
|
|
|
|
|
|
|
|
|
|
|
|
|
|
|
|
|
|
|
|
|
|
|
|
|
|
|
|
|
|
|
|
|
|
|
|
|
|
|
1 |
+
from Video2Roll_dataset import Video2RollDataset
|
2 |
+
from torch.utils.data import DataLoader
|
3 |
+
import torch
|
4 |
+
from torch import optim
|
5 |
+
|
6 |
+
import Video2RollNet
|
7 |
+
|
8 |
+
from Video2Roll_solver import Solver
|
9 |
+
import torch.nn as nn
|
10 |
+
from balance_data import MultilabelBalancedRandomSampler
|
11 |
+
|
12 |
+
if __name__ == "__main__":
|
13 |
+
train_dataset = Video2RollDataset(subset='train')
|
14 |
+
train_sampler = MultilabelBalancedRandomSampler(train_dataset.train_labels)
|
15 |
+
train_data_loader = DataLoader(train_dataset, batch_size=64, sampler=train_sampler)
|
16 |
+
test_dataset = Video2RollDataset(subset='test')
|
17 |
+
test_data_loader = DataLoader(test_dataset, batch_size=64)
|
18 |
+
device = torch.device('cuda:6')
|
19 |
+
|
20 |
+
net = Video2RollNet.resnet18()
|
21 |
+
net.cuda()
|
22 |
+
optimizer = optim.Adam(net.parameters(), lr=0.001, betas=(0.9, 0.999))
|
23 |
+
criterion = nn.BCEWithLogitsLoss()
|
24 |
+
scheduler = optim.lr_scheduler.ReduceLROnPlateau(optimizer, 'min', patience=2)
|
25 |
+
solver = Solver(train_data_loader, test_data_loader, net, criterion, optimizer, scheduler, epochs=50)
|
26 |
+
solver.train()
|
src/audeo/Video_Id.md
ADDED
@@ -0,0 +1,30 @@
|
|
|
|
|
|
|
|
|
|
|
|
|
|
|
|
|
|
|
|
|
|
|
|
|
|
|
|
|
|
|
|
|
|
|
|
|
|
|
|
|
|
|
|
|
|
|
|
|
|
|
|
|
|
|
|
|
|
|
|
|
|
|
1 |
+
# Training
|
2 |
+
- https://youtu.be/_3qnL9ddHuw
|
3 |
+
- https://youtu.be/HB8-w5CvMls
|
4 |
+
- https://youtu.be/vGdV4mJhaKU
|
5 |
+
- https://youtu.be/W5lOLZsjOp8
|
6 |
+
- https://youtu.be/vHi3_k4XOrA
|
7 |
+
- https://youtu.be/PIS76X17Mf8
|
8 |
+
- https://youtu.be/DMdJLEGrUrg
|
9 |
+
- https://youtu.be/xXwCryMItHs
|
10 |
+
- https://youtu.be/49dCBsIGsgY
|
11 |
+
- https://youtu.be/OZVMVVQPPPI
|
12 |
+
- https://youtu.be/cAnmwgC-JRw
|
13 |
+
- https://youtu.be/w77mBaWOOh0
|
14 |
+
- https://youtu.be/MGMxImcYhiI
|
15 |
+
- https://youtu.be/WqFyqbD9VEQ
|
16 |
+
- https://youtu.be/V0P_2QG84MM
|
17 |
+
- https://youtu.be/1eEcy3MgqxA
|
18 |
+
- https://youtu.be/GH-kkZQQ8G8
|
19 |
+
- https://youtu.be/Kk58v56rD0s
|
20 |
+
- https://youtu.be/WWqRR7RZGXw
|
21 |
+
- https://youtu.be/ouhp7O3Sz8M
|
22 |
+
- https://youtu.be/U0v4CckNE68
|
23 |
+
- https://youtu.be/VaqWF70DjYs
|
24 |
+
- https://youtu.be/m2yadhLP8H8
|
25 |
+
- https://youtu.be/wRJlm0lCyoI
|
26 |
+
|
27 |
+
# Testing
|
28 |
+
- https://youtu.be/u5nBBJndN3I
|
29 |
+
- https://youtu.be/nwwHuxHMIpc
|
30 |
+
- https://youtu.be/ra1jf2nzJPg
|
src/audeo/balance_data.py
ADDED
@@ -0,0 +1,91 @@
|
|
|
|
|
|
|
|
|
|
|
|
|
|
|
|
|
|
|
|
|
|
|
|
|
|
|
|
|
|
|
|
|
|
|
|
|
|
|
|
|
|
|
|
|
|
|
|
|
|
|
|
|
|
|
|
|
|
|
|
|
|
|
|
|
|
|
|
|
|
|
|
|
|
|
|
|
|
|
|
|
|
|
|
|
|
|
|
|
|
|
|
|
|
|
|
|
|
|
|
|
|
|
|
|
|
|
|
|
|
|
|
|
|
|
|
|
|
|
|
|
|
|
|
|
|
|
|
|
|
|
|
|
|
|
|
|
|
|
|
|
|
|
|
|
|
|
|
|
|
|
|
|
|
|
|
|
|
|
|
|
|
|
|
|
|
|
|
|
|
|
|
|
|
|
|
|
|
|
|
|
|
|
|
|
1 |
+
import random
|
2 |
+
import numpy as np
|
3 |
+
import torch
|
4 |
+
from torch.utils.data.sampler import Sampler
|
5 |
+
# torch.cuda.set_device(1)
|
6 |
+
|
7 |
+
class MultilabelBalancedRandomSampler(Sampler):
|
8 |
+
"""
|
9 |
+
MultilabelBalancedRandomSampler: Given a multilabel dataset of length n_samples and
|
10 |
+
number of classes n_classes, samples from the data with equal probability per class
|
11 |
+
effectively oversampling minority classes and undersampling majority classes at the
|
12 |
+
same time. Note that using this sampler does not guarantee that the distribution of
|
13 |
+
classes in the output samples will be uniform, since the dataset is multilabel and
|
14 |
+
sampling is based on a single class. This does however guarantee that all classes
|
15 |
+
will have at least batch_size / n_classes samples as batch_size approaches infinity
|
16 |
+
"""
|
17 |
+
|
18 |
+
def __init__(self, labels, indices=None, class_choice="random"):
|
19 |
+
"""
|
20 |
+
Parameters:
|
21 |
+
-----------
|
22 |
+
labels: a multi-hot encoding numpy array of shape (n_samples, n_classes)
|
23 |
+
indices: an arbitrary-length 1-dimensional numpy array representing a list
|
24 |
+
of indices to sample only from.
|
25 |
+
class_choice: a string indicating how class will be selected for every
|
26 |
+
sample.
|
27 |
+
"random": class is chosen uniformly at random.
|
28 |
+
"cycle": the sampler cycles through the classes sequentially.
|
29 |
+
"""
|
30 |
+
self.labels = labels
|
31 |
+
self.indices = indices
|
32 |
+
if self.indices is None:
|
33 |
+
self.indices = range(len(labels))
|
34 |
+
self.map = []
|
35 |
+
for class_ in range(self.labels.shape[1]):
|
36 |
+
lst = np.where(self.labels[:, class_] == 1)[0]
|
37 |
+
lst = lst[np.isin(lst, self.indices)]
|
38 |
+
self.map.append(lst)
|
39 |
+
all_zero = []
|
40 |
+
for row in range(self.labels.shape[0]):
|
41 |
+
if not np.any(labels[row]):
|
42 |
+
all_zero.append(row)
|
43 |
+
|
44 |
+
print("all zero sample number is: ",len(all_zero))
|
45 |
+
self.map.append(all_zero)
|
46 |
+
print("counting-----")
|
47 |
+
for i in range(len(self.map)):
|
48 |
+
print("class {0} has {1} samples:".format(i,len(self.map[i])))
|
49 |
+
|
50 |
+
assert class_choice in ["random", "cycle"]
|
51 |
+
self.class_choice = class_choice
|
52 |
+
self.current_class = 0
|
53 |
+
|
54 |
+
def __iter__(self):
|
55 |
+
self.count = 0
|
56 |
+
return self
|
57 |
+
|
58 |
+
def __next__(self):
|
59 |
+
# if self.count >= len(self.indices):
|
60 |
+
if self.count >= 20000:
|
61 |
+
raise StopIteration
|
62 |
+
self.count += 1
|
63 |
+
return self.sample()
|
64 |
+
|
65 |
+
def sample(self):
|
66 |
+
if self.class_choice == "random":
|
67 |
+
class_ = random.randint(0, self.labels.shape[1])# - 1)
|
68 |
+
# print(class_)
|
69 |
+
elif self.class_choice == "cycle":
|
70 |
+
class_ = self.current_class
|
71 |
+
self.current_class = (self.current_class + 1) % self.labels.shape[1]
|
72 |
+
class_indices = self.map[class_]
|
73 |
+
return np.random.choice(class_indices)
|
74 |
+
|
75 |
+
def __len__(self):
|
76 |
+
return 20000
|
77 |
+
# return len(self.indices)
|
78 |
+
|
79 |
+
# if __name__ == "__main__":
|
80 |
+
# train_dataset = Video2RollDataset(subset='train')
|
81 |
+
# train_sampler = MultilabelBalancedRandomSampler(train_dataset.train_labels)
|
82 |
+
# train_data_loader = DataLoader(train_dataset, batch_size=64, sampler=train_sampler)
|
83 |
+
# for i, data in enumerate(train_data_loader):
|
84 |
+
# print(i)
|
85 |
+
# imgs,label,ref_imgs,rng = data
|
86 |
+
# print(torch.unique(torch.nonzero(label)[:,1]))
|
87 |
+
# for j in range(len(label)):
|
88 |
+
# if label[j].sum()==0:
|
89 |
+
# print("yes")
|
90 |
+
# if i == 1:
|
91 |
+
# break
|
src/audeo/models/Video2Roll_50_0.4/14.pth
ADDED
@@ -0,0 +1,3 @@
|
|
|
|
|
|
|
|
|
1 |
+
version https://git-lfs.github.com/spec/v1
|
2 |
+
oid sha256:c0e46b8dcf33cb6bf953fe09326edb0bbdcf06b697f64a6f448e3baa42bd822c
|
3 |
+
size 50945493
|
src/audeo/piano_coords.py
ADDED
@@ -0,0 +1,9 @@
|
|
|
|
|
|
|
|
|
|
|
|
|
|
|
|
|
|
|
|
|
1 |
+
# upper_left_x, upper_left_y, lower_right_x, lower_right_y
|
2 |
+
train_piano_coords = [(68,674,1869,863), (38,680,1882,875), (42,678,1870,874), (42,678,1870,874),
|
3 |
+
(44,670,1876,865), (35,678,1875,869), (30,451,1249,583), (28,454,1254,584),
|
4 |
+
(39,678,1886,881), (33,671,1886,860), (29,446,1252,576), (26,447,1252,577),
|
5 |
+
(42,673,1879,871), (43,669,1870,869), (45,675,1864,870), (53,674,1868,860),
|
6 |
+
(51,679,1866,866), (51,674,1861,861), (48,674,1878,861), (45,671,1879,870),
|
7 |
+
(50,671,1879,866), (54,670,1864,863), (50,670,1870,867), (43,673,1882,869)]
|
8 |
+
|
9 |
+
test_piano_coords = [(41,679,1880,881), (43,675,1883,875), (40,671,1879,871)]
|
src/audeo/thumbnail_image.png
ADDED
![]() |
Git LFS Details
|
src/audeo/videomae_fintune.ipynb
ADDED
The diff for this file is too large to render.
See raw diff
|
|
src/audioldm/__init__.py
ADDED
@@ -0,0 +1,8 @@
|
|
|
|
|
|
|
|
|
|
|
|
|
|
|
|
|
|
|
1 |
+
from .ldm import LatentDiffusion
|
2 |
+
from .utils import seed_everything, save_wave, get_time, get_duration
|
3 |
+
from .pipeline import *
|
4 |
+
|
5 |
+
|
6 |
+
|
7 |
+
|
8 |
+
|
src/audioldm/__main__.py
ADDED
@@ -0,0 +1,183 @@
|
|
|
|
|
|
|
|
|
|
|
|
|
|
|
|
|
|
|
|
|
|
|
|
|
|
|
|
|
|
|
|
|
|
|
|
|
|
|
|
|
|
|
|
|
|
|
|
|
|
|
|
|
|
|
|
|
|
|
|
|
|
|
|
|
|
|
|
|
|
|
|
|
|
|
|
|
|
|
|
|
|
|
|
|
|
|
|
|
|
|
|
|
|
|
|
|
|
|
|
|
|
|
|
|
|
|
|
|
|
|
|
|
|
|
|
|
|
|
|
|
|
|
|
|
|
|
|
|
|
|
|
|
|
|
|
|
|
|
|
|
|
|
|
|
|
|
|
|
|
|
|
|
|
|
|
|
|
|
|
|
|
|
|
|
|
|
|
|
|
|
|
|
|
|
|
|
|
|
|
|
|
|
|
|
|
|
|
|
|
|
|
|
|
|
|
|
|
|
|
|
|
|
|
|
|
|
|
|
|
|
|
|
|
|
|
|
|
|
|
|
|
|
|
|
|
|
|
|
|
|
|
|
|
|
|
|
|
|
|
|
|
|
|
|
|
|
|
|
|
|
|
|
|
|
|
|
|
|
|
|
|
|
|
|
|
|
|
|
|
|
|
|
|
|
|
|
|
|
|
|
|
|
|
|
|
|
|
|
|
|
|
|
|
|
|
|
|
|
|
|
|
|
|
|
|
|
|
|
|
|
|
|
|
|
|
|
|
|
|
|
|
|
|
|
|
|
|
|
|
|
|
|
|
|
|
|
|
|
|
|
|
|
|
|
|
|
|
|
|
|
|
|
|
|
|
|
|
|
|
|
|
|
|
|
|
|
|
|
1 |
+
#!/usr/bin/python3
|
2 |
+
import os
|
3 |
+
from audioldm import text_to_audio, style_transfer, build_model, save_wave, get_time, round_up_duration, get_duration
|
4 |
+
import argparse
|
5 |
+
|
6 |
+
CACHE_DIR = os.getenv(
|
7 |
+
"AUDIOLDM_CACHE_DIR",
|
8 |
+
os.path.join(os.path.expanduser("~"), ".cache/audioldm"))
|
9 |
+
|
10 |
+
parser = argparse.ArgumentParser()
|
11 |
+
|
12 |
+
parser.add_argument(
|
13 |
+
"--mode",
|
14 |
+
type=str,
|
15 |
+
required=False,
|
16 |
+
default="generation",
|
17 |
+
help="generation: text-to-audio generation; transfer: style transfer",
|
18 |
+
choices=["generation", "transfer"]
|
19 |
+
)
|
20 |
+
|
21 |
+
parser.add_argument(
|
22 |
+
"-t",
|
23 |
+
"--text",
|
24 |
+
type=str,
|
25 |
+
required=False,
|
26 |
+
default="",
|
27 |
+
help="Text prompt to the model for audio generation",
|
28 |
+
)
|
29 |
+
|
30 |
+
parser.add_argument(
|
31 |
+
"-f",
|
32 |
+
"--file_path",
|
33 |
+
type=str,
|
34 |
+
required=False,
|
35 |
+
default=None,
|
36 |
+
help="(--mode transfer): Original audio file for style transfer; Or (--mode generation): the guidance audio file for generating simialr audio",
|
37 |
+
)
|
38 |
+
|
39 |
+
parser.add_argument(
|
40 |
+
"--transfer_strength",
|
41 |
+
type=float,
|
42 |
+
required=False,
|
43 |
+
default=0.5,
|
44 |
+
help="A value between 0 and 1. 0 means original audio without transfer, 1 means completely transfer to the audio indicated by text",
|
45 |
+
)
|
46 |
+
|
47 |
+
parser.add_argument(
|
48 |
+
"-s",
|
49 |
+
"--save_path",
|
50 |
+
type=str,
|
51 |
+
required=False,
|
52 |
+
help="The path to save model output",
|
53 |
+
default="./output",
|
54 |
+
)
|
55 |
+
|
56 |
+
parser.add_argument(
|
57 |
+
"--model_name",
|
58 |
+
type=str,
|
59 |
+
required=False,
|
60 |
+
help="The checkpoint you gonna use",
|
61 |
+
default="audioldm-s-full",
|
62 |
+
choices=["audioldm-s-full", "audioldm-l-full", "audioldm-s-full-v2"]
|
63 |
+
)
|
64 |
+
|
65 |
+
parser.add_argument(
|
66 |
+
"-ckpt",
|
67 |
+
"--ckpt_path",
|
68 |
+
type=str,
|
69 |
+
required=False,
|
70 |
+
help="The path to the pretrained .ckpt model",
|
71 |
+
default=None,
|
72 |
+
)
|
73 |
+
|
74 |
+
parser.add_argument(
|
75 |
+
"-b",
|
76 |
+
"--batchsize",
|
77 |
+
type=int,
|
78 |
+
required=False,
|
79 |
+
default=1,
|
80 |
+
help="Generate how many samples at the same time",
|
81 |
+
)
|
82 |
+
|
83 |
+
parser.add_argument(
|
84 |
+
"--ddim_steps",
|
85 |
+
type=int,
|
86 |
+
required=False,
|
87 |
+
default=200,
|
88 |
+
help="The sampling step for DDIM",
|
89 |
+
)
|
90 |
+
|
91 |
+
parser.add_argument(
|
92 |
+
"-gs",
|
93 |
+
"--guidance_scale",
|
94 |
+
type=float,
|
95 |
+
required=False,
|
96 |
+
default=2.5,
|
97 |
+
help="Guidance scale (Large => better quality and relavancy to text; Small => better diversity)",
|
98 |
+
)
|
99 |
+
|
100 |
+
parser.add_argument(
|
101 |
+
"-dur",
|
102 |
+
"--duration",
|
103 |
+
type=float,
|
104 |
+
required=False,
|
105 |
+
default=10.0,
|
106 |
+
help="The duration of the samples",
|
107 |
+
)
|
108 |
+
|
109 |
+
parser.add_argument(
|
110 |
+
"-n",
|
111 |
+
"--n_candidate_gen_per_text",
|
112 |
+
type=int,
|
113 |
+
required=False,
|
114 |
+
default=3,
|
115 |
+
help="Automatic quality control. This number control the number of candidates (e.g., generate three audios and choose the best to show you). A Larger value usually lead to better quality with heavier computation",
|
116 |
+
)
|
117 |
+
|
118 |
+
parser.add_argument(
|
119 |
+
"--seed",
|
120 |
+
type=int,
|
121 |
+
required=False,
|
122 |
+
default=42,
|
123 |
+
help="Change this value (any integer number) will lead to a different generation result.",
|
124 |
+
)
|
125 |
+
|
126 |
+
args = parser.parse_args()
|
127 |
+
|
128 |
+
if(args.ckpt_path is not None):
|
129 |
+
print("Warning: ckpt_path has no effect after version 0.0.20.")
|
130 |
+
|
131 |
+
assert args.duration % 2.5 == 0, "Duration must be a multiple of 2.5"
|
132 |
+
|
133 |
+
mode = args.mode
|
134 |
+
if(mode == "generation" and args.file_path is not None):
|
135 |
+
mode = "generation_audio_to_audio"
|
136 |
+
if(len(args.text) > 0):
|
137 |
+
print("Warning: You have specified the --file_path. --text will be ignored")
|
138 |
+
args.text = ""
|
139 |
+
|
140 |
+
save_path = os.path.join(args.save_path, mode)
|
141 |
+
|
142 |
+
if(args.file_path is not None):
|
143 |
+
save_path = os.path.join(save_path, os.path.basename(args.file_path.split(".")[0]))
|
144 |
+
|
145 |
+
text = args.text
|
146 |
+
random_seed = args.seed
|
147 |
+
duration = args.duration
|
148 |
+
guidance_scale = args.guidance_scale
|
149 |
+
n_candidate_gen_per_text = args.n_candidate_gen_per_text
|
150 |
+
|
151 |
+
os.makedirs(save_path, exist_ok=True)
|
152 |
+
audioldm = build_model(model_name=args.model_name)
|
153 |
+
|
154 |
+
if(args.mode == "generation"):
|
155 |
+
waveform = text_to_audio(
|
156 |
+
audioldm,
|
157 |
+
text,
|
158 |
+
args.file_path,
|
159 |
+
random_seed,
|
160 |
+
duration=duration,
|
161 |
+
guidance_scale=guidance_scale,
|
162 |
+
ddim_steps=args.ddim_steps,
|
163 |
+
n_candidate_gen_per_text=n_candidate_gen_per_text,
|
164 |
+
batchsize=args.batchsize,
|
165 |
+
)
|
166 |
+
|
167 |
+
elif(args.mode == "transfer"):
|
168 |
+
assert args.file_path is not None
|
169 |
+
assert os.path.exists(args.file_path), "The original audio file \'%s\' for style transfer does not exist." % args.file_path
|
170 |
+
waveform = style_transfer(
|
171 |
+
audioldm,
|
172 |
+
text,
|
173 |
+
args.file_path,
|
174 |
+
args.transfer_strength,
|
175 |
+
random_seed,
|
176 |
+
duration=duration,
|
177 |
+
guidance_scale=guidance_scale,
|
178 |
+
ddim_steps=args.ddim_steps,
|
179 |
+
batchsize=args.batchsize,
|
180 |
+
)
|
181 |
+
waveform = waveform[:,None,:]
|
182 |
+
|
183 |
+
save_wave(waveform, save_path, name="%s_%s" % (get_time(), text))
|
src/audioldm/audio/__init__.py
ADDED
@@ -0,0 +1,2 @@
|
|
|
|
|
|
|
1 |
+
from .tools import wav_to_fbank, read_wav_file
|
2 |
+
from .stft import TacotronSTFT
|
src/audioldm/audio/audio_processing.py
ADDED
@@ -0,0 +1,100 @@
|
|
|
|
|
|
|
|
|
|
|
|
|
|
|
|
|
|
|
|
|
|
|
|
|
|
|
|
|
|
|
|
|
|
|
|
|
|
|
|
|
|
|
|
|
|
|
|
|
|
|
|
|
|
|
|
|
|
|
|
|
|
|
|
|
|
|
|
|
|
|
|
|
|
|
|
|
|
|
|
|
|
|
|
|
|
|
|
|
|
|
|
|
|
|
|
|
|
|
|
|
|
|
|
|
|
|
|
|
|
|
|
|
|
|
|
|
|
|
|
|
|
|
|
|
|
|
|
|
|
|
|
|
|
|
|
|
|
|
|
|
|
|
|
|
|
|
|
|
|
|
|
|
|
|
|
|
|
|
|
|
|
|
|
|
|
|
|
|
|
|
|
|
|
|
|
|
|
|
|
|
|
|
|
|
|
|
|
|
|
|
|
|
|
|
|
|
|
|
|
|
|
|
1 |
+
import torch
|
2 |
+
import numpy as np
|
3 |
+
import librosa.util as librosa_util
|
4 |
+
from scipy.signal import get_window
|
5 |
+
|
6 |
+
|
7 |
+
def window_sumsquare(
|
8 |
+
window,
|
9 |
+
n_frames,
|
10 |
+
hop_length,
|
11 |
+
win_length,
|
12 |
+
n_fft,
|
13 |
+
dtype=np.float32,
|
14 |
+
norm=None,
|
15 |
+
):
|
16 |
+
"""
|
17 |
+
# from librosa 0.6
|
18 |
+
Compute the sum-square envelope of a window function at a given hop length.
|
19 |
+
|
20 |
+
This is used to estimate modulation effects induced by windowing
|
21 |
+
observations in short-time fourier transforms.
|
22 |
+
|
23 |
+
Parameters
|
24 |
+
----------
|
25 |
+
window : string, tuple, number, callable, or list-like
|
26 |
+
Window specification, as in `get_window`
|
27 |
+
|
28 |
+
n_frames : int > 0
|
29 |
+
The number of analysis frames
|
30 |
+
|
31 |
+
hop_length : int > 0
|
32 |
+
The number of samples to advance between frames
|
33 |
+
|
34 |
+
win_length : [optional]
|
35 |
+
The length of the window function. By default, this matches `n_fft`.
|
36 |
+
|
37 |
+
n_fft : int > 0
|
38 |
+
The length of each analysis frame.
|
39 |
+
|
40 |
+
dtype : np.dtype
|
41 |
+
The data type of the output
|
42 |
+
|
43 |
+
Returns
|
44 |
+
-------
|
45 |
+
wss : np.ndarray, shape=`(n_fft + hop_length * (n_frames - 1))`
|
46 |
+
The sum-squared envelope of the window function
|
47 |
+
"""
|
48 |
+
if win_length is None:
|
49 |
+
win_length = n_fft
|
50 |
+
|
51 |
+
n = n_fft + hop_length * (n_frames - 1)
|
52 |
+
x = np.zeros(n, dtype=dtype)
|
53 |
+
|
54 |
+
# Compute the squared window at the desired length
|
55 |
+
win_sq = get_window(window, win_length, fftbins=True)
|
56 |
+
win_sq = librosa_util.normalize(win_sq, norm=norm) ** 2
|
57 |
+
win_sq = librosa_util.pad_center(win_sq, n_fft)
|
58 |
+
|
59 |
+
# Fill the envelope
|
60 |
+
for i in range(n_frames):
|
61 |
+
sample = i * hop_length
|
62 |
+
x[sample : min(n, sample + n_fft)] += win_sq[: max(0, min(n_fft, n - sample))]
|
63 |
+
return x
|
64 |
+
|
65 |
+
|
66 |
+
def griffin_lim(magnitudes, stft_fn, n_iters=30):
|
67 |
+
"""
|
68 |
+
PARAMS
|
69 |
+
------
|
70 |
+
magnitudes: spectrogram magnitudes
|
71 |
+
stft_fn: STFT class with transform (STFT) and inverse (ISTFT) methods
|
72 |
+
"""
|
73 |
+
|
74 |
+
angles = np.angle(np.exp(2j * np.pi * np.random.rand(*magnitudes.size())))
|
75 |
+
angles = angles.astype(np.float32)
|
76 |
+
angles = torch.autograd.Variable(torch.from_numpy(angles))
|
77 |
+
signal = stft_fn.inverse(magnitudes, angles).squeeze(1)
|
78 |
+
|
79 |
+
for i in range(n_iters):
|
80 |
+
_, angles = stft_fn.transform(signal)
|
81 |
+
signal = stft_fn.inverse(magnitudes, angles).squeeze(1)
|
82 |
+
return signal
|
83 |
+
|
84 |
+
|
85 |
+
def dynamic_range_compression(x, normalize_fun=torch.log, C=1, clip_val=1e-5):
|
86 |
+
"""
|
87 |
+
PARAMS
|
88 |
+
------
|
89 |
+
C: compression factor
|
90 |
+
"""
|
91 |
+
return normalize_fun(torch.clamp(x, min=clip_val) * C)
|
92 |
+
|
93 |
+
|
94 |
+
def dynamic_range_decompression(x, C=1):
|
95 |
+
"""
|
96 |
+
PARAMS
|
97 |
+
------
|
98 |
+
C: compression factor used to compress
|
99 |
+
"""
|
100 |
+
return torch.exp(x) / C
|
src/audioldm/audio/stft.py
ADDED
@@ -0,0 +1,186 @@
|
|
|
|
|
|
|
|
|
|
|
|
|
|
|
|
|
|
|
|
|
|
|
|
|
|
|
|
|
|
|
|
|
|
|
|
|
|
|
|
|
|
|
|
|
|
|
|
|
|
|
|
|
|
|
|
|
|
|
|
|
|
|
|
|
|
|
|
|
|
|
|
|
|
|
|
|
|
|
|
|
|
|
|
|
|
|
|
|
|
|
|
|
|
|
|
|
|
|
|
|
|
|
|
|
|
|
|
|
|
|
|
|
|
|
|
|
|
|
|
|
|
|
|
|
|
|
|
|
|
|
|
|
|
|
|
|
|
|
|
|
|
|
|
|
|
|
|
|
|
|
|
|
|
|
|
|
|
|
|
|
|
|
|
|
|
|
|
|
|
|
|
|
|
|
|
|
|
|
|
|
|
|
|
|
|
|
|
|
|
|
|
|
|
|
|
|
|
|
|
|
|
|
|
|
|
|
|
|
|
|
|
|
|
|
|
|
|
|
|
|
|
|
|
|
|
|
|
|
|
|
|
|
|
|
|
|
|
|
|
|
|
|
|
|
|
|
|
|
|
|
|
|
|
|
|
|
|
|
|
|
|
|
|
|
|
|
|
|
|
|
|
|
|
|
|
|
|
|
|
|
|
|
|
|
|
|
|
|
|
|
|
|
|
|
|
|
|
|
|
|
|
|
|
|
|
|
|
|
|
|
|
|
|
|
|
|
|
|
|
|
|
|
|
|
|
|
|
|
|
|
|
|
|
|
|
|
|
|
|
|
|
|
|
|
|
|
|
|
|
|
|
|
|
|
|
|
|
|
|
|
|
|
|
|
|
|
|
|
|
|
|
|
|
|
1 |
+
import torch
|
2 |
+
import torch.nn.functional as F
|
3 |
+
import numpy as np
|
4 |
+
from scipy.signal import get_window
|
5 |
+
from librosa.util import pad_center, tiny
|
6 |
+
from librosa.filters import mel as librosa_mel_fn
|
7 |
+
|
8 |
+
from audioldm.audio.audio_processing import (
|
9 |
+
dynamic_range_compression,
|
10 |
+
dynamic_range_decompression,
|
11 |
+
window_sumsquare,
|
12 |
+
)
|
13 |
+
|
14 |
+
|
15 |
+
class STFT(torch.nn.Module):
|
16 |
+
"""adapted from Prem Seetharaman's https://github.com/pseeth/pytorch-stft"""
|
17 |
+
|
18 |
+
def __init__(self, filter_length, hop_length, win_length, window="hann"):
|
19 |
+
super(STFT, self).__init__()
|
20 |
+
self.filter_length = filter_length
|
21 |
+
self.hop_length = hop_length
|
22 |
+
self.win_length = win_length
|
23 |
+
self.window = window
|
24 |
+
self.forward_transform = None
|
25 |
+
scale = self.filter_length / self.hop_length
|
26 |
+
fourier_basis = np.fft.fft(np.eye(self.filter_length))
|
27 |
+
|
28 |
+
cutoff = int((self.filter_length / 2 + 1))
|
29 |
+
fourier_basis = np.vstack(
|
30 |
+
[np.real(fourier_basis[:cutoff, :]), np.imag(fourier_basis[:cutoff, :])]
|
31 |
+
)
|
32 |
+
|
33 |
+
forward_basis = torch.FloatTensor(fourier_basis[:, None, :])
|
34 |
+
inverse_basis = torch.FloatTensor(
|
35 |
+
np.linalg.pinv(scale * fourier_basis).T[:, None, :]
|
36 |
+
)
|
37 |
+
|
38 |
+
if window is not None:
|
39 |
+
assert filter_length >= win_length
|
40 |
+
# get window and zero center pad it to filter_length
|
41 |
+
fft_window = get_window(window, win_length, fftbins=True)
|
42 |
+
fft_window = pad_center(fft_window, filter_length)
|
43 |
+
fft_window = torch.from_numpy(fft_window).float()
|
44 |
+
|
45 |
+
# window the bases
|
46 |
+
forward_basis *= fft_window
|
47 |
+
inverse_basis *= fft_window
|
48 |
+
|
49 |
+
self.register_buffer("forward_basis", forward_basis.float())
|
50 |
+
self.register_buffer("inverse_basis", inverse_basis.float())
|
51 |
+
|
52 |
+
def transform(self, input_data):
|
53 |
+
device = self.forward_basis.device
|
54 |
+
input_data = input_data.to(device)
|
55 |
+
|
56 |
+
num_batches = input_data.size(0)
|
57 |
+
num_samples = input_data.size(1)
|
58 |
+
|
59 |
+
self.num_samples = num_samples
|
60 |
+
|
61 |
+
# similar to librosa, reflect-pad the input
|
62 |
+
input_data = input_data.view(num_batches, 1, num_samples)
|
63 |
+
input_data = F.pad(
|
64 |
+
input_data.unsqueeze(1),
|
65 |
+
(int(self.filter_length / 2), int(self.filter_length / 2), 0, 0),
|
66 |
+
mode="reflect",
|
67 |
+
)
|
68 |
+
input_data = input_data.squeeze(1)
|
69 |
+
|
70 |
+
forward_transform = F.conv1d(
|
71 |
+
input_data,
|
72 |
+
torch.autograd.Variable(self.forward_basis, requires_grad=False),
|
73 |
+
stride=self.hop_length,
|
74 |
+
padding=0,
|
75 |
+
)#.cpu()
|
76 |
+
|
77 |
+
cutoff = int((self.filter_length / 2) + 1)
|
78 |
+
real_part = forward_transform[:, :cutoff, :]
|
79 |
+
imag_part = forward_transform[:, cutoff:, :]
|
80 |
+
|
81 |
+
magnitude = torch.sqrt(real_part**2 + imag_part**2)
|
82 |
+
phase = torch.autograd.Variable(torch.atan2(imag_part.data, real_part.data))
|
83 |
+
|
84 |
+
return magnitude, phase
|
85 |
+
|
86 |
+
def inverse(self, magnitude, phase):
|
87 |
+
device = self.forward_basis.device
|
88 |
+
magnitude, phase = magnitude.to(device), phase.to(device)
|
89 |
+
|
90 |
+
recombine_magnitude_phase = torch.cat(
|
91 |
+
[magnitude * torch.cos(phase), magnitude * torch.sin(phase)], dim=1
|
92 |
+
)
|
93 |
+
|
94 |
+
inverse_transform = F.conv_transpose1d(
|
95 |
+
recombine_magnitude_phase,
|
96 |
+
torch.autograd.Variable(self.inverse_basis, requires_grad=False),
|
97 |
+
stride=self.hop_length,
|
98 |
+
padding=0,
|
99 |
+
)
|
100 |
+
|
101 |
+
if self.window is not None:
|
102 |
+
window_sum = window_sumsquare(
|
103 |
+
self.window,
|
104 |
+
magnitude.size(-1),
|
105 |
+
hop_length=self.hop_length,
|
106 |
+
win_length=self.win_length,
|
107 |
+
n_fft=self.filter_length,
|
108 |
+
dtype=np.float32,
|
109 |
+
)
|
110 |
+
# remove modulation effects
|
111 |
+
approx_nonzero_indices = torch.from_numpy(
|
112 |
+
np.where(window_sum > tiny(window_sum))[0]
|
113 |
+
)
|
114 |
+
window_sum = torch.autograd.Variable(
|
115 |
+
torch.from_numpy(window_sum), requires_grad=False
|
116 |
+
)
|
117 |
+
window_sum = window_sum
|
118 |
+
inverse_transform[:, :, approx_nonzero_indices] /= window_sum[
|
119 |
+
approx_nonzero_indices
|
120 |
+
]
|
121 |
+
|
122 |
+
# scale by hop ratio
|
123 |
+
inverse_transform *= float(self.filter_length) / self.hop_length
|
124 |
+
|
125 |
+
inverse_transform = inverse_transform[:, :, int(self.filter_length / 2) :]
|
126 |
+
inverse_transform = inverse_transform[:, :, : -int(self.filter_length / 2) :]
|
127 |
+
|
128 |
+
return inverse_transform
|
129 |
+
|
130 |
+
def forward(self, input_data):
|
131 |
+
self.magnitude, self.phase = self.transform(input_data)
|
132 |
+
reconstruction = self.inverse(self.magnitude, self.phase)
|
133 |
+
return reconstruction
|
134 |
+
|
135 |
+
|
136 |
+
class TacotronSTFT(torch.nn.Module):
|
137 |
+
def __init__(
|
138 |
+
self,
|
139 |
+
filter_length,
|
140 |
+
hop_length,
|
141 |
+
win_length,
|
142 |
+
n_mel_channels,
|
143 |
+
sampling_rate,
|
144 |
+
mel_fmin,
|
145 |
+
mel_fmax,
|
146 |
+
):
|
147 |
+
super(TacotronSTFT, self).__init__()
|
148 |
+
self.n_mel_channels = n_mel_channels
|
149 |
+
self.sampling_rate = sampling_rate
|
150 |
+
self.stft_fn = STFT(filter_length, hop_length, win_length)
|
151 |
+
mel_basis = librosa_mel_fn(
|
152 |
+
sampling_rate, filter_length, n_mel_channels, mel_fmin, mel_fmax
|
153 |
+
)
|
154 |
+
mel_basis = torch.from_numpy(mel_basis).float()
|
155 |
+
self.register_buffer("mel_basis", mel_basis)
|
156 |
+
|
157 |
+
def spectral_normalize(self, magnitudes, normalize_fun):
|
158 |
+
output = dynamic_range_compression(magnitudes, normalize_fun)
|
159 |
+
return output
|
160 |
+
|
161 |
+
def spectral_de_normalize(self, magnitudes):
|
162 |
+
output = dynamic_range_decompression(magnitudes)
|
163 |
+
return output
|
164 |
+
|
165 |
+
def mel_spectrogram(self, y, normalize_fun=torch.log):
|
166 |
+
"""Computes mel-spectrograms from a batch of waves
|
167 |
+
PARAMS
|
168 |
+
------
|
169 |
+
y: Variable(torch.FloatTensor) with shape (B, T) in range [-1, 1]
|
170 |
+
|
171 |
+
RETURNS
|
172 |
+
-------
|
173 |
+
mel_output: torch.FloatTensor of shape (B, n_mel_channels, T)
|
174 |
+
"""
|
175 |
+
assert torch.min(y.data) >= -1, torch.min(y.data)
|
176 |
+
assert torch.max(y.data) <= 1, torch.max(y.data)
|
177 |
+
|
178 |
+
magnitudes, phases = self.stft_fn.transform(y)
|
179 |
+
magnitudes = magnitudes.data
|
180 |
+
mel_output = torch.matmul(self.mel_basis, magnitudes)
|
181 |
+
mel_output = self.spectral_normalize(mel_output, normalize_fun)
|
182 |
+
energy = torch.norm(magnitudes, dim=1)
|
183 |
+
|
184 |
+
log_magnitudes = self.spectral_normalize(magnitudes, normalize_fun)
|
185 |
+
|
186 |
+
return mel_output, log_magnitudes, energy
|
src/audioldm/audio/tools.py
ADDED
@@ -0,0 +1,85 @@
|
|
|
|
|
|
|
|
|
|
|
|
|
|
|
|
|
|
|
|
|
|
|
|
|
|
|
|
|
|
|
|
|
|
|
|
|
|
|
|
|
|
|
|
|
|
|
|
|
|
|
|
|
|
|
|
|
|
|
|
|
|
|
|
|
|
|
|
|
|
|
|
|
|
|
|
|
|
|
|
|
|
|
|
|
|
|
|
|
|
|
|
|
|
|
|
|
|
|
|
|
|
|
|
|
|
|
|
|
|
|
|
|
|
|
|
|
|
|
|
|
|
|
|
|
|
|
|
|
|
|
|
|
|
|
|
|
|
|
|
|
|
|
|
|
|
|
|
|
|
|
|
|
|
|
|
|
|
|
|
|
|
|
|
|
|
|
|
|
|
|
|
|
1 |
+
import torch
|
2 |
+
import numpy as np
|
3 |
+
import torchaudio
|
4 |
+
|
5 |
+
|
6 |
+
def get_mel_from_wav(audio, _stft):
|
7 |
+
audio = torch.clip(torch.FloatTensor(audio).unsqueeze(0), -1, 1)
|
8 |
+
audio = torch.autograd.Variable(audio, requires_grad=False)
|
9 |
+
melspec, log_magnitudes_stft, energy = _stft.mel_spectrogram(audio)
|
10 |
+
melspec = torch.squeeze(melspec, 0).numpy().astype(np.float32)
|
11 |
+
log_magnitudes_stft = (
|
12 |
+
torch.squeeze(log_magnitudes_stft, 0).numpy().astype(np.float32)
|
13 |
+
)
|
14 |
+
energy = torch.squeeze(energy, 0).numpy().astype(np.float32)
|
15 |
+
return melspec, log_magnitudes_stft, energy
|
16 |
+
|
17 |
+
|
18 |
+
def _pad_spec(fbank, target_length=1024):
|
19 |
+
n_frames = fbank.shape[0]
|
20 |
+
p = target_length - n_frames
|
21 |
+
# cut and pad
|
22 |
+
if p > 0:
|
23 |
+
m = torch.nn.ZeroPad2d((0, 0, 0, p))
|
24 |
+
fbank = m(fbank)
|
25 |
+
elif p < 0:
|
26 |
+
fbank = fbank[0:target_length, :]
|
27 |
+
|
28 |
+
if fbank.size(-1) % 2 != 0:
|
29 |
+
fbank = fbank[..., :-1]
|
30 |
+
|
31 |
+
return fbank
|
32 |
+
|
33 |
+
|
34 |
+
def pad_wav(waveform, segment_length):
|
35 |
+
waveform_length = waveform.shape[-1]
|
36 |
+
assert waveform_length > 100, "Waveform is too short, %s" % waveform_length
|
37 |
+
if segment_length is None or waveform_length == segment_length:
|
38 |
+
return waveform
|
39 |
+
elif waveform_length > segment_length:
|
40 |
+
return waveform[:segment_length]
|
41 |
+
elif waveform_length < segment_length:
|
42 |
+
temp_wav = np.zeros((1, segment_length))
|
43 |
+
temp_wav[:, :waveform_length] = waveform
|
44 |
+
return temp_wav
|
45 |
+
|
46 |
+
def normalize_wav(waveform):
|
47 |
+
waveform = waveform - np.mean(waveform)
|
48 |
+
waveform = waveform / (np.max(np.abs(waveform)) + 1e-8)
|
49 |
+
return waveform * 0.5
|
50 |
+
|
51 |
+
|
52 |
+
def read_wav_file(filename, segment_length):
|
53 |
+
# waveform, sr = librosa.load(filename, sr=None, mono=True) # 4 times slower
|
54 |
+
waveform, sr = torchaudio.load(filename) # Faster!!!
|
55 |
+
waveform = torchaudio.functional.resample(waveform, orig_freq=sr, new_freq=16000)
|
56 |
+
waveform = waveform.numpy()[0, ...]
|
57 |
+
waveform = normalize_wav(waveform)
|
58 |
+
waveform = waveform[None, ...]
|
59 |
+
waveform = pad_wav(waveform, segment_length)
|
60 |
+
|
61 |
+
waveform = waveform / np.max(np.abs(waveform))
|
62 |
+
waveform = 0.5 * waveform
|
63 |
+
|
64 |
+
return waveform
|
65 |
+
|
66 |
+
|
67 |
+
def wav_to_fbank(filename, target_length=1024, fn_STFT=None):
|
68 |
+
assert fn_STFT is not None
|
69 |
+
|
70 |
+
# mixup
|
71 |
+
waveform = read_wav_file(filename, target_length * 160) # hop size is 160
|
72 |
+
|
73 |
+
waveform = waveform[0, ...]
|
74 |
+
waveform = torch.FloatTensor(waveform)
|
75 |
+
|
76 |
+
fbank, log_magnitudes_stft, energy = get_mel_from_wav(waveform, fn_STFT)
|
77 |
+
|
78 |
+
fbank = torch.FloatTensor(fbank.T)
|
79 |
+
log_magnitudes_stft = torch.FloatTensor(log_magnitudes_stft.T)
|
80 |
+
|
81 |
+
fbank, log_magnitudes_stft = _pad_spec(fbank, target_length), _pad_spec(
|
82 |
+
log_magnitudes_stft, target_length
|
83 |
+
)
|
84 |
+
|
85 |
+
return fbank, log_magnitudes_stft, waveform
|
src/audioldm/clap/__init__.py
ADDED
File without changes
|
src/audioldm/clap/encoders.py
ADDED
@@ -0,0 +1,170 @@
|
|
|
|
|
|
|
|
|
|
|
|
|
|
|
|
|
|
|
|
|
|
|
|
|
|
|
|
|
|
|
|
|
|
|
|
|
|
|
|
|
|
|
|
|
|
|
|
|
|
|
|
|
|
|
|
|
|
|
|
|
|
|
|
|
|
|
|
|
|
|
|
|
|
|
|
|
|
|
|
|
|
|
|
|
|
|
|
|
|
|
|
|
|
|
|
|
|
|
|
|
|
|
|
|
|
|
|
|
|
|
|
|
|
|
|
|
|
|
|
|
|
|
|
|
|
|
|
|
|
|
|
|
|
|
|
|
|
|
|
|
|
|
|
|
|
|
|
|
|
|
|
|
|
|
|
|
|
|
|
|
|
|
|
|
|
|
|
|
|
|
|
|
|
|
|
|
|
|
|
|
|
|
|
|
|
|
|
|
|
|
|
|
|
|
|
|
|
|
|
|
|
|
|
|
|
|
|
|
|
|
|
|
|
|
|
|
|
|
|
|
|
|
|
|
|
|
|
|
|
|
|
|
|
|
|
|
|
|
|
|
|
|
|
|
|
|
|
|
|
|
|
|
|
|
|
|
|
|
|
|
|
|
|
|
|
|
|
|
|
|
|
|
|
|
|
|
|
|
|
|
|
|
|
|
|
|
|
|
|
|
|
|
|
|
|
|
|
|
|
|
|
|
|
|
|
|
|
|
|
|
|
|
|
|
|
|
|
|
|
|
|
|
|
|
|
|
|
|
|
|
|
|
|
|
|
|
|
|
|
|
|
|
1 |
+
import torch
|
2 |
+
import torch.nn as nn
|
3 |
+
from audioldm.clap.open_clip import create_model
|
4 |
+
from audioldm.clap.training.data import get_audio_features
|
5 |
+
import torchaudio
|
6 |
+
from transformers import RobertaTokenizer
|
7 |
+
import torch.nn.functional as F
|
8 |
+
|
9 |
+
|
10 |
+
class CLAPAudioEmbeddingClassifierFreev2(nn.Module):
|
11 |
+
def __init__(
|
12 |
+
self,
|
13 |
+
pretrained_path="",
|
14 |
+
key="class",
|
15 |
+
sampling_rate=16000,
|
16 |
+
embed_mode="audio",
|
17 |
+
amodel = "HTSAT-tiny",
|
18 |
+
unconditional_prob=0.1,
|
19 |
+
random_mute=False,
|
20 |
+
max_random_mute_portion=0.5,
|
21 |
+
training_mode=True,
|
22 |
+
):
|
23 |
+
super().__init__()
|
24 |
+
|
25 |
+
self.key = key
|
26 |
+
self.device = "cpu"
|
27 |
+
self.precision = "fp32"
|
28 |
+
self.amodel = amodel # or 'PANN-14'
|
29 |
+
self.tmodel = "roberta" # the best text encoder in our training
|
30 |
+
self.enable_fusion = False # False if you do not want to use the fusion model
|
31 |
+
self.fusion_type = "aff_2d"
|
32 |
+
self.pretrained = pretrained_path
|
33 |
+
self.embed_mode = embed_mode
|
34 |
+
self.embed_mode_orig = embed_mode
|
35 |
+
self.sampling_rate = sampling_rate
|
36 |
+
self.unconditional_prob = unconditional_prob
|
37 |
+
self.random_mute = random_mute
|
38 |
+
self.tokenize = RobertaTokenizer.from_pretrained("roberta-base")
|
39 |
+
self.max_random_mute_portion = max_random_mute_portion
|
40 |
+
self.training_mode = training_mode
|
41 |
+
self.model, self.model_cfg = create_model(
|
42 |
+
self.amodel,
|
43 |
+
self.tmodel,
|
44 |
+
self.pretrained,
|
45 |
+
precision=self.precision,
|
46 |
+
device=self.device,
|
47 |
+
enable_fusion=self.enable_fusion,
|
48 |
+
fusion_type=self.fusion_type,
|
49 |
+
)
|
50 |
+
for p in self.model.parameters():
|
51 |
+
p.requires_grad = False
|
52 |
+
|
53 |
+
self.model.eval()
|
54 |
+
|
55 |
+
def get_unconditional_condition(self, batchsize):
|
56 |
+
self.unconditional_token = self.model.get_text_embedding(
|
57 |
+
self.tokenizer(["", ""])
|
58 |
+
)[0:1]
|
59 |
+
return torch.cat([self.unconditional_token.unsqueeze(0)] * batchsize, dim=0)
|
60 |
+
|
61 |
+
def batch_to_list(self, batch):
|
62 |
+
ret = []
|
63 |
+
for i in range(batch.size(0)):
|
64 |
+
ret.append(batch[i])
|
65 |
+
return ret
|
66 |
+
|
67 |
+
def make_decision(self, probability):
|
68 |
+
if float(torch.rand(1)) < probability:
|
69 |
+
return True
|
70 |
+
else:
|
71 |
+
return False
|
72 |
+
|
73 |
+
def random_uniform(self, start, end):
|
74 |
+
val = torch.rand(1).item()
|
75 |
+
return start + (end - start) * val
|
76 |
+
|
77 |
+
def _random_mute(self, waveform):
|
78 |
+
# waveform: [bs, t-steps]
|
79 |
+
t_steps = waveform.size(-1)
|
80 |
+
for i in range(waveform.size(0)):
|
81 |
+
mute_size = int(
|
82 |
+
self.random_uniform(0, end=int(t_steps * self.max_random_mute_portion))
|
83 |
+
)
|
84 |
+
mute_start = int(self.random_uniform(0, t_steps - mute_size))
|
85 |
+
waveform[i, mute_start : mute_start + mute_size] = 0
|
86 |
+
return waveform
|
87 |
+
|
88 |
+
def cos_similarity(self, waveform, text):
|
89 |
+
# waveform: [bs, t_steps]
|
90 |
+
with torch.no_grad():
|
91 |
+
self.embed_mode = "audio"
|
92 |
+
audio_emb = self(waveform.cuda())
|
93 |
+
self.embed_mode = "text"
|
94 |
+
text_emb = self(text)
|
95 |
+
similarity = F.cosine_similarity(audio_emb, text_emb, dim=2), audio_emb, text_emb
|
96 |
+
return similarity.squeeze()
|
97 |
+
|
98 |
+
def forward(self, batch, key=None):
|
99 |
+
# If you want this conditioner to be unconditional, set self.unconditional_prob = 1.0
|
100 |
+
# If you want this conditioner to be fully conditional, set self.unconditional_prob = 0.0
|
101 |
+
if self.model.training == True and not self.training_mode:
|
102 |
+
print(
|
103 |
+
"The pretrained CLAP model should always be in eval mode. Reloading model just in case you change the parameters."
|
104 |
+
)
|
105 |
+
self.model, self.model_cfg = create_model(
|
106 |
+
self.amodel,
|
107 |
+
self.tmodel,
|
108 |
+
self.pretrained,
|
109 |
+
precision=self.precision,
|
110 |
+
device="cuda",
|
111 |
+
enable_fusion=self.enable_fusion,
|
112 |
+
fusion_type=self.fusion_type,
|
113 |
+
)
|
114 |
+
for p in self.model.parameters():
|
115 |
+
p.requires_grad = False
|
116 |
+
self.model.eval()
|
117 |
+
|
118 |
+
# the 'fusion' truncate mode can be changed to 'rand_trunc' if run in unfusion mode
|
119 |
+
if self.embed_mode == "audio":
|
120 |
+
with torch.no_grad():
|
121 |
+
audio_dict_list = []
|
122 |
+
assert (
|
123 |
+
self.sampling_rate == 16000
|
124 |
+
), "We only support 16000 sampling rate"
|
125 |
+
if self.random_mute:
|
126 |
+
batch = self._random_mute(batch)
|
127 |
+
# batch: [bs, 1, t-samples]
|
128 |
+
batch = torchaudio.functional.resample(
|
129 |
+
batch, orig_freq=self.sampling_rate, new_freq=48000
|
130 |
+
)
|
131 |
+
for waveform in self.batch_to_list(batch):
|
132 |
+
audio_dict = {}
|
133 |
+
audio_dict = get_audio_features(
|
134 |
+
audio_dict,
|
135 |
+
waveform,
|
136 |
+
480000,
|
137 |
+
data_truncating="fusion",
|
138 |
+
data_filling="repeatpad",
|
139 |
+
audio_cfg=self.model_cfg["audio_cfg"],
|
140 |
+
)
|
141 |
+
audio_dict_list.append(audio_dict)
|
142 |
+
# [bs, 512]
|
143 |
+
embed = self.model.get_audio_embedding(audio_dict_list)
|
144 |
+
elif self.embed_mode == "text":
|
145 |
+
with torch.no_grad():
|
146 |
+
# the 'fusion' truncate mode can be changed to 'rand_trunc' if run in unfusion mode
|
147 |
+
text_data = self.tokenizer(batch)
|
148 |
+
embed = self.model.get_text_embedding(text_data)
|
149 |
+
|
150 |
+
embed = embed.unsqueeze(1)
|
151 |
+
self.unconditional_token = self.model.get_text_embedding(
|
152 |
+
self.tokenizer(["", ""])
|
153 |
+
)[0:1]
|
154 |
+
|
155 |
+
for i in range(embed.size(0)):
|
156 |
+
if self.make_decision(self.unconditional_prob):
|
157 |
+
embed[i] = self.unconditional_token
|
158 |
+
|
159 |
+
# [bs, 1, 512]
|
160 |
+
return embed.detach()
|
161 |
+
|
162 |
+
def tokenizer(self, text):
|
163 |
+
result = self.tokenize(
|
164 |
+
text,
|
165 |
+
padding="max_length",
|
166 |
+
truncation=True,
|
167 |
+
max_length=512,
|
168 |
+
return_tensors="pt",
|
169 |
+
)
|
170 |
+
return {k: v.squeeze(0) for k, v in result.items()}
|
src/audioldm/clap/open_clip/__init__.py
ADDED
@@ -0,0 +1,25 @@
|
|
|
|
|
|
|
|
|
|
|
|
|
|
|
|
|
|
|
|
|
|
|
|
|
|
|
|
|
|
|
|
|
|
|
|
|
|
|
|
|
|
|
|
|
|
|
|
|
|
|
|
|
1 |
+
from .factory import (
|
2 |
+
list_models,
|
3 |
+
create_model,
|
4 |
+
create_model_and_transforms,
|
5 |
+
add_model_config,
|
6 |
+
)
|
7 |
+
from .loss import ClipLoss, gather_features, LPLoss, lp_gather_features, LPMetrics
|
8 |
+
from .model import (
|
9 |
+
CLAP,
|
10 |
+
CLAPTextCfg,
|
11 |
+
CLAPVisionCfg,
|
12 |
+
CLAPAudioCfp,
|
13 |
+
convert_weights_to_fp16,
|
14 |
+
trace_model,
|
15 |
+
)
|
16 |
+
from .openai import load_openai_model, list_openai_models
|
17 |
+
from .pretrained import (
|
18 |
+
list_pretrained,
|
19 |
+
list_pretrained_tag_models,
|
20 |
+
list_pretrained_model_tags,
|
21 |
+
get_pretrained_url,
|
22 |
+
download_pretrained,
|
23 |
+
)
|
24 |
+
from .tokenizer import SimpleTokenizer, tokenize
|
25 |
+
from .transform import image_transform
|
src/audioldm/clap/open_clip/bert.py
ADDED
@@ -0,0 +1,40 @@
|
|
|
|
|
|
|
|
|
|
|
|
|
|
|
|
|
|
|
|
|
|
|
|
|
|
|
|
|
|
|
|
|
|
|
|
|
|
|
|
|
|
|
|
|
|
|
|
|
|
|
|
|
|
|
|
|
|
|
|
|
|
|
|
|
|
|
|
|
|
|
|
|
|
|
|
|
|
|
|
|
|
|
1 |
+
from transformers import BertTokenizer, BertModel
|
2 |
+
|
3 |
+
tokenizer = BertTokenizer.from_pretrained("bert-base-uncased")
|
4 |
+
model = BertModel.from_pretrained("bert-base-uncased")
|
5 |
+
text = "Replace me by any text you'd like."
|
6 |
+
|
7 |
+
|
8 |
+
def bert_embeddings(text):
|
9 |
+
# text = "Replace me by any text you'd like."
|
10 |
+
encoded_input = tokenizer(text, return_tensors="pt")
|
11 |
+
output = model(**encoded_input)
|
12 |
+
return output
|
13 |
+
|
14 |
+
|
15 |
+
from transformers import RobertaTokenizer, RobertaModel
|
16 |
+
|
17 |
+
tokenizer = RobertaTokenizer.from_pretrained("roberta-base")
|
18 |
+
model = RobertaModel.from_pretrained("roberta-base")
|
19 |
+
text = "Replace me by any text you'd like."
|
20 |
+
|
21 |
+
|
22 |
+
def Roberta_embeddings(text):
|
23 |
+
# text = "Replace me by any text you'd like."
|
24 |
+
encoded_input = tokenizer(text, return_tensors="pt")
|
25 |
+
output = model(**encoded_input)
|
26 |
+
return output
|
27 |
+
|
28 |
+
|
29 |
+
from transformers import BartTokenizer, BartModel
|
30 |
+
|
31 |
+
tokenizer = BartTokenizer.from_pretrained("facebook/bart-base")
|
32 |
+
model = BartModel.from_pretrained("facebook/bart-base")
|
33 |
+
text = "Replace me by any text you'd like."
|
34 |
+
|
35 |
+
|
36 |
+
def bart_embeddings(text):
|
37 |
+
# text = "Replace me by any text you'd like."
|
38 |
+
encoded_input = tokenizer(text, return_tensors="pt")
|
39 |
+
output = model(**encoded_input)
|
40 |
+
return output
|
src/audioldm/clap/open_clip/bpe_simple_vocab_16e6.txt.gz
ADDED
@@ -0,0 +1,3 @@
|
|
|
|
|
|
|
|
|
1 |
+
version https://git-lfs.github.com/spec/v1
|
2 |
+
oid sha256:924691ac288e54409236115652ad4aa250f48203de50a9e4722a6ecd48d6804a
|
3 |
+
size 1356917
|
src/audioldm/clap/open_clip/factory.py
ADDED
@@ -0,0 +1,279 @@
|
|
|
|
|
|
|
|
|
|
|
|
|
|
|
|
|
|
|
|
|
|
|
|
|
|
|
|
|
|
|
|
|
|
|
|
|
|
|
|
|
|
|
|
|
|
|
|
|
|
|
|
|
|
|
|
|
|
|
|
|
|
|
|
|
|
|
|
|
|
|
|
|
|
|
|
|
|
|
|
|
|
|
|
|
|
|
|
|
|
|
|
|
|
|
|
|
|
|
|
|
|
|
|
|
|
|
|
|
|
|
|
|
|
|
|
|
|
|
|
|
|
|
|
|
|
|
|
|
|
|
|
|
|
|
|
|
|
|
|
|
|
|
|
|
|
|
|
|
|
|
|
|
|
|
|
|
|
|
|
|
|
|
|
|
|
|
|
|
|
|
|
|
|
|
|
|
|
|
|
|
|
|
|
|
|
|
|
|
|
|
|
|
|
|
|
|
|
|
|
|
|
|
|
|
|
|
|
|
|
|
|
|
|
|
|
|
|
|
|
|
|
|
|
|
|
|
|
|
|
|
|
|
|
|
|
|
|
|
|
|
|
|
|
|
|
|
|
|
|
|
|
|
|
|
|
|
|
|
|
|
|
|
|
|
|
|
|
|
|
|
|
|
|
|
|
|
|
|
|
|
|
|
|
|
|
|
|
|
|
|
|
|
|
|
|
|
|
|
|
|
|
|
|
|
|
|
|
|
|
|
|
|
|
|
|
|
|
|
|
|
|
|
|
|
|
|
|
|
|
|
|
|
|
|
|
|
|
|
|
|
|
|
|
|
|
|
|
|
|
|
|
|
|
|
|
|
|
|
|
|
|
|
|
|
|
|
|
|
|
|
|
|
|
|
|
|
|
|
|
|
|
|
|
|
|
|
|
|
|
|
|
|
|
|
|
|
|
|
|
|
|
|
|
|
|
|
|
|
|
|
|
|
|
|
|
|
|
|
|
|
|
|
|
|
|
|
|
|
|
|
|
|
|
|
|
|
|
|
|
|
|
|
|
|
|
|
|
|
|
|
|
|
|
|
|
|
|
|
|
|
|
|
|
|
|
|
|
|
|
|
|
|
|
|
|
|
|
|
|
|
|
|
|
|
|
|
|
|
|
|
|
|
|
|
|
|
|
|
|
|
|
|
|
|
|
|
|
|
|
|
|
|
|
|
|
|
|
|
|
|
|
|
|
|
|
|
|
|
|
|
|
|
|
|
|
|
|
|
|
|
|
|
|
|
|
|
|
|
|
|
|
|
|
|
|
|
|
|
|
|
1 |
+
import json
|
2 |
+
import logging
|
3 |
+
import os
|
4 |
+
import pathlib
|
5 |
+
import re
|
6 |
+
from copy import deepcopy
|
7 |
+
from pathlib import Path
|
8 |
+
|
9 |
+
import torch
|
10 |
+
|
11 |
+
from .model import CLAP, convert_weights_to_fp16
|
12 |
+
from .openai import load_openai_model
|
13 |
+
from .pretrained import get_pretrained_url, download_pretrained
|
14 |
+
from .transform import image_transform
|
15 |
+
|
16 |
+
_MODEL_CONFIG_PATHS = [Path(__file__).parent / f"model_configs/"]
|
17 |
+
_MODEL_CONFIGS = {} # directory (model_name: config) of model architecture configs
|
18 |
+
CACHE_DIR = os.getenv("AUDIOLDM_CACHE_DIR", "~/.cache/audioldm")
|
19 |
+
|
20 |
+
|
21 |
+
|
22 |
+
def _natural_key(string_):
|
23 |
+
return [int(s) if s.isdigit() else s for s in re.split(r"(\d+)", string_.lower())]
|
24 |
+
|
25 |
+
|
26 |
+
def _rescan_model_configs():
|
27 |
+
global _MODEL_CONFIGS
|
28 |
+
|
29 |
+
config_ext = (".json",)
|
30 |
+
config_files = []
|
31 |
+
for config_path in _MODEL_CONFIG_PATHS:
|
32 |
+
if config_path.is_file() and config_path.suffix in config_ext:
|
33 |
+
config_files.append(config_path)
|
34 |
+
elif config_path.is_dir():
|
35 |
+
for ext in config_ext:
|
36 |
+
config_files.extend(config_path.glob(f"*{ext}"))
|
37 |
+
|
38 |
+
for cf in config_files:
|
39 |
+
if os.path.basename(cf)[0] == ".":
|
40 |
+
continue # Ignore hidden files
|
41 |
+
|
42 |
+
with open(cf, "r") as f:
|
43 |
+
model_cfg = json.load(f)
|
44 |
+
if all(a in model_cfg for a in ("embed_dim", "audio_cfg", "text_cfg")):
|
45 |
+
_MODEL_CONFIGS[cf.stem] = model_cfg
|
46 |
+
|
47 |
+
_MODEL_CONFIGS = {
|
48 |
+
k: v
|
49 |
+
for k, v in sorted(_MODEL_CONFIGS.items(), key=lambda x: _natural_key(x[0]))
|
50 |
+
}
|
51 |
+
|
52 |
+
|
53 |
+
_rescan_model_configs() # initial populate of model config registry
|
54 |
+
|
55 |
+
|
56 |
+
def load_state_dict(checkpoint_path: str, map_location="cpu", skip_params=True):
|
57 |
+
checkpoint = torch.load(checkpoint_path, map_location=map_location)
|
58 |
+
if isinstance(checkpoint, dict) and "state_dict" in checkpoint:
|
59 |
+
state_dict = checkpoint["state_dict"]
|
60 |
+
else:
|
61 |
+
state_dict = checkpoint
|
62 |
+
if skip_params:
|
63 |
+
if next(iter(state_dict.items()))[0].startswith("module"):
|
64 |
+
state_dict = {k[7:]: v for k, v in state_dict.items()}
|
65 |
+
# for k in state_dict:
|
66 |
+
# if k.startswith('transformer'):
|
67 |
+
# v = state_dict.pop(k)
|
68 |
+
# state_dict['text_branch.' + k[12:]] = v
|
69 |
+
return state_dict
|
70 |
+
|
71 |
+
|
72 |
+
def create_model(
|
73 |
+
amodel_name: str,
|
74 |
+
tmodel_name: str,
|
75 |
+
pretrained: str = "",
|
76 |
+
precision: str = "fp32",
|
77 |
+
device: torch.device = torch.device("cpu"),
|
78 |
+
jit: bool = False,
|
79 |
+
force_quick_gelu: bool = False,
|
80 |
+
openai_model_cache_dir: str = os.path.expanduser(f"{CACHE_DIR}/clip"),
|
81 |
+
skip_params=True,
|
82 |
+
pretrained_audio: str = "",
|
83 |
+
pretrained_text: str = "",
|
84 |
+
enable_fusion: bool = False,
|
85 |
+
fusion_type: str = "None"
|
86 |
+
# pretrained_image: bool = False,
|
87 |
+
):
|
88 |
+
amodel_name = amodel_name.replace(
|
89 |
+
"/", "-"
|
90 |
+
) # for callers using old naming with / in ViT names
|
91 |
+
pretrained_orig = pretrained
|
92 |
+
pretrained = pretrained.lower()
|
93 |
+
if pretrained == "openai":
|
94 |
+
if amodel_name in _MODEL_CONFIGS:
|
95 |
+
logging.info(f"Loading {amodel_name} model config.")
|
96 |
+
model_cfg = deepcopy(_MODEL_CONFIGS[amodel_name])
|
97 |
+
else:
|
98 |
+
logging.error(
|
99 |
+
f"Model config for {amodel_name} not found; available models {list_models()}."
|
100 |
+
)
|
101 |
+
raise RuntimeError(f"Model config for {amodel_name} not found.")
|
102 |
+
|
103 |
+
logging.info(f"Loading pretrained ViT-B-16 text encoder from OpenAI.")
|
104 |
+
# Hard Code in model name
|
105 |
+
model_cfg["text_cfg"]["model_type"] = tmodel_name
|
106 |
+
model = load_openai_model(
|
107 |
+
"ViT-B-16",
|
108 |
+
model_cfg,
|
109 |
+
device=device,
|
110 |
+
jit=jit,
|
111 |
+
cache_dir=openai_model_cache_dir,
|
112 |
+
enable_fusion=enable_fusion,
|
113 |
+
fusion_type=fusion_type,
|
114 |
+
)
|
115 |
+
# See https://discuss.pytorch.org/t/valueerror-attemting-to-unscale-fp16-gradients/81372
|
116 |
+
if precision == "amp" or precision == "fp32":
|
117 |
+
model = model.float()
|
118 |
+
else:
|
119 |
+
if amodel_name in _MODEL_CONFIGS:
|
120 |
+
logging.info(f"Loading {amodel_name} model config.")
|
121 |
+
model_cfg = deepcopy(_MODEL_CONFIGS[amodel_name])
|
122 |
+
else:
|
123 |
+
logging.error(
|
124 |
+
f"Model config for {amodel_name} not found; available models {list_models()}."
|
125 |
+
)
|
126 |
+
raise RuntimeError(f"Model config for {amodel_name} not found.")
|
127 |
+
|
128 |
+
if force_quick_gelu:
|
129 |
+
# override for use of QuickGELU on non-OpenAI transformer models
|
130 |
+
model_cfg["quick_gelu"] = True
|
131 |
+
|
132 |
+
# if pretrained_image:
|
133 |
+
# if 'timm_amodel_name' in model_cfg.get('vision_cfg', {}):
|
134 |
+
# # pretrained weight loading for timm models set via vision_cfg
|
135 |
+
# model_cfg['vision_cfg']['timm_model_pretrained'] = True
|
136 |
+
# else:
|
137 |
+
# assert False, 'pretrained image towers currently only supported for timm models'
|
138 |
+
model_cfg["text_cfg"]["model_type"] = tmodel_name
|
139 |
+
model_cfg["enable_fusion"] = enable_fusion
|
140 |
+
model_cfg["fusion_type"] = fusion_type
|
141 |
+
model = CLAP(**model_cfg)
|
142 |
+
|
143 |
+
if pretrained:
|
144 |
+
checkpoint_path = ""
|
145 |
+
url = get_pretrained_url(amodel_name, pretrained)
|
146 |
+
if url:
|
147 |
+
checkpoint_path = download_pretrained(url, root=openai_model_cache_dir)
|
148 |
+
elif os.path.exists(pretrained_orig):
|
149 |
+
checkpoint_path = pretrained_orig
|
150 |
+
if checkpoint_path:
|
151 |
+
logging.info(
|
152 |
+
f"Loading pretrained {amodel_name}-{tmodel_name} weights ({pretrained})."
|
153 |
+
)
|
154 |
+
ckpt = load_state_dict(checkpoint_path, skip_params=True)
|
155 |
+
model.load_state_dict(ckpt)
|
156 |
+
param_names = [n for n, p in model.named_parameters()]
|
157 |
+
# for n in param_names:
|
158 |
+
# print(n, "\t", "Loaded" if n in ckpt else "Unloaded")
|
159 |
+
else:
|
160 |
+
logging.warning(
|
161 |
+
f"Pretrained weights ({pretrained}) not found for model {amodel_name}."
|
162 |
+
)
|
163 |
+
raise RuntimeError(
|
164 |
+
f"Pretrained weights ({pretrained}) not found for model {amodel_name}."
|
165 |
+
)
|
166 |
+
|
167 |
+
if pretrained_audio:
|
168 |
+
if amodel_name.startswith("PANN"):
|
169 |
+
if "Cnn14_mAP" in pretrained_audio: # official checkpoint
|
170 |
+
audio_ckpt = torch.load(pretrained_audio, map_location="cpu")
|
171 |
+
audio_ckpt = audio_ckpt["model"]
|
172 |
+
keys = list(audio_ckpt.keys())
|
173 |
+
for key in keys:
|
174 |
+
if (
|
175 |
+
"spectrogram_extractor" not in key
|
176 |
+
and "logmel_extractor" not in key
|
177 |
+
):
|
178 |
+
v = audio_ckpt.pop(key)
|
179 |
+
audio_ckpt["audio_branch." + key] = v
|
180 |
+
elif os.path.basename(pretrained_audio).startswith(
|
181 |
+
"PANN"
|
182 |
+
): # checkpoint trained via HTSAT codebase
|
183 |
+
audio_ckpt = torch.load(pretrained_audio, map_location="cpu")
|
184 |
+
audio_ckpt = audio_ckpt["state_dict"]
|
185 |
+
keys = list(audio_ckpt.keys())
|
186 |
+
for key in keys:
|
187 |
+
if key.startswith("sed_model"):
|
188 |
+
v = audio_ckpt.pop(key)
|
189 |
+
audio_ckpt["audio_branch." + key[10:]] = v
|
190 |
+
elif os.path.basename(pretrained_audio).startswith(
|
191 |
+
"finetuned"
|
192 |
+
): # checkpoint trained via linear probe codebase
|
193 |
+
audio_ckpt = torch.load(pretrained_audio, map_location="cpu")
|
194 |
+
else:
|
195 |
+
raise ValueError("Unknown audio checkpoint")
|
196 |
+
elif amodel_name.startswith("HTSAT"):
|
197 |
+
if "HTSAT_AudioSet_Saved" in pretrained_audio: # official checkpoint
|
198 |
+
audio_ckpt = torch.load(pretrained_audio, map_location="cpu")
|
199 |
+
audio_ckpt = audio_ckpt["state_dict"]
|
200 |
+
keys = list(audio_ckpt.keys())
|
201 |
+
for key in keys:
|
202 |
+
if key.startswith("sed_model") and (
|
203 |
+
"spectrogram_extractor" not in key
|
204 |
+
and "logmel_extractor" not in key
|
205 |
+
):
|
206 |
+
v = audio_ckpt.pop(key)
|
207 |
+
audio_ckpt["audio_branch." + key[10:]] = v
|
208 |
+
elif os.path.basename(pretrained_audio).startswith(
|
209 |
+
"HTSAT"
|
210 |
+
): # checkpoint trained via HTSAT codebase
|
211 |
+
audio_ckpt = torch.load(pretrained_audio, map_location="cpu")
|
212 |
+
audio_ckpt = audio_ckpt["state_dict"]
|
213 |
+
keys = list(audio_ckpt.keys())
|
214 |
+
for key in keys:
|
215 |
+
if key.startswith("sed_model"):
|
216 |
+
v = audio_ckpt.pop(key)
|
217 |
+
audio_ckpt["audio_branch." + key[10:]] = v
|
218 |
+
elif os.path.basename(pretrained_audio).startswith(
|
219 |
+
"finetuned"
|
220 |
+
): # checkpoint trained via linear probe codebase
|
221 |
+
audio_ckpt = torch.load(pretrained_audio, map_location="cpu")
|
222 |
+
else:
|
223 |
+
raise ValueError("Unknown audio checkpoint")
|
224 |
+
else:
|
225 |
+
raise f"this audio encoder pretrained checkpoint is not support"
|
226 |
+
|
227 |
+
model.load_state_dict(audio_ckpt, strict=False)
|
228 |
+
logging.info(
|
229 |
+
f"Loading pretrained {amodel_name} weights ({pretrained_audio})."
|
230 |
+
)
|
231 |
+
param_names = [n for n, p in model.named_parameters()]
|
232 |
+
for n in param_names:
|
233 |
+
print(n, "\t", "Loaded" if n in audio_ckpt else "Unloaded")
|
234 |
+
|
235 |
+
model.to(device=device)
|
236 |
+
if precision == "fp16":
|
237 |
+
assert device.type != "cpu"
|
238 |
+
convert_weights_to_fp16(model)
|
239 |
+
|
240 |
+
if jit:
|
241 |
+
model = torch.jit.script(model)
|
242 |
+
|
243 |
+
return model, model_cfg
|
244 |
+
|
245 |
+
|
246 |
+
def create_model_and_transforms(
|
247 |
+
model_name: str,
|
248 |
+
pretrained: str = "",
|
249 |
+
precision: str = "fp32",
|
250 |
+
device: torch.device = torch.device("cpu"),
|
251 |
+
jit: bool = False,
|
252 |
+
force_quick_gelu: bool = False,
|
253 |
+
# pretrained_image: bool = False,
|
254 |
+
):
|
255 |
+
model = create_model(
|
256 |
+
model_name,
|
257 |
+
pretrained,
|
258 |
+
precision,
|
259 |
+
device,
|
260 |
+
jit,
|
261 |
+
force_quick_gelu=force_quick_gelu,
|
262 |
+
# pretrained_image=pretrained_image
|
263 |
+
)
|
264 |
+
preprocess_train = image_transform(model.visual.image_size, is_train=True)
|
265 |
+
preprocess_val = image_transform(model.visual.image_size, is_train=False)
|
266 |
+
return model, preprocess_train, preprocess_val
|
267 |
+
|
268 |
+
|
269 |
+
def list_models():
|
270 |
+
"""enumerate available model architectures based on config files"""
|
271 |
+
return list(_MODEL_CONFIGS.keys())
|
272 |
+
|
273 |
+
|
274 |
+
def add_model_config(path):
|
275 |
+
"""add model config path or file and update registry"""
|
276 |
+
if not isinstance(path, Path):
|
277 |
+
path = Path(path)
|
278 |
+
_MODEL_CONFIG_PATHS.append(path)
|
279 |
+
_rescan_model_configs()
|
src/audioldm/clap/open_clip/feature_fusion.py
ADDED
@@ -0,0 +1,192 @@
|
|
|
|
|
|
|
|
|
|
|
|
|
|
|
|
|
|
|
|
|
|
|
|
|
|
|
|
|
|
|
|
|
|
|
|
|
|
|
|
|
|
|
|
|
|
|
|
|
|
|
|
|
|
|
|
|
|
|
|
|
|
|
|
|
|
|
|
|
|
|
|
|
|
|
|
|
|
|
|
|
|
|
|
|
|
|
|
|
|
|
|
|
|
|
|
|
|
|
|
|
|
|
|
|
|
|
|
|
|
|
|
|
|
|
|
|
|
|
|
|
|
|
|
|
|
|
|
|
|
|
|
|
|
|
|
|
|
|
|
|
|
|
|
|
|
|
|
|
|
|
|
|
|
|
|
|
|
|
|
|
|
|
|
|
|
|
|
|
|
|
|
|
|
|
|
|
|
|
|
|
|
|
|
|
|
|
|
|
|
|
|
|
|
|
|
|
|
|
|
|
|
|
|
|
|
|
|
|
|
|
|
|
|
|
|
|
|
|
|
|
|
|
|
|
|
|
|
|
|
|
|
|
|
|
|
|
|
|
|
|
|
|
|
|
|
|
|
|
|
|
|
|
|
|
|
|
|
|
|
|
|
|
|
|
|
|
|
|
|
|
|
|
|
|
|
|
|
|
|
|
|
|
|
|
|
|
|
|
|
|
|
|
|
|
|
|
|
|
|
|
|
|
|
|
|
|
|
|
|
|
|
|
|
|
|
|
|
|
|
|
|
|
|
|
|
|
|
|
|
|
|
|
|
|
|
|
|
|
|
|
|
|
|
|
|
|
|
|
|
|
|
|
|
|
|
|
|
|
|
|
|
|
|
|
|
|
|
|
|
|
|
|
|
|
|
|
|
|
|
|
|
|
|
|
|
|
1 |
+
"""
|
2 |
+
Feature Fusion for Varible-Length Data Processing
|
3 |
+
AFF/iAFF is referred and modified from https://github.com/YimianDai/open-aff/blob/master/aff_pytorch/aff_net/fusion.py
|
4 |
+
According to the paper: Yimian Dai et al, Attentional Feature Fusion, IEEE Winter Conference on Applications of Computer Vision, WACV 2021
|
5 |
+
"""
|
6 |
+
|
7 |
+
import torch
|
8 |
+
import torch.nn as nn
|
9 |
+
|
10 |
+
|
11 |
+
class DAF(nn.Module):
|
12 |
+
"""
|
13 |
+
直接相加 DirectAddFuse
|
14 |
+
"""
|
15 |
+
|
16 |
+
def __init__(self):
|
17 |
+
super(DAF, self).__init__()
|
18 |
+
|
19 |
+
def forward(self, x, residual):
|
20 |
+
return x + residual
|
21 |
+
|
22 |
+
|
23 |
+
class iAFF(nn.Module):
|
24 |
+
"""
|
25 |
+
多特征融合 iAFF
|
26 |
+
"""
|
27 |
+
|
28 |
+
def __init__(self, channels=64, r=4, type="2D"):
|
29 |
+
super(iAFF, self).__init__()
|
30 |
+
inter_channels = int(channels // r)
|
31 |
+
|
32 |
+
if type == "1D":
|
33 |
+
# 本地注意力
|
34 |
+
self.local_att = nn.Sequential(
|
35 |
+
nn.Conv1d(channels, inter_channels, kernel_size=1, stride=1, padding=0),
|
36 |
+
nn.BatchNorm1d(inter_channels),
|
37 |
+
nn.ReLU(inplace=True),
|
38 |
+
nn.Conv1d(inter_channels, channels, kernel_size=1, stride=1, padding=0),
|
39 |
+
nn.BatchNorm1d(channels),
|
40 |
+
)
|
41 |
+
|
42 |
+
# 全局注意力
|
43 |
+
self.global_att = nn.Sequential(
|
44 |
+
nn.AdaptiveAvgPool1d(1),
|
45 |
+
nn.Conv1d(channels, inter_channels, kernel_size=1, stride=1, padding=0),
|
46 |
+
nn.BatchNorm1d(inter_channels),
|
47 |
+
nn.ReLU(inplace=True),
|
48 |
+
nn.Conv1d(inter_channels, channels, kernel_size=1, stride=1, padding=0),
|
49 |
+
nn.BatchNorm1d(channels),
|
50 |
+
)
|
51 |
+
|
52 |
+
# 第二次本地注意力
|
53 |
+
self.local_att2 = nn.Sequential(
|
54 |
+
nn.Conv1d(channels, inter_channels, kernel_size=1, stride=1, padding=0),
|
55 |
+
nn.BatchNorm1d(inter_channels),
|
56 |
+
nn.ReLU(inplace=True),
|
57 |
+
nn.Conv1d(inter_channels, channels, kernel_size=1, stride=1, padding=0),
|
58 |
+
nn.BatchNorm1d(channels),
|
59 |
+
)
|
60 |
+
# 第二次全局注意力
|
61 |
+
self.global_att2 = nn.Sequential(
|
62 |
+
nn.AdaptiveAvgPool1d(1),
|
63 |
+
nn.Conv1d(channels, inter_channels, kernel_size=1, stride=1, padding=0),
|
64 |
+
nn.BatchNorm1d(inter_channels),
|
65 |
+
nn.ReLU(inplace=True),
|
66 |
+
nn.Conv1d(inter_channels, channels, kernel_size=1, stride=1, padding=0),
|
67 |
+
nn.BatchNorm1d(channels),
|
68 |
+
)
|
69 |
+
elif type == "2D":
|
70 |
+
# 本地注意力
|
71 |
+
self.local_att = nn.Sequential(
|
72 |
+
nn.Conv2d(channels, inter_channels, kernel_size=1, stride=1, padding=0),
|
73 |
+
nn.BatchNorm2d(inter_channels),
|
74 |
+
nn.ReLU(inplace=True),
|
75 |
+
nn.Conv2d(inter_channels, channels, kernel_size=1, stride=1, padding=0),
|
76 |
+
nn.BatchNorm2d(channels),
|
77 |
+
)
|
78 |
+
|
79 |
+
# 全局注意力
|
80 |
+
self.global_att = nn.Sequential(
|
81 |
+
nn.AdaptiveAvgPool2d(1),
|
82 |
+
nn.Conv2d(channels, inter_channels, kernel_size=1, stride=1, padding=0),
|
83 |
+
nn.BatchNorm2d(inter_channels),
|
84 |
+
nn.ReLU(inplace=True),
|
85 |
+
nn.Conv2d(inter_channels, channels, kernel_size=1, stride=1, padding=0),
|
86 |
+
nn.BatchNorm2d(channels),
|
87 |
+
)
|
88 |
+
|
89 |
+
# 第二次本地注意力
|
90 |
+
self.local_att2 = nn.Sequential(
|
91 |
+
nn.Conv2d(channels, inter_channels, kernel_size=1, stride=1, padding=0),
|
92 |
+
nn.BatchNorm2d(inter_channels),
|
93 |
+
nn.ReLU(inplace=True),
|
94 |
+
nn.Conv2d(inter_channels, channels, kernel_size=1, stride=1, padding=0),
|
95 |
+
nn.BatchNorm2d(channels),
|
96 |
+
)
|
97 |
+
# 第二次全局注意力
|
98 |
+
self.global_att2 = nn.Sequential(
|
99 |
+
nn.AdaptiveAvgPool2d(1),
|
100 |
+
nn.Conv2d(channels, inter_channels, kernel_size=1, stride=1, padding=0),
|
101 |
+
nn.BatchNorm2d(inter_channels),
|
102 |
+
nn.ReLU(inplace=True),
|
103 |
+
nn.Conv2d(inter_channels, channels, kernel_size=1, stride=1, padding=0),
|
104 |
+
nn.BatchNorm2d(channels),
|
105 |
+
)
|
106 |
+
else:
|
107 |
+
raise f"the type is not supported"
|
108 |
+
|
109 |
+
self.sigmoid = nn.Sigmoid()
|
110 |
+
|
111 |
+
def forward(self, x, residual):
|
112 |
+
flag = False
|
113 |
+
xa = x + residual
|
114 |
+
if xa.size(0) == 1:
|
115 |
+
xa = torch.cat([xa, xa], dim=0)
|
116 |
+
flag = True
|
117 |
+
xl = self.local_att(xa)
|
118 |
+
xg = self.global_att(xa)
|
119 |
+
xlg = xl + xg
|
120 |
+
wei = self.sigmoid(xlg)
|
121 |
+
xi = x * wei + residual * (1 - wei)
|
122 |
+
|
123 |
+
xl2 = self.local_att2(xi)
|
124 |
+
xg2 = self.global_att(xi)
|
125 |
+
xlg2 = xl2 + xg2
|
126 |
+
wei2 = self.sigmoid(xlg2)
|
127 |
+
xo = x * wei2 + residual * (1 - wei2)
|
128 |
+
if flag:
|
129 |
+
xo = xo[0].unsqueeze(0)
|
130 |
+
return xo
|
131 |
+
|
132 |
+
|
133 |
+
class AFF(nn.Module):
|
134 |
+
"""
|
135 |
+
多特征融合 AFF
|
136 |
+
"""
|
137 |
+
|
138 |
+
def __init__(self, channels=64, r=4, type="2D"):
|
139 |
+
super(AFF, self).__init__()
|
140 |
+
inter_channels = int(channels // r)
|
141 |
+
|
142 |
+
if type == "1D":
|
143 |
+
self.local_att = nn.Sequential(
|
144 |
+
nn.Conv1d(channels, inter_channels, kernel_size=1, stride=1, padding=0),
|
145 |
+
nn.BatchNorm1d(inter_channels),
|
146 |
+
nn.ReLU(inplace=True),
|
147 |
+
nn.Conv1d(inter_channels, channels, kernel_size=1, stride=1, padding=0),
|
148 |
+
nn.BatchNorm1d(channels),
|
149 |
+
)
|
150 |
+
self.global_att = nn.Sequential(
|
151 |
+
nn.AdaptiveAvgPool1d(1),
|
152 |
+
nn.Conv1d(channels, inter_channels, kernel_size=1, stride=1, padding=0),
|
153 |
+
nn.BatchNorm1d(inter_channels),
|
154 |
+
nn.ReLU(inplace=True),
|
155 |
+
nn.Conv1d(inter_channels, channels, kernel_size=1, stride=1, padding=0),
|
156 |
+
nn.BatchNorm1d(channels),
|
157 |
+
)
|
158 |
+
elif type == "2D":
|
159 |
+
self.local_att = nn.Sequential(
|
160 |
+
nn.Conv2d(channels, inter_channels, kernel_size=1, stride=1, padding=0),
|
161 |
+
nn.BatchNorm2d(inter_channels),
|
162 |
+
nn.ReLU(inplace=True),
|
163 |
+
nn.Conv2d(inter_channels, channels, kernel_size=1, stride=1, padding=0),
|
164 |
+
nn.BatchNorm2d(channels),
|
165 |
+
)
|
166 |
+
self.global_att = nn.Sequential(
|
167 |
+
nn.AdaptiveAvgPool2d(1),
|
168 |
+
nn.Conv2d(channels, inter_channels, kernel_size=1, stride=1, padding=0),
|
169 |
+
nn.BatchNorm2d(inter_channels),
|
170 |
+
nn.ReLU(inplace=True),
|
171 |
+
nn.Conv2d(inter_channels, channels, kernel_size=1, stride=1, padding=0),
|
172 |
+
nn.BatchNorm2d(channels),
|
173 |
+
)
|
174 |
+
else:
|
175 |
+
raise f"the type is not supported."
|
176 |
+
|
177 |
+
self.sigmoid = nn.Sigmoid()
|
178 |
+
|
179 |
+
def forward(self, x, residual):
|
180 |
+
flag = False
|
181 |
+
xa = x + residual
|
182 |
+
if xa.size(0) == 1:
|
183 |
+
xa = torch.cat([xa, xa], dim=0)
|
184 |
+
flag = True
|
185 |
+
xl = self.local_att(xa)
|
186 |
+
xg = self.global_att(xa)
|
187 |
+
xlg = xl + xg
|
188 |
+
wei = self.sigmoid(xlg)
|
189 |
+
xo = 2 * x * wei + 2 * residual * (1 - wei)
|
190 |
+
if flag:
|
191 |
+
xo = xo[0].unsqueeze(0)
|
192 |
+
return xo
|
src/audioldm/clap/open_clip/htsat.py
ADDED
@@ -0,0 +1,1308 @@
|
|
|
|
|
|
|
|
|
|
|
|
|
|
|
|
|
|
|
|
|
|
|
|
|
|
|
|
|
|
|
|
|
|
|
|
|
|
|
|
|
|
|
|
|
|
|
|
|
|
|
|
|
|
|
|
|
|
|
|
|
|
|
|
|
|
|
|
|
|
|
|
|
|
|
|
|
|
|
|
|
|
|
|
|
|
|
|
|
|
|
|
|
|
|
|
|
|
|
|
|
|
|
|
|
|
|
|
|
|
|
|
|
|
|
|
|
|
|
|
|
|
|
|
|
|
|
|
|
|
|
|
|
|
|
|
|
|
|
|
|
|
|
|
|
|
|
|
|
|
|
|
|
|
|
|
|
|
|
|
|
|
|
|
|
|
|
|
|
|
|
|
|
|
|
|
|
|
|
|
|
|
|
|
|
|
|
|
|
|
|
|
|
|
|
|
|
|
|
|
|
|
|
|
|
|
|
|
|
|
|
|
|
|
|
|
|
|
|
|
|
|
|
|
|
|
|
|
|
|
|
|
|
|
|
|
|
|
|
|
|
|
|
|
|
|
|
|
|
|
|
|
|
|
|
|
|
|
|
|
|
|
|
|
|
|
|
|
|
|
|
|
|
|
|
|
|
|
|
|
|
|
|
|
|
|
|
|
|
|
|
|
|
|
|
|
|
|
|
|
|
|
|
|
|
|
|
|
|
|
|
|
|
|
|
|
|
|
|
|
|
|
|
|
|
|
|
|
|
|
|
|
|
|
|
|
|
|
|
|
|
|
|
|
|
|
|
|
|
|
|
|
|
|
|
|
|
|
|
|
|
|
|
|
|
|
|
|
|
|
|
|
|
|
|
|
|
|
|
|
|
|
|
|
|
|
|
|
|
|
|
|
|
|
|
|
|
|
|
|
|
|
|
|
|
|
|
|
|
|
|
|
|
|
|
|
|
|
|
|
|
|
|
|
|
|
|
|
|
|
|
|
|
|
|
|
|
|
|
|
|
|
|
|
|
|
|
|
|
|
|
|
|
|
|
|
|
|
|
|
|
|
|
|
|
|
|
|
|
|
|
|
|
|
|
|
|
|
|
|
|
|
|
|
|
|
|
|
|
|
|
|
|
|
|
|
|
|
|
|
|
|
|
|
|
|
|
|
|
|
|
|
|
|
|
|
|
|
|
|
|
|
|
|
|
|
|
|
|
|
|
|
|
|
|
|
|
|
|
|
|
|
|
|
|
|
|
|
|
|
|
|
|
|
|
|
|
|
|
|
|
|
|
|
|
|
|
|
|
|
|
|
|
|
|
|
|
|
|
|
|
|
|
|
|
|
|
|
|
|
|
|
|
|
|
|
|
|
|
|
|
|
|
|
|
|
|
|
|
|
|
|
|
|
|
|
|
|
|
|
|
|
|
|
|
|
|
|
|
|
|
|
|
|
|
|
|
|
|
|
|
|
|
|
|
|
|
|
|
|
|
|
|
|
|
|
|
|
|
|
|
|
|
|
|
|
|
|
|
|
|
|
|
|
|
|
|
|
|
|
|
|
|
|
|
|
|
|
|
|
|
|
|
|
|
|
|
|
|
|
|
|
|
|
|
|
|
|
|
|
|
|
|
|
|
|
|
|
|
|
|
|
|
|
|
|
|
|
|
|
|
|
|
|
|
|
|
|
|
|
|
|
|
|
|
|
|
|
|
|
|
|
|
|
|
|
|
|
|
|
|
|
|
|
|
|
|
|
|
|
|
|
|
|
|
|
|
|
|
|
|
|
|
|
|
|
|
|
|
|
|
|
|
|
|
|
|
|
|
|
|
|
|
|
|
|
|
|
|
|
|
|
|
|
|
|
|
|
|
|
|
|
|
|
|
|
|
|
|
|
|
|
|
|
|
|
|
|
|
|
|
|
|
|
|
|
|
|
|
|
|
|
|
|
|
|
|
|
|
|
|
|
|
|
|
|
|
|
|
|
|
|
|
|
|
|
|
|
|
|
|
|
|
|
|
|
|
|
|
|
|
|
|
|
|
|
|
|
|
|
|
|
|
|
|
|
|
|
|
|
|
|
|
|
|
|
|
|
|
|
|
|
|
|
|
|
|
|
|
|
|
|
|
|
|
|
|
|
|
|
|
|
|
|
|
|
|
|
|
|
|
|
|
|
|
|
|
|
|
|
|
|
|
|
|
|
|
|
|
|
|
|
|
|
|
|
|
|
|
|
|
|
|
|
|
|
|
|
|
|
|
|
|
|
|
|
|
|
|
|
|
|
|
|
|
|
|
|
|
|
|
|
|
|
|
|
|
|
|
|
|
|
|
|
|
|
|
|
|
|
|
|
|
|
|
|
|
|
|
|
|
|
|
|
|
|
|
|
|
|
|
|
|
|
|
|
|
|
|
|
|
|
|
|
|
|
|
|
|
|
|
|
|
|
|
|
|
|
|
|
|
|
|
|
|
|
|
|
|
|
|
|
|
|
|
|
|
|
|
|
|
|
|
|
|
|
|
|
|
|
|
|
|
|
|
|
|
|
|
|
|
|
|
|
|
|
|
|
|
|
|
|
|
|
|
|
|
|
|
|
|
|
|
|
|
|
|
|
|
|
|
|
|
|
|
|
|
|
|
|
|
|
|
|
|
|
|
|
|
|
|
|
|
|
|
|
|
|
|
|
|
|
|
|
|
|
|
|
|
|
|
|
|
|
|
|
|
|
|
|
|
|
|
|
|
|
|
|
|
|
|
|
|
|
|
|
|
|
|
|
|
|
|
|
|
|
|
|
|
|
|
|
|
|
|
|
|
|
|
|
|
|
|
|
|
|
|
|
|
|
|
|
|
|
|
|
|
|
|
|
|
|
|
|
|
|
|
|
|
|
|
|
|
|
|
|
|
|
|
|
|
|
|
|
|
|
|
|
|
|
|
|
|
|
|
|
|
|
|
|
|
|
|
|
|
|
|
|
|
|
|
|
|
|
|
|
|
|
|
|
|
|
|
|
|
|
|
|
|
|
|
|
|
|
|
|
|
|
|
|
|
|
|
|
|
|
|
|
|
|
|
|
|
|
|
|
|
|
|
|
|
|
|
|
|
|
|
|
|
|
|
|
|
|
|
|
|
|
|
|
|
|
|
|
|
|
|
|
|
|
|
|
|
|
|
|
|
|
|
|
|
|
|
|
|
|
|
|
|
|
|
|
|
|
|
|
|
|
|
|
|
|
|
|
|
|
|
|
|
|
|
|
|
|
|
|
|
|
|
|
|
|
|
|
|
|
|
|
|
|
|
|
|
|
|
|
|
|
|
|
|
|
|
|
|
|
|
|
|
|
|
|
|
|
|
|
|
|
|
|
|
|
|
|
|
|
|
|
|
|
|
|
|
|
|
|
|
|
|
|
|
|
|
|
|
|
|
|
|
|
|
|
|
|
|
|
|
|
|
|
|
|
|
|
|
|
|
|
|
|
|
|
|
|
|
|
|
|
|
|
|
|
|
|
|
|
|
|
|
|
|
|
|
|
|
|
|
|
|
|
|
|
|
|
|
|
|
|
|
|
|
|
|
|
|
|
|
|
|
|
|
|
|
|
|
|
|
|
|
|
|
|
|
|
|
|
|
|
|
|
|
|
|
|
|
|
|
|
|
|
|
|
|
|
|
|
|
|
|
|
|
|
|
|
|
|
|
|
|
|
|
|
|
|
|
|
|
|
|
|
|
|
|
|
|
|
|
|
|
|
|
|
|
|
|
|
|
|
|
|
|
|
|
|
|
|
|
|
|
|
|
|
|
|
|
|
|
|
|
|
|
|
|
|
|
|
|
|
|
|
|
|
|
|
|
|
|
|
|
|
|
|
|
|
|
|
|
|
|
|
|
|
|
|
|
|
|
|
|
|
|
|
|
|
|
|
|
|
|
|
|
|
|
|
|
|
|
|
|
|
|
|
|
|
|
|
|
|
|
|
|
|
|
|
|
|
|
|
|
|
|
|
|
|
|
|
|
|
|
|
|
|
|
|
|
|
|
|
|
|
|
|
|
|
|
|
|
|
|
|
|
|
|
|
|
|
|
|
|
|
|
|
|
|
|
|
|
|
|
|
|
|
|
|
|
|
|
|
|
|
|
|
|
|
|
|
|
|
|
|
|
|
|
|
|
|
|
|
|
|
|
|
|
|
|
|
|
|
|
|
|
|
|
|
|
|
|
|
|
|
|
|
|
|
|
|
|
|
|
|
|
|
|
|
|
|
|
|
|
|
|
|
|
|
|
|
|
|
|
|
|
|
|
|
|
|
|
|
|
|
|
|
|
|
|
|
|
|
|
|
|
|
|
|
|
|
|
|
|
|
|
|
|
|
|
|
|
|
|
|
|
|
|
|
|
|
|
|
|
|
|
|
|
|
|
|
|
|
|
|
|
|
|
|
|
|
|
|
|
|
|
|
|
|
|
|
|
|
|
|
|
|
|
|
|
|
|
|
|
|
|
|
|
|
|
|
|
|
|
|
|
|
|
|
|
|
|
|
|
|
|
|
|
|
|
|
|
|
|
|
|
|
|
|
|
|
|
|
|
|
|
|
|
|
|
|
|
|
|
|
|
|
|
|
|
|
|
|
|
|
|
|
|
|
|
|
|
|
|
|
|
|
|
|
|
|
|
|
|
|
|
|
|
|
|
|
|
|
|
|
|
|
|
|
|
|
|
|
|
|
|
|
|
|
|
|
|
|
|
|
|
|
|
|
|
|
|
|
|
|
|
|
|
|
|
|
|
|
|
|
|
|
|
|
|
|
|
|
|
|
|
|
|
|
|
|
|
|
|
|
|
|
|
|
|
|
|
|
|
|
|
|
|
|
|
|
|
|
|
|
|
|
|
|
|
|
|
|
|
|
|
|
|
|
|
|
|
|
|
|
|
|
|
|
|
|
|
|
|
|
|
|
|
|
|
|
|
|
|
|
|
|
|
|
|
|
|
|
|
|
|
|
|
|
|
|
|
|
|
|
|
|
|
|
|
|
|
|
|
|
|
|
|
|
|
|
|
|
|
|
|
|
|
|
|
|
|
|
|
|
|
|
|
|
|
|
|
|
|
|
|
|
|
|
|
|
|
|
|
|
|
|
|
|
|
|
|
|
|
|
|
|
|
|
|
|
|
|
|
|
|
|
|
|
|
|
|
|
|
|
|
|
|
|
|
|
|
|
|
|
|
|
|
|
|
|
|
|
|
|
|
|
|
|
|
|
|
|
|
|
|
|
|
|
|
|
|
|
|
|
|
|
|
|
|
|
|
|
|
|
|
|
|
|
|
|
|
|
|
|
|
|
|
|
|
|
|
|
|
|
|
|
|
|
|
|
|
|
|
|
|
|
|
|
|
|
|
|
|
|
|
|
|
|
|
|
|
|
|
|
|
|
|
|
|
|
|
|
|
|
|
|
|
|
|
|
|
|
|
|
|
|
|
|
|
|
|
|
|
|
|
|
|
|
|
|
|
|
|
|
|
|
|
|
|
|
|
|
|
|
|
|
|
|
|
|
|
|
|
|
|
|
|
|
|
|
|
|
|
|
|
|
|
|
|
|
|
|
|
|
|
|
|
|
|
|
|
|
|
|
|
|
|
|
|
|
|
|
|
|
|
|
|
|
|
|
|
|
|
|
|
|
|
|
|
|
|
|
|
|
|
|
|
|
|
|
|
|
|
|
|
|
|
|
|
|
|
|
|
|
|
|
|
|
|
|
|
|
|
|
|
|
|
|
|
|
|
|
|
|
|
|
|
|
|
|
|
|
|
|
|
|
|
|
|
|
|
|
|
|
|
|
|
|
|
|
|
|
|
|
|
|
|
|
|
|
|
|
|
|
|
|
|
|
|
|
|
|
|
|
|
|
|
|
|
|
|
|
|
|
|
|
|
|
|
|
|
|
|
1 |
+
# Ke Chen
|
2 | |
3 |
+
# HTS-AT: A HIERARCHICAL TOKEN-SEMANTIC AUDIO TRANSFORMER FOR SOUND CLASSIFICATION AND DETECTION
|
4 |
+
# Some layers designed on the model
|
5 |
+
# below codes are based and referred from https://github.com/microsoft/Swin-Transformer
|
6 |
+
# Swin Transformer for Computer Vision: https://arxiv.org/pdf/2103.14030.pdf
|
7 |
+
|
8 |
+
import torch
|
9 |
+
import torch.nn as nn
|
10 |
+
import torch.nn.functional as F
|
11 |
+
from itertools import repeat
|
12 |
+
import collections.abc
|
13 |
+
import math
|
14 |
+
import warnings
|
15 |
+
|
16 |
+
from torch.nn.init import _calculate_fan_in_and_fan_out
|
17 |
+
import torch.utils.checkpoint as checkpoint
|
18 |
+
|
19 |
+
import random
|
20 |
+
|
21 |
+
from torchlibrosa.stft import Spectrogram, LogmelFilterBank
|
22 |
+
from torchlibrosa.augmentation import SpecAugmentation
|
23 |
+
|
24 |
+
from itertools import repeat
|
25 |
+
from .utils import do_mixup, interpolate
|
26 |
+
|
27 |
+
from .feature_fusion import iAFF, AFF, DAF
|
28 |
+
|
29 |
+
# from PyTorch internals
|
30 |
+
def _ntuple(n):
|
31 |
+
def parse(x):
|
32 |
+
if isinstance(x, collections.abc.Iterable):
|
33 |
+
return x
|
34 |
+
return tuple(repeat(x, n))
|
35 |
+
|
36 |
+
return parse
|
37 |
+
|
38 |
+
|
39 |
+
to_1tuple = _ntuple(1)
|
40 |
+
to_2tuple = _ntuple(2)
|
41 |
+
to_3tuple = _ntuple(3)
|
42 |
+
to_4tuple = _ntuple(4)
|
43 |
+
to_ntuple = _ntuple
|
44 |
+
|
45 |
+
|
46 |
+
def drop_path(x, drop_prob: float = 0.0, training: bool = False):
|
47 |
+
"""Drop paths (Stochastic Depth) per sample (when applied in main path of residual blocks).
|
48 |
+
This is the same as the DropConnect impl I created for EfficientNet, etc networks, however,
|
49 |
+
the original name is misleading as 'Drop Connect' is a different form of dropout in a separate paper...
|
50 |
+
See discussion: https://github.com/tensorflow/tpu/issues/494#issuecomment-532968956 ... I've opted for
|
51 |
+
changing the layer and argument names to 'drop path' rather than mix DropConnect as a layer name and use
|
52 |
+
'survival rate' as the argument.
|
53 |
+
"""
|
54 |
+
if drop_prob == 0.0 or not training:
|
55 |
+
return x
|
56 |
+
keep_prob = 1 - drop_prob
|
57 |
+
shape = (x.shape[0],) + (1,) * (
|
58 |
+
x.ndim - 1
|
59 |
+
) # work with diff dim tensors, not just 2D ConvNets
|
60 |
+
random_tensor = keep_prob + torch.rand(shape, dtype=x.dtype, device=x.device)
|
61 |
+
random_tensor.floor_() # binarize
|
62 |
+
output = x.div(keep_prob) * random_tensor
|
63 |
+
return output
|
64 |
+
|
65 |
+
|
66 |
+
class DropPath(nn.Module):
|
67 |
+
"""Drop paths (Stochastic Depth) per sample (when applied in main path of residual blocks)."""
|
68 |
+
|
69 |
+
def __init__(self, drop_prob=None):
|
70 |
+
super(DropPath, self).__init__()
|
71 |
+
self.drop_prob = drop_prob
|
72 |
+
|
73 |
+
def forward(self, x):
|
74 |
+
return drop_path(x, self.drop_prob, self.training)
|
75 |
+
|
76 |
+
|
77 |
+
class PatchEmbed(nn.Module):
|
78 |
+
"""2D Image to Patch Embedding"""
|
79 |
+
|
80 |
+
def __init__(
|
81 |
+
self,
|
82 |
+
img_size=224,
|
83 |
+
patch_size=16,
|
84 |
+
in_chans=3,
|
85 |
+
embed_dim=768,
|
86 |
+
norm_layer=None,
|
87 |
+
flatten=True,
|
88 |
+
patch_stride=16,
|
89 |
+
enable_fusion=False,
|
90 |
+
fusion_type="None",
|
91 |
+
):
|
92 |
+
super().__init__()
|
93 |
+
img_size = to_2tuple(img_size)
|
94 |
+
patch_size = to_2tuple(patch_size)
|
95 |
+
patch_stride = to_2tuple(patch_stride)
|
96 |
+
self.img_size = img_size
|
97 |
+
self.patch_size = patch_size
|
98 |
+
self.patch_stride = patch_stride
|
99 |
+
self.grid_size = (
|
100 |
+
img_size[0] // patch_stride[0],
|
101 |
+
img_size[1] // patch_stride[1],
|
102 |
+
)
|
103 |
+
self.num_patches = self.grid_size[0] * self.grid_size[1]
|
104 |
+
self.flatten = flatten
|
105 |
+
self.in_chans = in_chans
|
106 |
+
self.embed_dim = embed_dim
|
107 |
+
|
108 |
+
self.enable_fusion = enable_fusion
|
109 |
+
self.fusion_type = fusion_type
|
110 |
+
|
111 |
+
padding = (
|
112 |
+
(patch_size[0] - patch_stride[0]) // 2,
|
113 |
+
(patch_size[1] - patch_stride[1]) // 2,
|
114 |
+
)
|
115 |
+
|
116 |
+
if (self.enable_fusion) and (self.fusion_type == "channel_map"):
|
117 |
+
self.proj = nn.Conv2d(
|
118 |
+
in_chans * 4,
|
119 |
+
embed_dim,
|
120 |
+
kernel_size=patch_size,
|
121 |
+
stride=patch_stride,
|
122 |
+
padding=padding,
|
123 |
+
)
|
124 |
+
else:
|
125 |
+
self.proj = nn.Conv2d(
|
126 |
+
in_chans,
|
127 |
+
embed_dim,
|
128 |
+
kernel_size=patch_size,
|
129 |
+
stride=patch_stride,
|
130 |
+
padding=padding,
|
131 |
+
)
|
132 |
+
self.norm = norm_layer(embed_dim) if norm_layer else nn.Identity()
|
133 |
+
|
134 |
+
if (self.enable_fusion) and (
|
135 |
+
self.fusion_type in ["daf_2d", "aff_2d", "iaff_2d"]
|
136 |
+
):
|
137 |
+
self.mel_conv2d = nn.Conv2d(
|
138 |
+
in_chans,
|
139 |
+
embed_dim,
|
140 |
+
kernel_size=(patch_size[0], patch_size[1] * 3),
|
141 |
+
stride=(patch_stride[0], patch_stride[1] * 3),
|
142 |
+
padding=padding,
|
143 |
+
)
|
144 |
+
if self.fusion_type == "daf_2d":
|
145 |
+
self.fusion_model = DAF()
|
146 |
+
elif self.fusion_type == "aff_2d":
|
147 |
+
self.fusion_model = AFF(channels=embed_dim, type="2D")
|
148 |
+
elif self.fusion_type == "iaff_2d":
|
149 |
+
self.fusion_model = iAFF(channels=embed_dim, type="2D")
|
150 |
+
|
151 |
+
def forward(self, x, longer_idx=None):
|
152 |
+
if (self.enable_fusion) and (
|
153 |
+
self.fusion_type in ["daf_2d", "aff_2d", "iaff_2d"]
|
154 |
+
):
|
155 |
+
global_x = x[:, 0:1, :, :]
|
156 |
+
|
157 |
+
# global processing
|
158 |
+
B, C, H, W = global_x.shape
|
159 |
+
assert (
|
160 |
+
H == self.img_size[0] and W == self.img_size[1]
|
161 |
+
), f"Input image size ({H}*{W}) doesn't match model ({self.img_size[0]}*{self.img_size[1]})."
|
162 |
+
global_x = self.proj(global_x)
|
163 |
+
TW = global_x.size(-1)
|
164 |
+
if len(longer_idx) > 0:
|
165 |
+
# local processing
|
166 |
+
local_x = x[longer_idx, 1:, :, :].contiguous()
|
167 |
+
B, C, H, W = local_x.shape
|
168 |
+
local_x = local_x.view(B * C, 1, H, W)
|
169 |
+
local_x = self.mel_conv2d(local_x)
|
170 |
+
local_x = local_x.view(
|
171 |
+
B, C, local_x.size(1), local_x.size(2), local_x.size(3)
|
172 |
+
)
|
173 |
+
local_x = local_x.permute((0, 2, 3, 1, 4)).contiguous().flatten(3)
|
174 |
+
TB, TC, TH, _ = local_x.size()
|
175 |
+
if local_x.size(-1) < TW:
|
176 |
+
local_x = torch.cat(
|
177 |
+
[
|
178 |
+
local_x,
|
179 |
+
torch.zeros(
|
180 |
+
(TB, TC, TH, TW - local_x.size(-1)),
|
181 |
+
device=global_x.device,
|
182 |
+
),
|
183 |
+
],
|
184 |
+
dim=-1,
|
185 |
+
)
|
186 |
+
else:
|
187 |
+
local_x = local_x[:, :, :, :TW]
|
188 |
+
|
189 |
+
global_x[longer_idx] = self.fusion_model(global_x[longer_idx], local_x)
|
190 |
+
x = global_x
|
191 |
+
else:
|
192 |
+
B, C, H, W = x.shape
|
193 |
+
assert (
|
194 |
+
H == self.img_size[0] and W == self.img_size[1]
|
195 |
+
), f"Input image size ({H}*{W}) doesn't match model ({self.img_size[0]}*{self.img_size[1]})."
|
196 |
+
x = self.proj(x)
|
197 |
+
|
198 |
+
if self.flatten:
|
199 |
+
x = x.flatten(2).transpose(1, 2) # BCHW -> BNC
|
200 |
+
x = self.norm(x)
|
201 |
+
return x
|
202 |
+
|
203 |
+
|
204 |
+
class Mlp(nn.Module):
|
205 |
+
"""MLP as used in Vision Transformer, MLP-Mixer and related networks"""
|
206 |
+
|
207 |
+
def __init__(
|
208 |
+
self,
|
209 |
+
in_features,
|
210 |
+
hidden_features=None,
|
211 |
+
out_features=None,
|
212 |
+
act_layer=nn.GELU,
|
213 |
+
drop=0.0,
|
214 |
+
):
|
215 |
+
super().__init__()
|
216 |
+
out_features = out_features or in_features
|
217 |
+
hidden_features = hidden_features or in_features
|
218 |
+
self.fc1 = nn.Linear(in_features, hidden_features)
|
219 |
+
self.act = act_layer()
|
220 |
+
self.fc2 = nn.Linear(hidden_features, out_features)
|
221 |
+
self.drop = nn.Dropout(drop)
|
222 |
+
|
223 |
+
def forward(self, x):
|
224 |
+
x = self.fc1(x)
|
225 |
+
x = self.act(x)
|
226 |
+
x = self.drop(x)
|
227 |
+
x = self.fc2(x)
|
228 |
+
x = self.drop(x)
|
229 |
+
return x
|
230 |
+
|
231 |
+
|
232 |
+
def _no_grad_trunc_normal_(tensor, mean, std, a, b):
|
233 |
+
# Cut & paste from PyTorch official master until it's in a few official releases - RW
|
234 |
+
# Method based on https://people.sc.fsu.edu/~jburkardt/presentations/truncated_normal.pdf
|
235 |
+
def norm_cdf(x):
|
236 |
+
# Computes standard normal cumulative distribution function
|
237 |
+
return (1.0 + math.erf(x / math.sqrt(2.0))) / 2.0
|
238 |
+
|
239 |
+
if (mean < a - 2 * std) or (mean > b + 2 * std):
|
240 |
+
warnings.warn(
|
241 |
+
"mean is more than 2 std from [a, b] in nn.init.trunc_normal_. "
|
242 |
+
"The distribution of values may be incorrect.",
|
243 |
+
stacklevel=2,
|
244 |
+
)
|
245 |
+
|
246 |
+
with torch.no_grad():
|
247 |
+
# Values are generated by using a truncated uniform distribution and
|
248 |
+
# then using the inverse CDF for the normal distribution.
|
249 |
+
# Get upper and lower cdf values
|
250 |
+
l = norm_cdf((a - mean) / std)
|
251 |
+
u = norm_cdf((b - mean) / std)
|
252 |
+
|
253 |
+
# Uniformly fill tensor with values from [l, u], then translate to
|
254 |
+
# [2l-1, 2u-1].
|
255 |
+
tensor.uniform_(2 * l - 1, 2 * u - 1)
|
256 |
+
|
257 |
+
# Use inverse cdf transform for normal distribution to get truncated
|
258 |
+
# standard normal
|
259 |
+
tensor.erfinv_()
|
260 |
+
|
261 |
+
# Transform to proper mean, std
|
262 |
+
tensor.mul_(std * math.sqrt(2.0))
|
263 |
+
tensor.add_(mean)
|
264 |
+
|
265 |
+
# Clamp to ensure it's in the proper range
|
266 |
+
tensor.clamp_(min=a, max=b)
|
267 |
+
return tensor
|
268 |
+
|
269 |
+
|
270 |
+
def trunc_normal_(tensor, mean=0.0, std=1.0, a=-2.0, b=2.0):
|
271 |
+
# type: (Tensor, float, float, float, float) -> Tensor
|
272 |
+
r"""Fills the input Tensor with values drawn from a truncated
|
273 |
+
normal distribution. The values are effectively drawn from the
|
274 |
+
normal distribution :math:`\mathcal{N}(\text{mean}, \text{std}^2)`
|
275 |
+
with values outside :math:`[a, b]` redrawn until they are within
|
276 |
+
the bounds. The method used for generating the random values works
|
277 |
+
best when :math:`a \leq \text{mean} \leq b`.
|
278 |
+
Args:
|
279 |
+
tensor: an n-dimensional `torch.Tensor`
|
280 |
+
mean: the mean of the normal distribution
|
281 |
+
std: the standard deviation of the normal distribution
|
282 |
+
a: the minimum cutoff value
|
283 |
+
b: the maximum cutoff value
|
284 |
+
Examples:
|
285 |
+
>>> w = torch.empty(3, 5)
|
286 |
+
>>> nn.init.trunc_normal_(w)
|
287 |
+
"""
|
288 |
+
return _no_grad_trunc_normal_(tensor, mean, std, a, b)
|
289 |
+
|
290 |
+
|
291 |
+
def variance_scaling_(tensor, scale=1.0, mode="fan_in", distribution="normal"):
|
292 |
+
fan_in, fan_out = _calculate_fan_in_and_fan_out(tensor)
|
293 |
+
if mode == "fan_in":
|
294 |
+
denom = fan_in
|
295 |
+
elif mode == "fan_out":
|
296 |
+
denom = fan_out
|
297 |
+
elif mode == "fan_avg":
|
298 |
+
denom = (fan_in + fan_out) / 2
|
299 |
+
|
300 |
+
variance = scale / denom
|
301 |
+
|
302 |
+
if distribution == "truncated_normal":
|
303 |
+
# constant is stddev of standard normal truncated to (-2, 2)
|
304 |
+
trunc_normal_(tensor, std=math.sqrt(variance) / 0.87962566103423978)
|
305 |
+
elif distribution == "normal":
|
306 |
+
tensor.normal_(std=math.sqrt(variance))
|
307 |
+
elif distribution == "uniform":
|
308 |
+
bound = math.sqrt(3 * variance)
|
309 |
+
tensor.uniform_(-bound, bound)
|
310 |
+
else:
|
311 |
+
raise ValueError(f"invalid distribution {distribution}")
|
312 |
+
|
313 |
+
|
314 |
+
def lecun_normal_(tensor):
|
315 |
+
variance_scaling_(tensor, mode="fan_in", distribution="truncated_normal")
|
316 |
+
|
317 |
+
|
318 |
+
def window_partition(x, window_size):
|
319 |
+
"""
|
320 |
+
Args:
|
321 |
+
x: (B, H, W, C)
|
322 |
+
window_size (int): window size
|
323 |
+
Returns:
|
324 |
+
windows: (num_windows*B, window_size, window_size, C)
|
325 |
+
"""
|
326 |
+
B, H, W, C = x.shape
|
327 |
+
x = x.view(B, H // window_size, window_size, W // window_size, window_size, C)
|
328 |
+
windows = (
|
329 |
+
x.permute(0, 1, 3, 2, 4, 5).contiguous().view(-1, window_size, window_size, C)
|
330 |
+
)
|
331 |
+
return windows
|
332 |
+
|
333 |
+
|
334 |
+
def window_reverse(windows, window_size, H, W):
|
335 |
+
"""
|
336 |
+
Args:
|
337 |
+
windows: (num_windows*B, window_size, window_size, C)
|
338 |
+
window_size (int): Window size
|
339 |
+
H (int): Height of image
|
340 |
+
W (int): Width of image
|
341 |
+
Returns:
|
342 |
+
x: (B, H, W, C)
|
343 |
+
"""
|
344 |
+
B = int(windows.shape[0] / (H * W / window_size / window_size))
|
345 |
+
x = windows.view(
|
346 |
+
B, H // window_size, W // window_size, window_size, window_size, -1
|
347 |
+
)
|
348 |
+
x = x.permute(0, 1, 3, 2, 4, 5).contiguous().view(B, H, W, -1)
|
349 |
+
return x
|
350 |
+
|
351 |
+
|
352 |
+
class WindowAttention(nn.Module):
|
353 |
+
r"""Window based multi-head self attention (W-MSA) module with relative position bias.
|
354 |
+
It supports both of shifted and non-shifted window.
|
355 |
+
Args:
|
356 |
+
dim (int): Number of input channels.
|
357 |
+
window_size (tuple[int]): The height and width of the window.
|
358 |
+
num_heads (int): Number of attention heads.
|
359 |
+
qkv_bias (bool, optional): If True, add a learnable bias to query, key, value. Default: True
|
360 |
+
qk_scale (float | None, optional): Override default qk scale of head_dim ** -0.5 if set
|
361 |
+
attn_drop (float, optional): Dropout ratio of attention weight. Default: 0.0
|
362 |
+
proj_drop (float, optional): Dropout ratio of output. Default: 0.0
|
363 |
+
"""
|
364 |
+
|
365 |
+
def __init__(
|
366 |
+
self,
|
367 |
+
dim,
|
368 |
+
window_size,
|
369 |
+
num_heads,
|
370 |
+
qkv_bias=True,
|
371 |
+
qk_scale=None,
|
372 |
+
attn_drop=0.0,
|
373 |
+
proj_drop=0.0,
|
374 |
+
):
|
375 |
+
|
376 |
+
super().__init__()
|
377 |
+
self.dim = dim
|
378 |
+
self.window_size = window_size # Wh, Ww
|
379 |
+
self.num_heads = num_heads
|
380 |
+
head_dim = dim // num_heads
|
381 |
+
self.scale = qk_scale or head_dim**-0.5
|
382 |
+
|
383 |
+
# define a parameter table of relative position bias
|
384 |
+
self.relative_position_bias_table = nn.Parameter(
|
385 |
+
torch.zeros((2 * window_size[0] - 1) * (2 * window_size[1] - 1), num_heads)
|
386 |
+
) # 2*Wh-1 * 2*Ww-1, nH
|
387 |
+
|
388 |
+
# get pair-wise relative position index for each token inside the window
|
389 |
+
coords_h = torch.arange(self.window_size[0])
|
390 |
+
coords_w = torch.arange(self.window_size[1])
|
391 |
+
coords = torch.stack(torch.meshgrid([coords_h, coords_w])) # 2, Wh, Ww
|
392 |
+
coords_flatten = torch.flatten(coords, 1) # 2, Wh*Ww
|
393 |
+
relative_coords = (
|
394 |
+
coords_flatten[:, :, None] - coords_flatten[:, None, :]
|
395 |
+
) # 2, Wh*Ww, Wh*Ww
|
396 |
+
relative_coords = relative_coords.permute(
|
397 |
+
1, 2, 0
|
398 |
+
).contiguous() # Wh*Ww, Wh*Ww, 2
|
399 |
+
relative_coords[:, :, 0] += self.window_size[0] - 1 # shift to start from 0
|
400 |
+
relative_coords[:, :, 1] += self.window_size[1] - 1
|
401 |
+
relative_coords[:, :, 0] *= 2 * self.window_size[1] - 1
|
402 |
+
relative_position_index = relative_coords.sum(-1) # Wh*Ww, Wh*Ww
|
403 |
+
self.register_buffer("relative_position_index", relative_position_index)
|
404 |
+
|
405 |
+
self.qkv = nn.Linear(dim, dim * 3, bias=qkv_bias)
|
406 |
+
self.attn_drop = nn.Dropout(attn_drop)
|
407 |
+
self.proj = nn.Linear(dim, dim)
|
408 |
+
self.proj_drop = nn.Dropout(proj_drop)
|
409 |
+
|
410 |
+
trunc_normal_(self.relative_position_bias_table, std=0.02)
|
411 |
+
self.softmax = nn.Softmax(dim=-1)
|
412 |
+
|
413 |
+
def forward(self, x, mask=None):
|
414 |
+
"""
|
415 |
+
Args:
|
416 |
+
x: input features with shape of (num_windows*B, N, C)
|
417 |
+
mask: (0/-inf) mask with shape of (num_windows, Wh*Ww, Wh*Ww) or None
|
418 |
+
"""
|
419 |
+
B_, N, C = x.shape
|
420 |
+
qkv = (
|
421 |
+
self.qkv(x)
|
422 |
+
.reshape(B_, N, 3, self.num_heads, C // self.num_heads)
|
423 |
+
.permute(2, 0, 3, 1, 4)
|
424 |
+
)
|
425 |
+
q, k, v = (
|
426 |
+
qkv[0],
|
427 |
+
qkv[1],
|
428 |
+
qkv[2],
|
429 |
+
) # make torchscript happy (cannot use tensor as tuple)
|
430 |
+
|
431 |
+
q = q * self.scale
|
432 |
+
attn = q @ k.transpose(-2, -1)
|
433 |
+
|
434 |
+
relative_position_bias = self.relative_position_bias_table[
|
435 |
+
self.relative_position_index.view(-1)
|
436 |
+
].view(
|
437 |
+
self.window_size[0] * self.window_size[1],
|
438 |
+
self.window_size[0] * self.window_size[1],
|
439 |
+
-1,
|
440 |
+
) # Wh*Ww,Wh*Ww,nH
|
441 |
+
relative_position_bias = relative_position_bias.permute(
|
442 |
+
2, 0, 1
|
443 |
+
).contiguous() # nH, Wh*Ww, Wh*Ww
|
444 |
+
attn = attn + relative_position_bias.unsqueeze(0)
|
445 |
+
|
446 |
+
if mask is not None:
|
447 |
+
nW = mask.shape[0]
|
448 |
+
attn = attn.view(B_ // nW, nW, self.num_heads, N, N) + mask.unsqueeze(
|
449 |
+
1
|
450 |
+
).unsqueeze(0)
|
451 |
+
attn = attn.view(-1, self.num_heads, N, N)
|
452 |
+
attn = self.softmax(attn)
|
453 |
+
else:
|
454 |
+
attn = self.softmax(attn)
|
455 |
+
|
456 |
+
attn = self.attn_drop(attn)
|
457 |
+
|
458 |
+
x = (attn @ v).transpose(1, 2).reshape(B_, N, C)
|
459 |
+
x = self.proj(x)
|
460 |
+
x = self.proj_drop(x)
|
461 |
+
return x, attn
|
462 |
+
|
463 |
+
def extra_repr(self):
|
464 |
+
return f"dim={self.dim}, window_size={self.window_size}, num_heads={self.num_heads}"
|
465 |
+
|
466 |
+
|
467 |
+
# We use the model based on Swintransformer Block, therefore we can use the swin-transformer pretrained model
|
468 |
+
class SwinTransformerBlock(nn.Module):
|
469 |
+
r"""Swin Transformer Block.
|
470 |
+
Args:
|
471 |
+
dim (int): Number of input channels.
|
472 |
+
input_resolution (tuple[int]): Input resulotion.
|
473 |
+
num_heads (int): Number of attention heads.
|
474 |
+
window_size (int): Window size.
|
475 |
+
shift_size (int): Shift size for SW-MSA.
|
476 |
+
mlp_ratio (float): Ratio of mlp hidden dim to embedding dim.
|
477 |
+
qkv_bias (bool, optional): If True, add a learnable bias to query, key, value. Default: True
|
478 |
+
qk_scale (float | None, optional): Override default qk scale of head_dim ** -0.5 if set.
|
479 |
+
drop (float, optional): Dropout rate. Default: 0.0
|
480 |
+
attn_drop (float, optional): Attention dropout rate. Default: 0.0
|
481 |
+
drop_path (float, optional): Stochastic depth rate. Default: 0.0
|
482 |
+
act_layer (nn.Module, optional): Activation layer. Default: nn.GELU
|
483 |
+
norm_layer (nn.Module, optional): Normalization layer. Default: nn.LayerNorm
|
484 |
+
"""
|
485 |
+
|
486 |
+
def __init__(
|
487 |
+
self,
|
488 |
+
dim,
|
489 |
+
input_resolution,
|
490 |
+
num_heads,
|
491 |
+
window_size=7,
|
492 |
+
shift_size=0,
|
493 |
+
mlp_ratio=4.0,
|
494 |
+
qkv_bias=True,
|
495 |
+
qk_scale=None,
|
496 |
+
drop=0.0,
|
497 |
+
attn_drop=0.0,
|
498 |
+
drop_path=0.0,
|
499 |
+
act_layer=nn.GELU,
|
500 |
+
norm_layer=nn.LayerNorm,
|
501 |
+
norm_before_mlp="ln",
|
502 |
+
):
|
503 |
+
super().__init__()
|
504 |
+
self.dim = dim
|
505 |
+
self.input_resolution = input_resolution
|
506 |
+
self.num_heads = num_heads
|
507 |
+
self.window_size = window_size
|
508 |
+
self.shift_size = shift_size
|
509 |
+
self.mlp_ratio = mlp_ratio
|
510 |
+
self.norm_before_mlp = norm_before_mlp
|
511 |
+
if min(self.input_resolution) <= self.window_size:
|
512 |
+
# if window size is larger than input resolution, we don't partition windows
|
513 |
+
self.shift_size = 0
|
514 |
+
self.window_size = min(self.input_resolution)
|
515 |
+
assert (
|
516 |
+
0 <= self.shift_size < self.window_size
|
517 |
+
), "shift_size must in 0-window_size"
|
518 |
+
|
519 |
+
self.norm1 = norm_layer(dim)
|
520 |
+
self.attn = WindowAttention(
|
521 |
+
dim,
|
522 |
+
window_size=to_2tuple(self.window_size),
|
523 |
+
num_heads=num_heads,
|
524 |
+
qkv_bias=qkv_bias,
|
525 |
+
qk_scale=qk_scale,
|
526 |
+
attn_drop=attn_drop,
|
527 |
+
proj_drop=drop,
|
528 |
+
)
|
529 |
+
|
530 |
+
self.drop_path = DropPath(drop_path) if drop_path > 0.0 else nn.Identity()
|
531 |
+
if self.norm_before_mlp == "ln":
|
532 |
+
self.norm2 = nn.LayerNorm(dim)
|
533 |
+
elif self.norm_before_mlp == "bn":
|
534 |
+
self.norm2 = lambda x: nn.BatchNorm1d(dim)(x.transpose(1, 2)).transpose(
|
535 |
+
1, 2
|
536 |
+
)
|
537 |
+
else:
|
538 |
+
raise NotImplementedError
|
539 |
+
mlp_hidden_dim = int(dim * mlp_ratio)
|
540 |
+
self.mlp = Mlp(
|
541 |
+
in_features=dim,
|
542 |
+
hidden_features=mlp_hidden_dim,
|
543 |
+
act_layer=act_layer,
|
544 |
+
drop=drop,
|
545 |
+
)
|
546 |
+
|
547 |
+
if self.shift_size > 0:
|
548 |
+
# calculate attention mask for SW-MSA
|
549 |
+
H, W = self.input_resolution
|
550 |
+
img_mask = torch.zeros((1, H, W, 1)) # 1 H W 1
|
551 |
+
h_slices = (
|
552 |
+
slice(0, -self.window_size),
|
553 |
+
slice(-self.window_size, -self.shift_size),
|
554 |
+
slice(-self.shift_size, None),
|
555 |
+
)
|
556 |
+
w_slices = (
|
557 |
+
slice(0, -self.window_size),
|
558 |
+
slice(-self.window_size, -self.shift_size),
|
559 |
+
slice(-self.shift_size, None),
|
560 |
+
)
|
561 |
+
cnt = 0
|
562 |
+
for h in h_slices:
|
563 |
+
for w in w_slices:
|
564 |
+
img_mask[:, h, w, :] = cnt
|
565 |
+
cnt += 1
|
566 |
+
|
567 |
+
mask_windows = window_partition(
|
568 |
+
img_mask, self.window_size
|
569 |
+
) # nW, window_size, window_size, 1
|
570 |
+
mask_windows = mask_windows.view(-1, self.window_size * self.window_size)
|
571 |
+
attn_mask = mask_windows.unsqueeze(1) - mask_windows.unsqueeze(2)
|
572 |
+
attn_mask = attn_mask.masked_fill(
|
573 |
+
attn_mask != 0, float(-100.0)
|
574 |
+
).masked_fill(attn_mask == 0, float(0.0))
|
575 |
+
else:
|
576 |
+
attn_mask = None
|
577 |
+
|
578 |
+
self.register_buffer("attn_mask", attn_mask)
|
579 |
+
|
580 |
+
def forward(self, x):
|
581 |
+
# pdb.set_trace()
|
582 |
+
H, W = self.input_resolution
|
583 |
+
# print("H: ", H)
|
584 |
+
# print("W: ", W)
|
585 |
+
# pdb.set_trace()
|
586 |
+
B, L, C = x.shape
|
587 |
+
# assert L == H * W, "input feature has wrong size"
|
588 |
+
|
589 |
+
shortcut = x
|
590 |
+
x = self.norm1(x)
|
591 |
+
x = x.view(B, H, W, C)
|
592 |
+
|
593 |
+
# cyclic shift
|
594 |
+
if self.shift_size > 0:
|
595 |
+
shifted_x = torch.roll(
|
596 |
+
x, shifts=(-self.shift_size, -self.shift_size), dims=(1, 2)
|
597 |
+
)
|
598 |
+
else:
|
599 |
+
shifted_x = x
|
600 |
+
|
601 |
+
# partition windows
|
602 |
+
x_windows = window_partition(
|
603 |
+
shifted_x, self.window_size
|
604 |
+
) # nW*B, window_size, window_size, C
|
605 |
+
x_windows = x_windows.view(
|
606 |
+
-1, self.window_size * self.window_size, C
|
607 |
+
) # nW*B, window_size*window_size, C
|
608 |
+
|
609 |
+
# W-MSA/SW-MSA
|
610 |
+
attn_windows, attn = self.attn(
|
611 |
+
x_windows, mask=self.attn_mask
|
612 |
+
) # nW*B, window_size*window_size, C
|
613 |
+
|
614 |
+
# merge windows
|
615 |
+
attn_windows = attn_windows.view(-1, self.window_size, self.window_size, C)
|
616 |
+
shifted_x = window_reverse(attn_windows, self.window_size, H, W) # B H' W' C
|
617 |
+
|
618 |
+
# reverse cyclic shift
|
619 |
+
if self.shift_size > 0:
|
620 |
+
x = torch.roll(
|
621 |
+
shifted_x, shifts=(self.shift_size, self.shift_size), dims=(1, 2)
|
622 |
+
)
|
623 |
+
else:
|
624 |
+
x = shifted_x
|
625 |
+
x = x.view(B, H * W, C)
|
626 |
+
|
627 |
+
# FFN
|
628 |
+
x = shortcut + self.drop_path(x)
|
629 |
+
x = x + self.drop_path(self.mlp(self.norm2(x)))
|
630 |
+
|
631 |
+
return x, attn
|
632 |
+
|
633 |
+
def extra_repr(self):
|
634 |
+
return (
|
635 |
+
f"dim={self.dim}, input_resolution={self.input_resolution}, num_heads={self.num_heads}, "
|
636 |
+
f"window_size={self.window_size}, shift_size={self.shift_size}, mlp_ratio={self.mlp_ratio}"
|
637 |
+
)
|
638 |
+
|
639 |
+
|
640 |
+
class PatchMerging(nn.Module):
|
641 |
+
r"""Patch Merging Layer.
|
642 |
+
Args:
|
643 |
+
input_resolution (tuple[int]): Resolution of input feature.
|
644 |
+
dim (int): Number of input channels.
|
645 |
+
norm_layer (nn.Module, optional): Normalization layer. Default: nn.LayerNorm
|
646 |
+
"""
|
647 |
+
|
648 |
+
def __init__(self, input_resolution, dim, norm_layer=nn.LayerNorm):
|
649 |
+
super().__init__()
|
650 |
+
self.input_resolution = input_resolution
|
651 |
+
self.dim = dim
|
652 |
+
self.reduction = nn.Linear(4 * dim, 2 * dim, bias=False)
|
653 |
+
self.norm = norm_layer(4 * dim)
|
654 |
+
|
655 |
+
def forward(self, x):
|
656 |
+
"""
|
657 |
+
x: B, H*W, C
|
658 |
+
"""
|
659 |
+
H, W = self.input_resolution
|
660 |
+
B, L, C = x.shape
|
661 |
+
assert L == H * W, "input feature has wrong size"
|
662 |
+
assert H % 2 == 0 and W % 2 == 0, f"x size ({H}*{W}) are not even."
|
663 |
+
|
664 |
+
x = x.view(B, H, W, C)
|
665 |
+
|
666 |
+
x0 = x[:, 0::2, 0::2, :] # B H/2 W/2 C
|
667 |
+
x1 = x[:, 1::2, 0::2, :] # B H/2 W/2 C
|
668 |
+
x2 = x[:, 0::2, 1::2, :] # B H/2 W/2 C
|
669 |
+
x3 = x[:, 1::2, 1::2, :] # B H/2 W/2 C
|
670 |
+
x = torch.cat([x0, x1, x2, x3], -1) # B H/2 W/2 4*C
|
671 |
+
x = x.view(B, -1, 4 * C) # B H/2*W/2 4*C
|
672 |
+
|
673 |
+
x = self.norm(x)
|
674 |
+
x = self.reduction(x)
|
675 |
+
|
676 |
+
return x
|
677 |
+
|
678 |
+
def extra_repr(self):
|
679 |
+
return f"input_resolution={self.input_resolution}, dim={self.dim}"
|
680 |
+
|
681 |
+
|
682 |
+
class BasicLayer(nn.Module):
|
683 |
+
"""A basic Swin Transformer layer for one stage.
|
684 |
+
Args:
|
685 |
+
dim (int): Number of input channels.
|
686 |
+
input_resolution (tuple[int]): Input resolution.
|
687 |
+
depth (int): Number of blocks.
|
688 |
+
num_heads (int): Number of attention heads.
|
689 |
+
window_size (int): Local window size.
|
690 |
+
mlp_ratio (float): Ratio of mlp hidden dim to embedding dim.
|
691 |
+
qkv_bias (bool, optional): If True, add a learnable bias to query, key, value. Default: True
|
692 |
+
qk_scale (float | None, optional): Override default qk scale of head_dim ** -0.5 if set.
|
693 |
+
drop (float, optional): Dropout rate. Default: 0.0
|
694 |
+
attn_drop (float, optional): Attention dropout rate. Default: 0.0
|
695 |
+
drop_path (float | tuple[float], optional): Stochastic depth rate. Default: 0.0
|
696 |
+
norm_layer (nn.Module, optional): Normalization layer. Default: nn.LayerNorm
|
697 |
+
downsample (nn.Module | None, optional): Downsample layer at the end of the layer. Default: None
|
698 |
+
use_checkpoint (bool): Whether to use checkpointing to save memory. Default: False.
|
699 |
+
"""
|
700 |
+
|
701 |
+
def __init__(
|
702 |
+
self,
|
703 |
+
dim,
|
704 |
+
input_resolution,
|
705 |
+
depth,
|
706 |
+
num_heads,
|
707 |
+
window_size,
|
708 |
+
mlp_ratio=4.0,
|
709 |
+
qkv_bias=True,
|
710 |
+
qk_scale=None,
|
711 |
+
drop=0.0,
|
712 |
+
attn_drop=0.0,
|
713 |
+
drop_path=0.0,
|
714 |
+
norm_layer=nn.LayerNorm,
|
715 |
+
downsample=None,
|
716 |
+
use_checkpoint=False,
|
717 |
+
norm_before_mlp="ln",
|
718 |
+
):
|
719 |
+
|
720 |
+
super().__init__()
|
721 |
+
self.dim = dim
|
722 |
+
self.input_resolution = input_resolution
|
723 |
+
self.depth = depth
|
724 |
+
self.use_checkpoint = use_checkpoint
|
725 |
+
|
726 |
+
# build blocks
|
727 |
+
self.blocks = nn.ModuleList(
|
728 |
+
[
|
729 |
+
SwinTransformerBlock(
|
730 |
+
dim=dim,
|
731 |
+
input_resolution=input_resolution,
|
732 |
+
num_heads=num_heads,
|
733 |
+
window_size=window_size,
|
734 |
+
shift_size=0 if (i % 2 == 0) else window_size // 2,
|
735 |
+
mlp_ratio=mlp_ratio,
|
736 |
+
qkv_bias=qkv_bias,
|
737 |
+
qk_scale=qk_scale,
|
738 |
+
drop=drop,
|
739 |
+
attn_drop=attn_drop,
|
740 |
+
drop_path=drop_path[i]
|
741 |
+
if isinstance(drop_path, list)
|
742 |
+
else drop_path,
|
743 |
+
norm_layer=norm_layer,
|
744 |
+
norm_before_mlp=norm_before_mlp,
|
745 |
+
)
|
746 |
+
for i in range(depth)
|
747 |
+
]
|
748 |
+
)
|
749 |
+
|
750 |
+
# patch merging layer
|
751 |
+
if downsample is not None:
|
752 |
+
self.downsample = downsample(
|
753 |
+
input_resolution, dim=dim, norm_layer=norm_layer
|
754 |
+
)
|
755 |
+
else:
|
756 |
+
self.downsample = None
|
757 |
+
|
758 |
+
def forward(self, x):
|
759 |
+
attns = []
|
760 |
+
for blk in self.blocks:
|
761 |
+
if self.use_checkpoint:
|
762 |
+
x = checkpoint.checkpoint(blk, x)
|
763 |
+
else:
|
764 |
+
x, attn = blk(x)
|
765 |
+
if not self.training:
|
766 |
+
attns.append(attn.unsqueeze(0))
|
767 |
+
if self.downsample is not None:
|
768 |
+
x = self.downsample(x)
|
769 |
+
if not self.training:
|
770 |
+
attn = torch.cat(attns, dim=0)
|
771 |
+
attn = torch.mean(attn, dim=0)
|
772 |
+
return x, attn
|
773 |
+
|
774 |
+
def extra_repr(self):
|
775 |
+
return f"dim={self.dim}, input_resolution={self.input_resolution}, depth={self.depth}"
|
776 |
+
|
777 |
+
|
778 |
+
# The Core of HTSAT
|
779 |
+
class HTSAT_Swin_Transformer(nn.Module):
|
780 |
+
r"""HTSAT based on the Swin Transformer
|
781 |
+
Args:
|
782 |
+
spec_size (int | tuple(int)): Input Spectrogram size. Default 256
|
783 |
+
patch_size (int | tuple(int)): Patch size. Default: 4
|
784 |
+
path_stride (iot | tuple(int)): Patch Stride for Frequency and Time Axis. Default: 4
|
785 |
+
in_chans (int): Number of input image channels. Default: 1 (mono)
|
786 |
+
num_classes (int): Number of classes for classification head. Default: 527
|
787 |
+
embed_dim (int): Patch embedding dimension. Default: 96
|
788 |
+
depths (tuple(int)): Depth of each HTSAT-Swin Transformer layer.
|
789 |
+
num_heads (tuple(int)): Number of attention heads in different layers.
|
790 |
+
window_size (int): Window size. Default: 8
|
791 |
+
mlp_ratio (float): Ratio of mlp hidden dim to embedding dim. Default: 4
|
792 |
+
qkv_bias (bool): If True, add a learnable bias to query, key, value. Default: True
|
793 |
+
qk_scale (float): Override default qk scale of head_dim ** -0.5 if set. Default: None
|
794 |
+
drop_rate (float): Dropout rate. Default: 0
|
795 |
+
attn_drop_rate (float): Attention dropout rate. Default: 0
|
796 |
+
drop_path_rate (float): Stochastic depth rate. Default: 0.1
|
797 |
+
norm_layer (nn.Module): Normalization layer. Default: nn.LayerNorm.
|
798 |
+
ape (bool): If True, add absolute position embedding to the patch embedding. Default: False
|
799 |
+
patch_norm (bool): If True, add normalization after patch embedding. Default: True
|
800 |
+
use_checkpoint (bool): Whether to use checkpointing to save memory. Default: False
|
801 |
+
config (module): The configuration Module from config.py
|
802 |
+
"""
|
803 |
+
|
804 |
+
def __init__(
|
805 |
+
self,
|
806 |
+
spec_size=256,
|
807 |
+
patch_size=4,
|
808 |
+
patch_stride=(4, 4),
|
809 |
+
in_chans=1,
|
810 |
+
num_classes=527,
|
811 |
+
embed_dim=96,
|
812 |
+
depths=[2, 2, 6, 2],
|
813 |
+
num_heads=[4, 8, 16, 32],
|
814 |
+
window_size=8,
|
815 |
+
mlp_ratio=4.0,
|
816 |
+
qkv_bias=True,
|
817 |
+
qk_scale=None,
|
818 |
+
drop_rate=0.0,
|
819 |
+
attn_drop_rate=0.0,
|
820 |
+
drop_path_rate=0.1,
|
821 |
+
norm_layer=nn.LayerNorm,
|
822 |
+
ape=False,
|
823 |
+
patch_norm=True,
|
824 |
+
use_checkpoint=False,
|
825 |
+
norm_before_mlp="ln",
|
826 |
+
config=None,
|
827 |
+
enable_fusion=False,
|
828 |
+
fusion_type="None",
|
829 |
+
**kwargs,
|
830 |
+
):
|
831 |
+
super(HTSAT_Swin_Transformer, self).__init__()
|
832 |
+
|
833 |
+
self.config = config
|
834 |
+
self.spec_size = spec_size
|
835 |
+
self.patch_stride = patch_stride
|
836 |
+
self.patch_size = patch_size
|
837 |
+
self.window_size = window_size
|
838 |
+
self.embed_dim = embed_dim
|
839 |
+
self.depths = depths
|
840 |
+
self.ape = ape
|
841 |
+
self.in_chans = in_chans
|
842 |
+
self.num_classes = num_classes
|
843 |
+
self.num_heads = num_heads
|
844 |
+
self.num_layers = len(self.depths)
|
845 |
+
self.num_features = int(self.embed_dim * 2 ** (self.num_layers - 1))
|
846 |
+
|
847 |
+
self.drop_rate = drop_rate
|
848 |
+
self.attn_drop_rate = attn_drop_rate
|
849 |
+
self.drop_path_rate = drop_path_rate
|
850 |
+
|
851 |
+
self.qkv_bias = qkv_bias
|
852 |
+
self.qk_scale = None
|
853 |
+
|
854 |
+
self.patch_norm = patch_norm
|
855 |
+
self.norm_layer = norm_layer if self.patch_norm else None
|
856 |
+
self.norm_before_mlp = norm_before_mlp
|
857 |
+
self.mlp_ratio = mlp_ratio
|
858 |
+
|
859 |
+
self.use_checkpoint = use_checkpoint
|
860 |
+
|
861 |
+
self.enable_fusion = enable_fusion
|
862 |
+
self.fusion_type = fusion_type
|
863 |
+
|
864 |
+
# process mel-spec ; used only once
|
865 |
+
self.freq_ratio = self.spec_size // self.config.mel_bins
|
866 |
+
window = "hann"
|
867 |
+
center = True
|
868 |
+
pad_mode = "reflect"
|
869 |
+
ref = 1.0
|
870 |
+
amin = 1e-10
|
871 |
+
top_db = None
|
872 |
+
self.interpolate_ratio = 32 # Downsampled ratio
|
873 |
+
# Spectrogram extractor
|
874 |
+
self.spectrogram_extractor = Spectrogram(
|
875 |
+
n_fft=config.window_size,
|
876 |
+
hop_length=config.hop_size,
|
877 |
+
win_length=config.window_size,
|
878 |
+
window=window,
|
879 |
+
center=center,
|
880 |
+
pad_mode=pad_mode,
|
881 |
+
freeze_parameters=True,
|
882 |
+
)
|
883 |
+
# Logmel feature extractor
|
884 |
+
self.logmel_extractor = LogmelFilterBank(
|
885 |
+
sr=config.sample_rate,
|
886 |
+
n_fft=config.window_size,
|
887 |
+
n_mels=config.mel_bins,
|
888 |
+
fmin=config.fmin,
|
889 |
+
fmax=config.fmax,
|
890 |
+
ref=ref,
|
891 |
+
amin=amin,
|
892 |
+
top_db=top_db,
|
893 |
+
freeze_parameters=True,
|
894 |
+
)
|
895 |
+
# Spec augmenter
|
896 |
+
self.spec_augmenter = SpecAugmentation(
|
897 |
+
time_drop_width=64,
|
898 |
+
time_stripes_num=2,
|
899 |
+
freq_drop_width=8,
|
900 |
+
freq_stripes_num=2,
|
901 |
+
) # 2 2
|
902 |
+
self.bn0 = nn.BatchNorm2d(self.config.mel_bins)
|
903 |
+
|
904 |
+
# split spctrogram into non-overlapping patches
|
905 |
+
self.patch_embed = PatchEmbed(
|
906 |
+
img_size=self.spec_size,
|
907 |
+
patch_size=self.patch_size,
|
908 |
+
in_chans=self.in_chans,
|
909 |
+
embed_dim=self.embed_dim,
|
910 |
+
norm_layer=self.norm_layer,
|
911 |
+
patch_stride=patch_stride,
|
912 |
+
enable_fusion=self.enable_fusion,
|
913 |
+
fusion_type=self.fusion_type,
|
914 |
+
)
|
915 |
+
|
916 |
+
num_patches = self.patch_embed.num_patches
|
917 |
+
patches_resolution = self.patch_embed.grid_size
|
918 |
+
self.patches_resolution = patches_resolution
|
919 |
+
|
920 |
+
# absolute position embedding
|
921 |
+
if self.ape:
|
922 |
+
self.absolute_pos_embed = nn.Parameter(
|
923 |
+
torch.zeros(1, num_patches, self.embed_dim)
|
924 |
+
)
|
925 |
+
trunc_normal_(self.absolute_pos_embed, std=0.02)
|
926 |
+
|
927 |
+
self.pos_drop = nn.Dropout(p=self.drop_rate)
|
928 |
+
|
929 |
+
# stochastic depth
|
930 |
+
dpr = [
|
931 |
+
x.item() for x in torch.linspace(0, self.drop_path_rate, sum(self.depths))
|
932 |
+
] # stochastic depth decay rule
|
933 |
+
|
934 |
+
# build layers
|
935 |
+
self.layers = nn.ModuleList()
|
936 |
+
for i_layer in range(self.num_layers):
|
937 |
+
layer = BasicLayer(
|
938 |
+
dim=int(self.embed_dim * 2**i_layer),
|
939 |
+
input_resolution=(
|
940 |
+
patches_resolution[0] // (2**i_layer),
|
941 |
+
patches_resolution[1] // (2**i_layer),
|
942 |
+
),
|
943 |
+
depth=self.depths[i_layer],
|
944 |
+
num_heads=self.num_heads[i_layer],
|
945 |
+
window_size=self.window_size,
|
946 |
+
mlp_ratio=self.mlp_ratio,
|
947 |
+
qkv_bias=self.qkv_bias,
|
948 |
+
qk_scale=self.qk_scale,
|
949 |
+
drop=self.drop_rate,
|
950 |
+
attn_drop=self.attn_drop_rate,
|
951 |
+
drop_path=dpr[
|
952 |
+
sum(self.depths[:i_layer]) : sum(self.depths[: i_layer + 1])
|
953 |
+
],
|
954 |
+
norm_layer=self.norm_layer,
|
955 |
+
downsample=PatchMerging if (i_layer < self.num_layers - 1) else None,
|
956 |
+
use_checkpoint=use_checkpoint,
|
957 |
+
norm_before_mlp=self.norm_before_mlp,
|
958 |
+
)
|
959 |
+
self.layers.append(layer)
|
960 |
+
|
961 |
+
self.norm = self.norm_layer(self.num_features)
|
962 |
+
self.avgpool = nn.AdaptiveAvgPool1d(1)
|
963 |
+
self.maxpool = nn.AdaptiveMaxPool1d(1)
|
964 |
+
|
965 |
+
SF = (
|
966 |
+
self.spec_size
|
967 |
+
// (2 ** (len(self.depths) - 1))
|
968 |
+
// self.patch_stride[0]
|
969 |
+
// self.freq_ratio
|
970 |
+
)
|
971 |
+
self.tscam_conv = nn.Conv2d(
|
972 |
+
in_channels=self.num_features,
|
973 |
+
out_channels=self.num_classes,
|
974 |
+
kernel_size=(SF, 3),
|
975 |
+
padding=(0, 1),
|
976 |
+
)
|
977 |
+
self.head = nn.Linear(num_classes, num_classes)
|
978 |
+
|
979 |
+
if (self.enable_fusion) and (
|
980 |
+
self.fusion_type in ["daf_1d", "aff_1d", "iaff_1d"]
|
981 |
+
):
|
982 |
+
self.mel_conv1d = nn.Sequential(
|
983 |
+
nn.Conv1d(64, 64, kernel_size=5, stride=3, padding=2),
|
984 |
+
nn.BatchNorm1d(64),
|
985 |
+
)
|
986 |
+
if self.fusion_type == "daf_1d":
|
987 |
+
self.fusion_model = DAF()
|
988 |
+
elif self.fusion_type == "aff_1d":
|
989 |
+
self.fusion_model = AFF(channels=64, type="1D")
|
990 |
+
elif self.fusion_type == "iaff_1d":
|
991 |
+
self.fusion_model = iAFF(channels=64, type="1D")
|
992 |
+
|
993 |
+
self.apply(self._init_weights)
|
994 |
+
|
995 |
+
def _init_weights(self, m):
|
996 |
+
if isinstance(m, nn.Linear):
|
997 |
+
trunc_normal_(m.weight, std=0.02)
|
998 |
+
if isinstance(m, nn.Linear) and m.bias is not None:
|
999 |
+
nn.init.constant_(m.bias, 0)
|
1000 |
+
elif isinstance(m, nn.LayerNorm):
|
1001 |
+
nn.init.constant_(m.bias, 0)
|
1002 |
+
nn.init.constant_(m.weight, 1.0)
|
1003 |
+
|
1004 |
+
@torch.jit.ignore
|
1005 |
+
def no_weight_decay(self):
|
1006 |
+
return {"absolute_pos_embed"}
|
1007 |
+
|
1008 |
+
@torch.jit.ignore
|
1009 |
+
def no_weight_decay_keywords(self):
|
1010 |
+
return {"relative_position_bias_table"}
|
1011 |
+
|
1012 |
+
def forward_features(self, x, longer_idx=None):
|
1013 |
+
# A deprecated optimization for using a hierarchical output from different blocks
|
1014 |
+
|
1015 |
+
frames_num = x.shape[2]
|
1016 |
+
x = self.patch_embed(x, longer_idx=longer_idx)
|
1017 |
+
if self.ape:
|
1018 |
+
x = x + self.absolute_pos_embed
|
1019 |
+
x = self.pos_drop(x)
|
1020 |
+
for i, layer in enumerate(self.layers):
|
1021 |
+
x, attn = layer(x)
|
1022 |
+
# for x
|
1023 |
+
x = self.norm(x)
|
1024 |
+
B, N, C = x.shape
|
1025 |
+
SF = frames_num // (2 ** (len(self.depths) - 1)) // self.patch_stride[0]
|
1026 |
+
ST = frames_num // (2 ** (len(self.depths) - 1)) // self.patch_stride[1]
|
1027 |
+
x = x.permute(0, 2, 1).contiguous().reshape(B, C, SF, ST)
|
1028 |
+
B, C, F, T = x.shape
|
1029 |
+
# group 2D CNN
|
1030 |
+
c_freq_bin = F // self.freq_ratio
|
1031 |
+
x = x.reshape(B, C, F // c_freq_bin, c_freq_bin, T)
|
1032 |
+
x = x.permute(0, 1, 3, 2, 4).contiguous().reshape(B, C, c_freq_bin, -1)
|
1033 |
+
# get latent_output
|
1034 |
+
fine_grained_latent_output = torch.mean(x, dim=2)
|
1035 |
+
fine_grained_latent_output = interpolate(
|
1036 |
+
fine_grained_latent_output.permute(0, 2, 1).contiguous(),
|
1037 |
+
8 * self.patch_stride[1],
|
1038 |
+
)
|
1039 |
+
|
1040 |
+
latent_output = self.avgpool(torch.flatten(x, 2))
|
1041 |
+
latent_output = torch.flatten(latent_output, 1)
|
1042 |
+
|
1043 |
+
# display the attention map, if needed
|
1044 |
+
|
1045 |
+
x = self.tscam_conv(x)
|
1046 |
+
x = torch.flatten(x, 2) # B, C, T
|
1047 |
+
|
1048 |
+
fpx = interpolate(
|
1049 |
+
torch.sigmoid(x).permute(0, 2, 1).contiguous(), 8 * self.patch_stride[1]
|
1050 |
+
)
|
1051 |
+
|
1052 |
+
x = self.avgpool(x)
|
1053 |
+
x = torch.flatten(x, 1)
|
1054 |
+
|
1055 |
+
output_dict = {
|
1056 |
+
"framewise_output": fpx, # already sigmoided
|
1057 |
+
"clipwise_output": torch.sigmoid(x),
|
1058 |
+
"fine_grained_embedding": fine_grained_latent_output,
|
1059 |
+
"embedding": latent_output,
|
1060 |
+
}
|
1061 |
+
|
1062 |
+
return output_dict
|
1063 |
+
|
1064 |
+
def crop_wav(self, x, crop_size, spe_pos=None):
|
1065 |
+
time_steps = x.shape[2]
|
1066 |
+
tx = torch.zeros(x.shape[0], x.shape[1], crop_size, x.shape[3]).to(x.device)
|
1067 |
+
for i in range(len(x)):
|
1068 |
+
if spe_pos is None:
|
1069 |
+
crop_pos = random.randint(0, time_steps - crop_size - 1)
|
1070 |
+
else:
|
1071 |
+
crop_pos = spe_pos
|
1072 |
+
tx[i][0] = x[i, 0, crop_pos : crop_pos + crop_size, :]
|
1073 |
+
return tx
|
1074 |
+
|
1075 |
+
# Reshape the wavform to a img size, if you want to use the pretrained swin transformer model
|
1076 |
+
def reshape_wav2img(self, x):
|
1077 |
+
B, C, T, F = x.shape
|
1078 |
+
target_T = int(self.spec_size * self.freq_ratio)
|
1079 |
+
target_F = self.spec_size // self.freq_ratio
|
1080 |
+
assert (
|
1081 |
+
T <= target_T and F <= target_F
|
1082 |
+
), "the wav size should less than or equal to the swin input size"
|
1083 |
+
# to avoid bicubic zero error
|
1084 |
+
if T < target_T:
|
1085 |
+
x = nn.functional.interpolate(
|
1086 |
+
x, (target_T, x.shape[3]), mode="bicubic", align_corners=True
|
1087 |
+
)
|
1088 |
+
if F < target_F:
|
1089 |
+
x = nn.functional.interpolate(
|
1090 |
+
x, (x.shape[2], target_F), mode="bicubic", align_corners=True
|
1091 |
+
)
|
1092 |
+
x = x.permute(0, 1, 3, 2).contiguous()
|
1093 |
+
x = x.reshape(
|
1094 |
+
x.shape[0],
|
1095 |
+
x.shape[1],
|
1096 |
+
x.shape[2],
|
1097 |
+
self.freq_ratio,
|
1098 |
+
x.shape[3] // self.freq_ratio,
|
1099 |
+
)
|
1100 |
+
# print(x.shape)
|
1101 |
+
x = x.permute(0, 1, 3, 2, 4).contiguous()
|
1102 |
+
x = x.reshape(x.shape[0], x.shape[1], x.shape[2] * x.shape[3], x.shape[4])
|
1103 |
+
return x
|
1104 |
+
|
1105 |
+
# Repeat the wavform to a img size, if you want to use the pretrained swin transformer model
|
1106 |
+
def repeat_wat2img(self, x, cur_pos):
|
1107 |
+
B, C, T, F = x.shape
|
1108 |
+
target_T = int(self.spec_size * self.freq_ratio)
|
1109 |
+
target_F = self.spec_size // self.freq_ratio
|
1110 |
+
assert (
|
1111 |
+
T <= target_T and F <= target_F
|
1112 |
+
), "the wav size should less than or equal to the swin input size"
|
1113 |
+
# to avoid bicubic zero error
|
1114 |
+
if T < target_T:
|
1115 |
+
x = nn.functional.interpolate(
|
1116 |
+
x, (target_T, x.shape[3]), mode="bicubic", align_corners=True
|
1117 |
+
)
|
1118 |
+
if F < target_F:
|
1119 |
+
x = nn.functional.interpolate(
|
1120 |
+
x, (x.shape[2], target_F), mode="bicubic", align_corners=True
|
1121 |
+
)
|
1122 |
+
x = x.permute(0, 1, 3, 2).contiguous() # B C F T
|
1123 |
+
x = x[:, :, :, cur_pos : cur_pos + self.spec_size]
|
1124 |
+
x = x.repeat(repeats=(1, 1, 4, 1))
|
1125 |
+
return x
|
1126 |
+
|
1127 |
+
def forward(
|
1128 |
+
self, x: torch.Tensor, mixup_lambda=None, infer_mode=False, device=None
|
1129 |
+
): # out_feat_keys: List[str] = None):
|
1130 |
+
|
1131 |
+
if self.enable_fusion and x["longer"].sum() == 0:
|
1132 |
+
# if no audio is longer than 10s, then randomly select one audio to be longer
|
1133 |
+
x["longer"][torch.randint(0, x["longer"].shape[0], (1,))] = True
|
1134 |
+
|
1135 |
+
if not self.enable_fusion:
|
1136 |
+
x = x["waveform"].to(device=device, non_blocking=True)
|
1137 |
+
x = self.spectrogram_extractor(x) # (batch_size, 1, time_steps, freq_bins)
|
1138 |
+
x = self.logmel_extractor(x) # (batch_size, 1, time_steps, mel_bins)
|
1139 |
+
x = x.transpose(1, 3)
|
1140 |
+
x = self.bn0(x)
|
1141 |
+
x = x.transpose(1, 3)
|
1142 |
+
if self.training:
|
1143 |
+
x = self.spec_augmenter(x)
|
1144 |
+
|
1145 |
+
if self.training and mixup_lambda is not None:
|
1146 |
+
x = do_mixup(x, mixup_lambda)
|
1147 |
+
|
1148 |
+
x = self.reshape_wav2img(x)
|
1149 |
+
output_dict = self.forward_features(x)
|
1150 |
+
else:
|
1151 |
+
longer_list = x["longer"].to(device=device, non_blocking=True)
|
1152 |
+
x = x["mel_fusion"].to(device=device, non_blocking=True)
|
1153 |
+
x = x.transpose(1, 3)
|
1154 |
+
x = self.bn0(x)
|
1155 |
+
x = x.transpose(1, 3)
|
1156 |
+
longer_list_idx = torch.where(longer_list)[0]
|
1157 |
+
if self.fusion_type in ["daf_1d", "aff_1d", "iaff_1d"]:
|
1158 |
+
new_x = x[:, 0:1, :, :].clone().contiguous()
|
1159 |
+
if len(longer_list_idx) > 0:
|
1160 |
+
# local processing
|
1161 |
+
fusion_x_local = x[longer_list_idx, 1:, :, :].clone().contiguous()
|
1162 |
+
FB, FC, FT, FF = fusion_x_local.size()
|
1163 |
+
fusion_x_local = fusion_x_local.view(FB * FC, FT, FF)
|
1164 |
+
fusion_x_local = torch.permute(
|
1165 |
+
fusion_x_local, (0, 2, 1)
|
1166 |
+
).contiguous()
|
1167 |
+
fusion_x_local = self.mel_conv1d(fusion_x_local)
|
1168 |
+
fusion_x_local = fusion_x_local.view(
|
1169 |
+
FB, FC, FF, fusion_x_local.size(-1)
|
1170 |
+
)
|
1171 |
+
fusion_x_local = (
|
1172 |
+
torch.permute(fusion_x_local, (0, 2, 1, 3))
|
1173 |
+
.contiguous()
|
1174 |
+
.flatten(2)
|
1175 |
+
)
|
1176 |
+
if fusion_x_local.size(-1) < FT:
|
1177 |
+
fusion_x_local = torch.cat(
|
1178 |
+
[
|
1179 |
+
fusion_x_local,
|
1180 |
+
torch.zeros(
|
1181 |
+
(FB, FF, FT - fusion_x_local.size(-1)),
|
1182 |
+
device=device,
|
1183 |
+
),
|
1184 |
+
],
|
1185 |
+
dim=-1,
|
1186 |
+
)
|
1187 |
+
else:
|
1188 |
+
fusion_x_local = fusion_x_local[:, :, :FT]
|
1189 |
+
# 1D fusion
|
1190 |
+
new_x = new_x.squeeze(1).permute((0, 2, 1)).contiguous()
|
1191 |
+
new_x[longer_list_idx] = self.fusion_model(
|
1192 |
+
new_x[longer_list_idx], fusion_x_local
|
1193 |
+
)
|
1194 |
+
x = new_x.permute((0, 2, 1)).contiguous()[:, None, :, :]
|
1195 |
+
else:
|
1196 |
+
x = new_x
|
1197 |
+
|
1198 |
+
elif self.fusion_type in ["daf_2d", "aff_2d", "iaff_2d", "channel_map"]:
|
1199 |
+
x = x # no change
|
1200 |
+
|
1201 |
+
if self.training:
|
1202 |
+
x = self.spec_augmenter(x)
|
1203 |
+
if self.training and mixup_lambda is not None:
|
1204 |
+
x = do_mixup(x, mixup_lambda)
|
1205 |
+
|
1206 |
+
x = self.reshape_wav2img(x)
|
1207 |
+
output_dict = self.forward_features(x, longer_idx=longer_list_idx)
|
1208 |
+
|
1209 |
+
# if infer_mode:
|
1210 |
+
# # in infer mode. we need to handle different length audio input
|
1211 |
+
# frame_num = x.shape[2]
|
1212 |
+
# target_T = int(self.spec_size * self.freq_ratio)
|
1213 |
+
# repeat_ratio = math.floor(target_T / frame_num)
|
1214 |
+
# x = x.repeat(repeats=(1,1,repeat_ratio,1))
|
1215 |
+
# x = self.reshape_wav2img(x)
|
1216 |
+
# output_dict = self.forward_features(x)
|
1217 |
+
# else:
|
1218 |
+
# if x.shape[2] > self.freq_ratio * self.spec_size:
|
1219 |
+
# if self.training:
|
1220 |
+
# x = self.crop_wav(x, crop_size=self.freq_ratio * self.spec_size)
|
1221 |
+
# x = self.reshape_wav2img(x)
|
1222 |
+
# output_dict = self.forward_features(x)
|
1223 |
+
# else:
|
1224 |
+
# # Change: Hard code here
|
1225 |
+
# overlap_size = (x.shape[2] - 1) // 4
|
1226 |
+
# output_dicts = []
|
1227 |
+
# crop_size = (x.shape[2] - 1) // 2
|
1228 |
+
# for cur_pos in range(0, x.shape[2] - crop_size - 1, overlap_size):
|
1229 |
+
# tx = self.crop_wav(x, crop_size = crop_size, spe_pos = cur_pos)
|
1230 |
+
# tx = self.reshape_wav2img(tx)
|
1231 |
+
# output_dicts.append(self.forward_features(tx))
|
1232 |
+
# clipwise_output = torch.zeros_like(output_dicts[0]["clipwise_output"]).float().to(x.device)
|
1233 |
+
# framewise_output = torch.zeros_like(output_dicts[0]["framewise_output"]).float().to(x.device)
|
1234 |
+
# for d in output_dicts:
|
1235 |
+
# clipwise_output += d["clipwise_output"]
|
1236 |
+
# framewise_output += d["framewise_output"]
|
1237 |
+
# clipwise_output = clipwise_output / len(output_dicts)
|
1238 |
+
# framewise_output = framewise_output / len(output_dicts)
|
1239 |
+
# output_dict = {
|
1240 |
+
# 'framewise_output': framewise_output,
|
1241 |
+
# 'clipwise_output': clipwise_output
|
1242 |
+
# }
|
1243 |
+
# else: # this part is typically used, and most easy one
|
1244 |
+
# x = self.reshape_wav2img(x)
|
1245 |
+
# output_dict = self.forward_features(x)
|
1246 |
+
# x = self.head(x)
|
1247 |
+
|
1248 |
+
# We process the data in the dataloader part, in that here we only consider the input_T < fixed_T
|
1249 |
+
|
1250 |
+
return output_dict
|
1251 |
+
|
1252 |
+
|
1253 |
+
def create_htsat_model(audio_cfg, enable_fusion=False, fusion_type="None"):
|
1254 |
+
try:
|
1255 |
+
|
1256 |
+
assert audio_cfg.model_name in [
|
1257 |
+
"tiny",
|
1258 |
+
"base",
|
1259 |
+
"large",
|
1260 |
+
], "model name for HTS-AT is wrong!"
|
1261 |
+
if audio_cfg.model_name == "tiny":
|
1262 |
+
model = HTSAT_Swin_Transformer(
|
1263 |
+
spec_size=256,
|
1264 |
+
patch_size=4,
|
1265 |
+
patch_stride=(4, 4),
|
1266 |
+
num_classes=audio_cfg.class_num,
|
1267 |
+
embed_dim=96,
|
1268 |
+
depths=[2, 2, 6, 2],
|
1269 |
+
num_heads=[4, 8, 16, 32],
|
1270 |
+
window_size=8,
|
1271 |
+
config=audio_cfg,
|
1272 |
+
enable_fusion=enable_fusion,
|
1273 |
+
fusion_type=fusion_type,
|
1274 |
+
)
|
1275 |
+
elif audio_cfg.model_name == "base":
|
1276 |
+
model = HTSAT_Swin_Transformer(
|
1277 |
+
spec_size=256,
|
1278 |
+
patch_size=4,
|
1279 |
+
patch_stride=(4, 4),
|
1280 |
+
num_classes=audio_cfg.class_num,
|
1281 |
+
embed_dim=128,
|
1282 |
+
depths=[2, 2, 12, 2],
|
1283 |
+
num_heads=[4, 8, 16, 32],
|
1284 |
+
window_size=8,
|
1285 |
+
config=audio_cfg,
|
1286 |
+
enable_fusion=enable_fusion,
|
1287 |
+
fusion_type=fusion_type,
|
1288 |
+
)
|
1289 |
+
elif audio_cfg.model_name == "large":
|
1290 |
+
model = HTSAT_Swin_Transformer(
|
1291 |
+
spec_size=256,
|
1292 |
+
patch_size=4,
|
1293 |
+
patch_stride=(4, 4),
|
1294 |
+
num_classes=audio_cfg.class_num,
|
1295 |
+
embed_dim=256,
|
1296 |
+
depths=[2, 2, 12, 2],
|
1297 |
+
num_heads=[4, 8, 16, 32],
|
1298 |
+
window_size=8,
|
1299 |
+
config=audio_cfg,
|
1300 |
+
enable_fusion=enable_fusion,
|
1301 |
+
fusion_type=fusion_type,
|
1302 |
+
)
|
1303 |
+
|
1304 |
+
return model
|
1305 |
+
except:
|
1306 |
+
raise RuntimeError(
|
1307 |
+
f"Import Model for {audio_cfg.model_name} not found, or the audio cfg parameters are not enough."
|
1308 |
+
)
|
src/audioldm/clap/open_clip/linear_probe.py
ADDED
@@ -0,0 +1,66 @@
|
|
|
|
|
|
|
|
|
|
|
|
|
|
|
|
|
|
|
|
|
|
|
|
|
|
|
|
|
|
|
|
|
|
|
|
|
|
|
|
|
|
|
|
|
|
|
|
|
|
|
|
|
|
|
|
|
|
|
|
|
|
|
|
|
|
|
|
|
|
|
|
|
|
|
|
|
|
|
|
|
|
|
|
|
|
|
|
|
|
|
|
|
|
|
|
|
|
|
|
|
|
|
|
|
|
|
|
|
|
|
|
|
|
|
|
|
|
|
|
|
|
|
|
|
|
|
|
|
|
|
|
|
|
|
1 |
+
import numpy as np
|
2 |
+
import torch.nn.functional as F
|
3 |
+
from torch import nn
|
4 |
+
from .model import MLPLayers
|
5 |
+
|
6 |
+
|
7 |
+
class LinearProbe(nn.Module):
|
8 |
+
def __init__(self, model, mlp, freeze, in_ch, out_ch, act=None):
|
9 |
+
"""
|
10 |
+
Args:
|
11 |
+
model: nn.Module
|
12 |
+
mlp: bool, if True, then use the MLP layer as the linear probe module
|
13 |
+
freeze: bool, if Ture, then freeze all the CLAP model's layers when training the linear probe
|
14 |
+
in_ch: int, the output channel from CLAP model
|
15 |
+
out_ch: int, the output channel from linear probe (class_num)
|
16 |
+
act: torch.nn.functional, the activation function before the loss function
|
17 |
+
"""
|
18 |
+
super().__init__()
|
19 |
+
in_ch = 512
|
20 |
+
self.clap_model = model
|
21 |
+
self.clap_model.text_branch = None # to save memory
|
22 |
+
self.freeze = freeze
|
23 |
+
if mlp:
|
24 |
+
self.lp_layer = MLPLayers(units=[in_ch, in_ch * 2, out_ch])
|
25 |
+
else:
|
26 |
+
self.lp_layer = nn.Linear(in_ch, out_ch)
|
27 |
+
|
28 |
+
if self.freeze:
|
29 |
+
for param in self.clap_model.parameters():
|
30 |
+
param.requires_grad = False
|
31 |
+
|
32 |
+
if act == "None":
|
33 |
+
self.act = None
|
34 |
+
elif act == "relu":
|
35 |
+
self.act = nn.ReLU()
|
36 |
+
elif act == "elu":
|
37 |
+
self.act = nn.ELU()
|
38 |
+
elif act == "prelu":
|
39 |
+
self.act = nn.PReLU(num_parameters=in_ch)
|
40 |
+
elif act == "softmax":
|
41 |
+
self.act = nn.Softmax(dim=-1)
|
42 |
+
elif act == "sigmoid":
|
43 |
+
self.act = nn.Sigmoid()
|
44 |
+
|
45 |
+
def forward(self, x, mix_lambda=None, device=None):
|
46 |
+
"""
|
47 |
+
Args:
|
48 |
+
x: waveform, torch.tensor [batch, t_samples] / batch of mel_spec and longer list
|
49 |
+
mix_lambda: torch.tensor [batch], the mixup lambda
|
50 |
+
Returns:
|
51 |
+
class_prob: torch.tensor [batch, class_num]
|
52 |
+
|
53 |
+
"""
|
54 |
+
# batchnorm cancel grandient
|
55 |
+
if self.freeze:
|
56 |
+
self.clap_model.eval()
|
57 |
+
|
58 |
+
x = self.clap_model.audio_projection(
|
59 |
+
self.clap_model.audio_branch(x, mixup_lambda=mix_lambda, device=device)[
|
60 |
+
"embedding"
|
61 |
+
]
|
62 |
+
)
|
63 |
+
out = self.lp_layer(x)
|
64 |
+
if self.act is not None:
|
65 |
+
out = self.act(out)
|
66 |
+
return out
|
src/audioldm/clap/open_clip/loss.py
ADDED
@@ -0,0 +1,398 @@
|
|
|
|
|
|
|
|
|
|
|
|
|
|
|
|
|
|
|
|
|
|
|
|
|
|
|
|
|
|
|
|
|
|
|
|
|
|
|
|
|
|
|
|
|
|
|
|
|
|
|
|
|
|
|
|
|
|
|
|
|
|
|
|
|
|
|
|
|
|
|
|
|
|
|
|
|
|
|
|
|
|
|
|
|
|
|
|
|
|
|
|
|
|
|
|
|
|
|
|
|
|
|
|
|
|
|
|
|
|
|
|
|
|
|
|
|
|
|
|
|
|
|
|
|
|
|
|
|
|
|
|
|
|
|
|
|
|
|
|
|
|
|
|
|
|
|
|
|
|
|
|
|
|
|
|
|
|
|
|
|
|
|
|
|
|
|
|
|
|
|
|
|
|
|
|
|
|
|
|
|
|
|
|
|
|
|
|
|
|
|
|
|
|
|
|
|
|
|
|
|
|
|
|
|
|
|
|
|
|
|
|
|
|
|
|
|
|
|
|
|
|
|
|
|
|
|
|
|
|
|
|
|
|
|
|
|
|
|
|
|
|
|
|
|
|
|
|
|
|
|
|
|
|
|
|
|
|
|
|
|
|
|
|
|
|
|
|
|
|
|
|
|
|
|
|
|
|
|
|
|
|
|
|
|
|
|
|
|
|
|
|
|
|
|
|
|
|
|
|
|
|
|
|
|
|
|
|
|
|
|
|
|
|
|
|
|
|
|
|
|
|
|
|
|
|
|
|
|
|
|
|
|
|
|
|
|
|
|
|
|
|
|
|
|
|
|
|
|
|
|
|
|
|
|
|
|
|
|
|
|
|
|
|
|
|
|
|
|
|
|
|
|
|
|
|
|
|
|
|
|
|
|
|
|
|
|
|
|
|
|
|
|
|
|
|
|
|
|
|
|
|
|
|
|
|
|
|
|
|
|
|
|
|
|
|
|
|
|
|
|
|
|
|
|
|
|
|
|
|
|
|
|
|
|
|
|
|
|
|
|
|
|
|
|
|
|
|
|
|
|
|
|
|
|
|
|
|
|
|
|
|
|
|
|
|
|
|
|
|
|
|
|
|
|
|
|
|
|
|
|
|
|
|
|
|
|
|
|
|
|
|
|
|
|
|
|
|
|
|
|
|
|
|
|
|
|
|
|
|
|
|
|
|
|
|
|
|
|
|
|
|
|
|
|
|
|
|
|
|
|
|
|
|
|
|
|
|
|
|
|
|
|
|
|
|
|
|
|
|
|
|
|
|
|
|
|
|
|
|
|
|
|
|
|
|
|
|
|
|
|
|
|
|
|
|
|
|
|
|
|
|
|
|
|
|
|
|
|
|
|
|
|
|
|
|
|
|
|
|
|
|
|
|
|
|
|
|
|
|
|
|
|
|
|
|
|
|
|
|
|
|
|
|
|
|
|
|
|
|
|
|
|
|
|
|
|
|
|
|
|
|
|
|
|
|
|
|
|
|
|
|
|
|
|
|
|
|
|
|
|
|
|
|
|
|
|
|
|
|
|
|
|
|
|
|
|
|
|
|
|
|
|
|
|
|
|
|
|
|
|
|
|
|
|
|
|
|
|
|
|
|
|
|
|
|
|
|
|
|
|
|
|
|
|
|
|
|
|
|
|
|
|
|
|
|
|
|
|
|
|
|
|
|
|
|
|
|
|
|
|
|
|
|
|
|
|
|
|
|
|
|
|
|
|
|
|
|
|
|
|
|
|
|
|
|
|
|
|
|
|
|
|
|
|
|
|
|
|
|
|
|
|
|
|
|
|
|
|
|
|
|
|
|
|
|
|
|
|
1 |
+
from multiprocessing.sharedctypes import Value
|
2 |
+
import torch
|
3 |
+
import torch.distributed.nn
|
4 |
+
from torch import distributed as dist, nn as nn
|
5 |
+
from torch.nn import functional as F
|
6 |
+
import numpy as np
|
7 |
+
from sklearn.metrics import average_precision_score, roc_auc_score, accuracy_score
|
8 |
+
|
9 |
+
try:
|
10 |
+
import horovod.torch as hvd
|
11 |
+
except ImportError:
|
12 |
+
hvd = None
|
13 |
+
|
14 |
+
|
15 |
+
def gather_features(
|
16 |
+
audio_features,
|
17 |
+
text_features,
|
18 |
+
audio_features_mlp=None,
|
19 |
+
text_features_mlp=None,
|
20 |
+
local_loss=False,
|
21 |
+
gather_with_grad=False,
|
22 |
+
rank=0,
|
23 |
+
world_size=1,
|
24 |
+
use_horovod=False,
|
25 |
+
mlp_loss=False,
|
26 |
+
):
|
27 |
+
if use_horovod:
|
28 |
+
assert hvd is not None, "Please install horovod"
|
29 |
+
if gather_with_grad:
|
30 |
+
all_audio_features = hvd.allgather(audio_features)
|
31 |
+
all_text_features = hvd.allgather(text_features)
|
32 |
+
if mlp_loss:
|
33 |
+
all_audio_features_mlp = hvd.allgather(audio_features_mlp)
|
34 |
+
all_text_features_mlp = hvd.allgather(text_features_mlp)
|
35 |
+
else:
|
36 |
+
with torch.no_grad():
|
37 |
+
all_audio_features = hvd.allgather(audio_features)
|
38 |
+
all_text_features = hvd.allgather(text_features)
|
39 |
+
if mlp_loss:
|
40 |
+
all_audio_features_mlp = hvd.allgather(audio_features_mlp)
|
41 |
+
all_text_features_mlp = hvd.allgather(text_features_mlp)
|
42 |
+
if not local_loss:
|
43 |
+
# ensure grads for local rank when all_* features don't have a gradient
|
44 |
+
gathered_audio_features = list(
|
45 |
+
all_audio_features.chunk(world_size, dim=0)
|
46 |
+
)
|
47 |
+
gathered_text_features = list(
|
48 |
+
all_text_features.chunk(world_size, dim=0)
|
49 |
+
)
|
50 |
+
gathered_audio_features[rank] = audio_features
|
51 |
+
gathered_text_features[rank] = text_features
|
52 |
+
all_audio_features = torch.cat(gathered_audio_features, dim=0)
|
53 |
+
all_text_features = torch.cat(gathered_text_features, dim=0)
|
54 |
+
if mlp_loss:
|
55 |
+
gathered_audio_features_mlp = list(
|
56 |
+
all_audio_features_mlp.chunk(world_size, dim=0)
|
57 |
+
)
|
58 |
+
gathered_text_features_mlp = list(
|
59 |
+
all_text_features_mlp.chunk(world_size, dim=0)
|
60 |
+
)
|
61 |
+
gathered_audio_features_mlp[rank] = audio_features_mlp
|
62 |
+
gathered_text_features_mlp[rank] = text_features_mlp
|
63 |
+
all_audio_features_mlp = torch.cat(
|
64 |
+
gathered_audio_features_mlp, dim=0
|
65 |
+
)
|
66 |
+
all_text_features_mlp = torch.cat(gathered_text_features_mlp, dim=0)
|
67 |
+
else:
|
68 |
+
# We gather tensors from all gpus
|
69 |
+
if gather_with_grad:
|
70 |
+
all_audio_features = torch.cat(
|
71 |
+
torch.distributed.nn.all_gather(audio_features), dim=0
|
72 |
+
)
|
73 |
+
all_text_features = torch.cat(
|
74 |
+
torch.distributed.nn.all_gather(text_features), dim=0
|
75 |
+
)
|
76 |
+
if mlp_loss:
|
77 |
+
all_audio_features_mlp = torch.cat(
|
78 |
+
torch.distributed.nn.all_gather(audio_features_mlp), dim=0
|
79 |
+
)
|
80 |
+
all_text_features_mlp = torch.cat(
|
81 |
+
torch.distributed.nn.all_gather(text_features_mlp), dim=0
|
82 |
+
)
|
83 |
+
else:
|
84 |
+
gathered_audio_features = [
|
85 |
+
torch.zeros_like(audio_features) for _ in range(world_size)
|
86 |
+
]
|
87 |
+
gathered_text_features = [
|
88 |
+
torch.zeros_like(text_features) for _ in range(world_size)
|
89 |
+
]
|
90 |
+
dist.all_gather(gathered_audio_features, audio_features)
|
91 |
+
dist.all_gather(gathered_text_features, text_features)
|
92 |
+
if mlp_loss:
|
93 |
+
gathered_audio_features_mlp = [
|
94 |
+
torch.zeros_like(audio_features_mlp) for _ in range(world_size)
|
95 |
+
]
|
96 |
+
gathered_text_features_mlp = [
|
97 |
+
torch.zeros_like(text_features_mlp) for _ in range(world_size)
|
98 |
+
]
|
99 |
+
dist.all_gather(gathered_audio_features_mlp, audio_features_mlp)
|
100 |
+
dist.all_gather(gathered_text_features_mlp, text_features_mlp)
|
101 |
+
if not local_loss:
|
102 |
+
# ensure grads for local rank when all_* features don't have a gradient
|
103 |
+
gathered_audio_features[rank] = audio_features
|
104 |
+
gathered_text_features[rank] = text_features
|
105 |
+
if mlp_loss:
|
106 |
+
gathered_audio_features_mlp[rank] = audio_features_mlp
|
107 |
+
gathered_text_features_mlp[rank] = text_features_mlp
|
108 |
+
|
109 |
+
all_audio_features = torch.cat(gathered_audio_features, dim=0)
|
110 |
+
all_text_features = torch.cat(gathered_text_features, dim=0)
|
111 |
+
if mlp_loss:
|
112 |
+
all_audio_features_mlp = torch.cat(gathered_audio_features_mlp, dim=0)
|
113 |
+
all_text_features_mlp = torch.cat(gathered_text_features_mlp, dim=0)
|
114 |
+
if mlp_loss:
|
115 |
+
return (
|
116 |
+
all_audio_features,
|
117 |
+
all_text_features,
|
118 |
+
all_audio_features_mlp,
|
119 |
+
all_text_features_mlp,
|
120 |
+
)
|
121 |
+
else:
|
122 |
+
return all_audio_features, all_text_features
|
123 |
+
|
124 |
+
|
125 |
+
class ClipLoss(nn.Module):
|
126 |
+
def __init__(
|
127 |
+
self,
|
128 |
+
local_loss=False,
|
129 |
+
gather_with_grad=False,
|
130 |
+
cache_labels=False,
|
131 |
+
rank=0,
|
132 |
+
world_size=1,
|
133 |
+
use_horovod=False,
|
134 |
+
mlp_loss=False,
|
135 |
+
weight_loss_kappa=0,
|
136 |
+
):
|
137 |
+
super().__init__()
|
138 |
+
self.local_loss = local_loss
|
139 |
+
self.gather_with_grad = gather_with_grad
|
140 |
+
self.cache_labels = cache_labels
|
141 |
+
self.rank = rank
|
142 |
+
self.world_size = world_size
|
143 |
+
self.use_horovod = use_horovod
|
144 |
+
self.mlp_loss = mlp_loss
|
145 |
+
self.weighted_loss = bool(weight_loss_kappa != 0)
|
146 |
+
self.weight_loss_kappa = weight_loss_kappa
|
147 |
+
# cache state
|
148 |
+
self.prev_num_logits = 0
|
149 |
+
self.labels = {}
|
150 |
+
|
151 |
+
def forward(
|
152 |
+
self,
|
153 |
+
audio_features,
|
154 |
+
text_features,
|
155 |
+
logit_scale_a,
|
156 |
+
logit_scale_t=None,
|
157 |
+
audio_features_mlp=None,
|
158 |
+
text_features_mlp=None,
|
159 |
+
):
|
160 |
+
device = audio_features.device
|
161 |
+
if self.mlp_loss:
|
162 |
+
if self.world_size > 1:
|
163 |
+
(
|
164 |
+
all_audio_features,
|
165 |
+
all_text_features,
|
166 |
+
all_audio_features_mlp,
|
167 |
+
all_text_features_mlp,
|
168 |
+
) = gather_features(
|
169 |
+
audio_features=audio_features,
|
170 |
+
text_features=text_features,
|
171 |
+
audio_features_mlp=audio_features_mlp,
|
172 |
+
text_features_mlp=text_features_mlp,
|
173 |
+
local_loss=self.local_loss,
|
174 |
+
gather_with_grad=self.gather_with_grad,
|
175 |
+
rank=self.rank,
|
176 |
+
world_size=self.world_size,
|
177 |
+
use_horovod=self.use_horovod,
|
178 |
+
mlp_loss=self.mlp_loss,
|
179 |
+
)
|
180 |
+
if self.local_loss:
|
181 |
+
a_logits_per_audio = (
|
182 |
+
logit_scale_a * audio_features @ all_text_features_mlp.T
|
183 |
+
)
|
184 |
+
a_logits_per_text = (
|
185 |
+
logit_scale_a * text_features_mlp @ all_audio_features.T
|
186 |
+
)
|
187 |
+
t_logits_per_audio = (
|
188 |
+
logit_scale_t * audio_features_mlp @ all_text_features.T
|
189 |
+
)
|
190 |
+
t_logits_per_text = (
|
191 |
+
logit_scale_t * text_features @ all_audio_features_mlp.T
|
192 |
+
)
|
193 |
+
else:
|
194 |
+
a_logits_per_audio = (
|
195 |
+
logit_scale_a * all_audio_features @ all_text_features_mlp.T
|
196 |
+
)
|
197 |
+
a_logits_per_text = a_logits_per_audio.T
|
198 |
+
t_logits_per_audio = (
|
199 |
+
logit_scale_t * all_audio_features_mlp @ all_text_features.T
|
200 |
+
)
|
201 |
+
t_logits_per_text = t_logits_per_audio.T
|
202 |
+
else:
|
203 |
+
a_logits_per_audio = (
|
204 |
+
logit_scale_a * audio_features @ text_features_mlp.T
|
205 |
+
)
|
206 |
+
a_logits_per_text = logit_scale_a * text_features_mlp @ audio_features.T
|
207 |
+
t_logits_per_audio = (
|
208 |
+
logit_scale_t * audio_features_mlp @ text_features.T
|
209 |
+
)
|
210 |
+
t_logits_per_text = logit_scale_t * text_features @ audio_features_mlp.T
|
211 |
+
|
212 |
+
# calculated ground-truth and cache if enabled
|
213 |
+
num_logits = a_logits_per_audio.shape[0]
|
214 |
+
if self.prev_num_logits != num_logits or device not in self.labels:
|
215 |
+
labels = torch.arange(num_logits, device=device, dtype=torch.long)
|
216 |
+
if self.world_size > 1 and self.local_loss:
|
217 |
+
labels = labels + num_logits * self.rank
|
218 |
+
if self.cache_labels:
|
219 |
+
self.labels[device] = labels
|
220 |
+
self.prev_num_logits = num_logits
|
221 |
+
else:
|
222 |
+
labels = self.labels[device]
|
223 |
+
|
224 |
+
if not self.weighted_loss:
|
225 |
+
total_loss = (
|
226 |
+
F.cross_entropy(a_logits_per_audio, labels)
|
227 |
+
+ F.cross_entropy(a_logits_per_text, labels)
|
228 |
+
+ F.cross_entropy(t_logits_per_audio, labels)
|
229 |
+
+ F.cross_entropy(t_logits_per_text, labels)
|
230 |
+
) / 4
|
231 |
+
else:
|
232 |
+
audio_weight = (audio_features @ audio_features.T).detach()
|
233 |
+
audio_weight = (
|
234 |
+
torch.exp(
|
235 |
+
torch.sum(audio_weight, axis=1)
|
236 |
+
/ (self.weight_loss_kappa * len(audio_weight))
|
237 |
+
)
|
238 |
+
).detach()
|
239 |
+
text_weight = (text_features @ text_features.T).detach()
|
240 |
+
text_weight = (
|
241 |
+
torch.exp(
|
242 |
+
torch.sum(text_weight, axis=1)
|
243 |
+
/ (self.weight_loss_kappa * len(text_features))
|
244 |
+
)
|
245 |
+
).detach()
|
246 |
+
total_loss = (
|
247 |
+
F.cross_entropy(a_logits_per_audio, labels, weight=audio_weight)
|
248 |
+
+ F.cross_entropy(a_logits_per_text, labels, weight=audio_weight)
|
249 |
+
+ F.cross_entropy(t_logits_per_audio, labels, weight=text_weight)
|
250 |
+
+ F.cross_entropy(t_logits_per_text, labels, weight=text_weight)
|
251 |
+
) / 4
|
252 |
+
else:
|
253 |
+
if self.world_size > 1:
|
254 |
+
all_audio_features, all_text_features = gather_features(
|
255 |
+
audio_features=audio_features,
|
256 |
+
text_features=text_features,
|
257 |
+
local_loss=self.local_loss,
|
258 |
+
gather_with_grad=self.gather_with_grad,
|
259 |
+
rank=self.rank,
|
260 |
+
world_size=self.world_size,
|
261 |
+
use_horovod=self.use_horovod,
|
262 |
+
mlp_loss=self.mlp_loss,
|
263 |
+
)
|
264 |
+
|
265 |
+
if self.local_loss:
|
266 |
+
logits_per_audio = (
|
267 |
+
logit_scale_a * audio_features @ all_text_features.T
|
268 |
+
)
|
269 |
+
logits_per_text = (
|
270 |
+
logit_scale_a * text_features @ all_audio_features.T
|
271 |
+
)
|
272 |
+
else:
|
273 |
+
logits_per_audio = (
|
274 |
+
logit_scale_a * all_audio_features @ all_text_features.T
|
275 |
+
)
|
276 |
+
logits_per_text = logits_per_audio.T
|
277 |
+
else:
|
278 |
+
logits_per_audio = logit_scale_a * audio_features @ text_features.T
|
279 |
+
logits_per_text = logit_scale_a * text_features @ audio_features.T
|
280 |
+
|
281 |
+
# calculated ground-truth and cache if enabled
|
282 |
+
num_logits = logits_per_audio.shape[0]
|
283 |
+
if self.prev_num_logits != num_logits or device not in self.labels:
|
284 |
+
labels = torch.arange(num_logits, device=device, dtype=torch.long)
|
285 |
+
if self.world_size > 1 and self.local_loss:
|
286 |
+
labels = labels + num_logits * self.rank
|
287 |
+
if self.cache_labels:
|
288 |
+
self.labels[device] = labels
|
289 |
+
self.prev_num_logits = num_logits
|
290 |
+
else:
|
291 |
+
labels = self.labels[device]
|
292 |
+
if not self.weighted_loss:
|
293 |
+
total_loss = (
|
294 |
+
F.cross_entropy(logits_per_audio, labels)
|
295 |
+
+ F.cross_entropy(logits_per_text, labels)
|
296 |
+
) / 2
|
297 |
+
else:
|
298 |
+
audio_weight = (all_audio_features @ all_audio_features.T).detach()
|
299 |
+
audio_weight = (
|
300 |
+
torch.exp(
|
301 |
+
torch.sum(audio_weight, axis=1)
|
302 |
+
/ (self.weight_loss_kappa * len(all_audio_features))
|
303 |
+
)
|
304 |
+
).detach()
|
305 |
+
text_weight = (all_text_features @ all_text_features.T).detach()
|
306 |
+
text_weight = (
|
307 |
+
torch.exp(
|
308 |
+
torch.sum(text_weight, axis=1)
|
309 |
+
/ (self.weight_loss_kappa * len(all_text_features))
|
310 |
+
)
|
311 |
+
).detach()
|
312 |
+
total_loss = (
|
313 |
+
F.cross_entropy(logits_per_audio, labels, weight=text_weight)
|
314 |
+
+ F.cross_entropy(logits_per_text, labels, weight=audio_weight)
|
315 |
+
) / 2
|
316 |
+
return total_loss
|
317 |
+
|
318 |
+
|
319 |
+
def lp_gather_features(pred, target, world_size=1, use_horovod=False):
|
320 |
+
if use_horovod:
|
321 |
+
assert hvd is not None, "Please install horovod"
|
322 |
+
with torch.no_grad():
|
323 |
+
all_preds = hvd.allgather(pred)
|
324 |
+
all_targets = hvd.allgath(target)
|
325 |
+
else:
|
326 |
+
gathered_preds = [torch.zeros_like(pred) for _ in range(world_size)]
|
327 |
+
gathered_targets = [torch.zeros_like(target) for _ in range(world_size)]
|
328 |
+
|
329 |
+
dist.all_gather(gathered_preds, pred)
|
330 |
+
dist.all_gather(gathered_targets, target)
|
331 |
+
all_preds = torch.cat(gathered_preds, dim=0)
|
332 |
+
all_targets = torch.cat(gathered_targets, dim=0)
|
333 |
+
|
334 |
+
return all_preds, all_targets
|
335 |
+
|
336 |
+
|
337 |
+
def get_map(pred, target):
|
338 |
+
pred = torch.sigmoid(pred).numpy()
|
339 |
+
target = target.numpy()
|
340 |
+
return np.mean(average_precision_score(target, pred, average=None))
|
341 |
+
|
342 |
+
|
343 |
+
def get_acc(pred, target):
|
344 |
+
pred = torch.argmax(pred, 1).numpy()
|
345 |
+
target = torch.argmax(target, 1).numpy()
|
346 |
+
return accuracy_score(target, pred)
|
347 |
+
|
348 |
+
|
349 |
+
def get_mauc(pred, target):
|
350 |
+
pred = torch.sigmoid(pred).numpy()
|
351 |
+
target = target.numpy()
|
352 |
+
return np.mean(roc_auc_score(target, pred, average=None))
|
353 |
+
|
354 |
+
|
355 |
+
class LPMetrics(object):
|
356 |
+
def __init__(self, metric_names=["map", "acc", "mauc"]):
|
357 |
+
self.metrics = []
|
358 |
+
for name in metric_names:
|
359 |
+
self.metrics.append(self.get_metric(name))
|
360 |
+
self.metric_names = metric_names
|
361 |
+
|
362 |
+
def get_metric(self, name):
|
363 |
+
if name == "map":
|
364 |
+
return get_map
|
365 |
+
elif name == "acc":
|
366 |
+
return get_acc
|
367 |
+
elif name == "mauc":
|
368 |
+
return get_mauc
|
369 |
+
else:
|
370 |
+
raise ValueError(f"the metric should be at least one of [map, acc, mauc]")
|
371 |
+
|
372 |
+
def evaluate_mertics(self, pred, target):
|
373 |
+
metric_dict = {}
|
374 |
+
for i in range(len(self.metric_names)):
|
375 |
+
metric_dict[self.metric_names[i]] = self.metrics[i](pred, target)
|
376 |
+
return metric_dict
|
377 |
+
|
378 |
+
|
379 |
+
def calc_celoss(pred, target):
|
380 |
+
target = torch.argmax(target, 1).long()
|
381 |
+
return nn.CrossEntropyLoss()(pred, target)
|
382 |
+
|
383 |
+
|
384 |
+
class LPLoss(nn.Module):
|
385 |
+
def __init__(self, loss_name):
|
386 |
+
super().__init__()
|
387 |
+
if loss_name == "bce":
|
388 |
+
self.loss_func = nn.BCEWithLogitsLoss()
|
389 |
+
elif loss_name == "ce":
|
390 |
+
self.loss_func = calc_celoss
|
391 |
+
elif loss_name == "mse":
|
392 |
+
self.loss_func = nn.MSELoss()
|
393 |
+
else:
|
394 |
+
raise ValueError(f"the loss func should be at least one of [bce, ce, mse]")
|
395 |
+
|
396 |
+
def forward(self, pred, target):
|
397 |
+
loss = self.loss_func(pred, target)
|
398 |
+
return loss
|
src/audioldm/clap/open_clip/model.py
ADDED
@@ -0,0 +1,936 @@
|
|
|
|
|
|
|
|
|
|
|
|
|
|
|
|
|
|
|
|
|
|
|
|
|
|
|
|
|
|
|
|
|
|
|
|
|
|
|
|
|
|
|
|
|
|
|
|
|
|
|
|
|
|
|
|
|
|
|
|
|
|
|
|
|
|
|
|
|
|
|
|
|
|
|
|
|
|
|
|
|
|
|
|
|
|
|
|
|
|
|
|
|
|
|
|
|
|
|
|
|
|
|
|
|
|
|
|
|
|
|
|
|
|
|
|
|
|
|
|
|
|
|
|
|
|
|
|
|
|
|
|
|
|
|
|
|
|
|
|
|
|
|
|
|
|
|
|
|
|
|
|
|
|
|
|
|
|
|
|
|
|
|
|
|
|
|
|
|
|
|
|
|
|
|
|
|
|
|
|
|
|
|
|
|
|
|
|
|
|
|
|
|
|
|
|
|
|
|
|
|
|
|
|
|
|
|
|
|
|
|
|
|
|
|
|
|
|
|
|
|
|
|
|
|
|
|
|
|
|
|
|
|
|
|
|
|
|
|
|
|
|
|
|
|
|
|
|
|
|
|
|
|
|
|
|
|
|
|
|
|
|
|
|
|
|
|
|
|
|
|
|
|
|
|
|
|
|
|
|
|
|
|
|
|
|
|
|
|
|
|
|
|
|
|
|
|
|
|
|
|
|
|
|
|
|
|
|
|
|
|
|
|
|
|
|
|
|
|
|
|
|
|
|
|
|
|
|
|
|
|
|
|
|
|
|
|
|
|
|
|
|
|
|
|
|
|
|
|
|
|
|
|
|
|
|
|
|
|
|
|
|
|
|
|
|
|
|
|
|
|
|
|
|
|
|
|
|
|
|
|
|
|
|
|
|
|
|
|
|
|
|
|
|
|
|
|
|
|
|
|
|
|
|
|
|
|
|
|
|
|
|
|
|
|
|
|
|
|
|
|
|
|
|
|
|
|
|
|
|
|
|
|
|
|
|
|
|
|
|
|
|
|
|
|
|
|
|
|
|
|
|
|
|
|
|
|
|
|
|
|
|
|
|
|
|
|
|
|
|
|
|
|
|
|
|
|
|
|
|
|
|
|
|
|
|
|
|
|
|
|
|
|
|
|
|
|
|
|
|
|
|
|
|
|
|
|
|
|
|
|
|
|
|
|
|
|
|
|
|
|
|
|
|
|
|
|
|
|
|
|
|
|
|
|
|
|
|
|
|
|
|
|
|
|
|
|
|
|
|
|
|
|
|
|
|
|
|
|
|
|
|
|
|
|
|
|
|
|
|
|
|
|
|
|
|
|
|
|
|
|
|
|
|
|
|
|
|
|
|
|
|
|
|
|
|
|
|
|
|
|
|
|
|
|
|
|
|
|
|
|
|
|
|
|
|
|
|
|
|
|
|
|
|
|
|
|
|
|
|
|
|
|
|
|
|
|
|
|
|
|
|
|
|
|
|
|
|
|
|
|
|
|
|
|
|
|
|
|
|
|
|
|
|
|
|
|
|
|
|
|
|
|
|
|
|
|
|
|
|
|
|
|
|
|
|
|
|
|
|
|
|
|
|
|
|
|
|
|
|
|
|
|
|
|
|
|
|
|
|
|
|
|
|
|
|
|
|
|
|
|
|
|
|
|
|
|
|
|
|
|
|
|
|
|
|
|
|
|
|
|
|
|
|
|
|
|
|
|
|
|
|
|
|
|
|
|
|
|
|
|
|
|
|
|
|
|
|
|
|
|
|
|
|
|
|
|
|
|
|
|
|
|
|
|
|
|
|
|
|
|
|
|
|
|
|
|
|
|
|
|
|
|
|
|
|
|
|
|
|
|
|
|
|
|
|
|
|
|
|
|
|
|
|
|
|
|
|
|
|
|
|
|
|
|
|
|
|
|
|
|
|
|
|
|
|
|
|
|
|
|
|
|
|
|
|
|
|
|
|
|
|
|
|
|
|
|
|
|
|
|
|
|
|
|
|
|
|
|
|
|
|
|
|
|
|
|
|
|
|
|
|
|
|
|
|
|
|
|
|
|
|
|
|
|
|
|
|
|
|
|
|
|
|
|
|
|
|
|
|
|
|
|
|
|
|
|
|
|
|
|
|
|
|
|
|
|
|
|
|
|
|
|
|
|
|
|
|
|
|
|
|
|
|
|
|
|
|
|
|
|
|
|
|
|
|
|
|
|
|
|
|
|
|
|
|
|
|
|
|
|
|
|
|
|
|
|
|
|
|
|
|
|
|
|
|
|
|
|
|
|
|
|
|
|
|
|
|
|
|
|
|
|
|
|
|
|
|
|
|
|
|
|
|
|
|
|
|
|
|
|
|
|
|
|
|
|
|
|
|
|
|
|
|
|
|
|
|
|
|
|
|
|
|
|
|
|
|
|
|
|
|
|
|
|
|
|
|
|
|
|
|
|
|
|
|
|
|
|
|
|
|
|
|
|
|
|
|
|
|
|
|
|
|
|
|
|
|
|
|
|
|
|
|
|
|
|
|
|
|
|
|
|
|
|
|
|
|
|
|
|
|
|
|
|
|
|
|
|
|
|
|
|
|
|
|
|
|
|
|
|
|
|
|
|
|
|
|
|
|
|
|
|
|
|
|
|
|
|
|
|
|
|
|
|
|
|
|
|
|
|
|
|
|
|
|
|
|
|
|
|
|
|
|
|
|
|
|
|
|
|
|
|
|
|
|
|
|
|
|
|
|
|
|
|
|
|
|
|
|
|
|
|
|
|
|
|
|
|
|
|
|
|
|
|
|
|
|
|
|
|
|
|
|
|
|
|
|
|
|
|
|
|
|
|
|
|
|
|
|
|
|
|
|
|
|
|
|
|
|
|
|
|
|
|
|
|
|
|
|
|
|
|
|
|
|
|
|
|
|
|
|
|
|
|
|
|
|
|
|
|
|
|
|
|
|
|
|
|
|
|
|
|
|
|
|
|
|
|
|
|
|
|
|
|
|
|
|
|
|
|
|
|
|
|
|
|
|
|
|
|
|
|
|
|
|
|
|
|
|
|
|
|
|
|
|
|
|
|
|
|
|
|
|
|
|
|
|
|
|
|
|
|
|
|
|
|
|
|
|
|
|
|
|
|
|
|
|
|
|
|
|
|
|
|
|
|
|
|
|
|
|
|
|
|
|
|
|
|
|
|
|
|
|
|
|
|
|
|
|
|
|
|
|
|
|
|
|
|
|
|
|
|
|
|
|
|
|
|
|
|
|
|
|
|
|
|
|
|
|
|
|
|
|
|
|
|
|
|
|
|
|
|
|
|
|
|
|
|
|
|
|
|
|
|
|
|
|
|
|
|
|
|
|
|
|
|
|
|
|
|
|
|
|
|
|
|
|
|
|
|
|
|
|
|
|
|
|
|
|
|
|
|
|
|
|
|
|
|
|
|
|
|
|
|
|
|
|
|
|
|
|
|
|
|
|
|
|
|
|
|
|
|
|
|
|
|
|
|
|
|
|
|
|
|
|
|
|
|
|
|
|
|
|
|
|
|
|
|
|
|
|
|
|
|
|
|
|
|
|
|
|
|
|
|
|
|
|
|
|
|
|
|
|
|
|
|
|
|
|
|
|
|
|
|
|
|
|
|
|
|
|
|
|
|
|
|
|
|
|
|
|
|
|
|
|
|
|
|
|
|
|
|
|
|
|
|
|
|
|
|
|
|
|
|
|
|
|
|
|
|
|
|
|
|
|
|
|
|
|
|
|
|
|
|
|
|
|
|
|
|
|
|
|
|
|
|
|
|
|
|
|
|
|
|
|
|
|
|
|
|
|
|
|
|
|
|
|
|
|
|
|
|
|
|
|
|
|
|
|
|
|
|
|
|
|
|
|
|
|
|
|
|
|
|
|
|
|
|
|
|
|
|
|
|
|
|
|
|
|
|
|
|
|
|
|
|
|
|
|
|
|
|
|
|
|
|
|
|
|
|
|
|
|
|
|
|
|
|
|
|
|
|
|
|
|
|
|
|
|
|
|
|
|
|
|
|
|
|
|
|
|
|
|
|
|
|
|
|
|
|
|
|
|
|
|
|
|
|
|
|
|
|
|
|
|
|
|
|
|
|
|
|
|
|
|
|
|
|
|
|
|
|
|
|
|
|
|
|
|
|
|
|
|
|
|
|
|
|
|
|
|
|
|
|
|
|
|
|
|
|
|
|
|
|
|
|
|
|
|
|
|
|
|
|
|
|
|
|
|
|
|
|
|
|
1 |
+
""" CLAP Model
|
2 |
+
|
3 |
+
Adapted from CLIP: https://github.com/openai/CLIP. Originally MIT License, Copyright (c) 2021 OpenAI.
|
4 |
+
Adapted to the Audio Task.
|
5 |
+
"""
|
6 |
+
|
7 |
+
from collections import OrderedDict
|
8 |
+
from dataclasses import dataclass
|
9 |
+
from email.mime import audio
|
10 |
+
from typing import Tuple, Union, Callable, Optional
|
11 |
+
|
12 |
+
import numpy as np
|
13 |
+
import torch
|
14 |
+
import torch.nn.functional as F
|
15 |
+
from torch import nn
|
16 |
+
|
17 |
+
from .timm_model import TimmModel
|
18 |
+
import logging
|
19 |
+
from .utils import freeze_batch_norm_2d
|
20 |
+
|
21 |
+
from .pann_model import create_pann_model
|
22 |
+
from .htsat import create_htsat_model
|
23 |
+
from transformers import BertModel, RobertaModel, BartModel
|
24 |
+
from transformers.tokenization_utils_base import BatchEncoding
|
25 |
+
|
26 |
+
|
27 |
+
class MLPLayers(nn.Module):
|
28 |
+
def __init__(self, units=[512, 512, 512], nonlin=nn.ReLU(), dropout=0.1):
|
29 |
+
super(MLPLayers, self).__init__()
|
30 |
+
self.nonlin = nonlin
|
31 |
+
self.dropout = dropout
|
32 |
+
|
33 |
+
sequence = []
|
34 |
+
for u0, u1 in zip(units[:-1], units[1:]):
|
35 |
+
sequence.append(nn.Linear(u0, u1))
|
36 |
+
sequence.append(self.nonlin)
|
37 |
+
sequence.append(nn.Dropout(self.dropout))
|
38 |
+
sequence = sequence[:-2]
|
39 |
+
|
40 |
+
self.sequential = nn.Sequential(*sequence)
|
41 |
+
|
42 |
+
def forward(self, X):
|
43 |
+
X = self.sequential(X)
|
44 |
+
return X
|
45 |
+
|
46 |
+
|
47 |
+
class Bottleneck(nn.Module):
|
48 |
+
expansion = 4
|
49 |
+
|
50 |
+
def __init__(self, inplanes, planes, stride=1):
|
51 |
+
super().__init__()
|
52 |
+
|
53 |
+
# all conv layers have stride 1. an avgpool is performed after the second convolution when stride > 1
|
54 |
+
self.conv1 = nn.Conv2d(inplanes, planes, 1, bias=False)
|
55 |
+
self.bn1 = nn.BatchNorm2d(planes)
|
56 |
+
|
57 |
+
self.conv2 = nn.Conv2d(planes, planes, 3, padding=1, bias=False)
|
58 |
+
self.bn2 = nn.BatchNorm2d(planes)
|
59 |
+
|
60 |
+
self.avgpool = nn.AvgPool2d(stride) if stride > 1 else nn.Identity()
|
61 |
+
|
62 |
+
self.conv3 = nn.Conv2d(planes, planes * self.expansion, 1, bias=False)
|
63 |
+
self.bn3 = nn.BatchNorm2d(planes * self.expansion)
|
64 |
+
|
65 |
+
self.relu = nn.ReLU(inplace=True)
|
66 |
+
self.downsample = None
|
67 |
+
self.stride = stride
|
68 |
+
|
69 |
+
if stride > 1 or inplanes != planes * Bottleneck.expansion:
|
70 |
+
# downsampling layer is prepended with an avgpool, and the subsequent convolution has stride 1
|
71 |
+
self.downsample = nn.Sequential(
|
72 |
+
OrderedDict(
|
73 |
+
[
|
74 |
+
("-1", nn.AvgPool2d(stride)),
|
75 |
+
(
|
76 |
+
"0",
|
77 |
+
nn.Conv2d(
|
78 |
+
inplanes,
|
79 |
+
planes * self.expansion,
|
80 |
+
1,
|
81 |
+
stride=1,
|
82 |
+
bias=False,
|
83 |
+
),
|
84 |
+
),
|
85 |
+
("1", nn.BatchNorm2d(planes * self.expansion)),
|
86 |
+
]
|
87 |
+
)
|
88 |
+
)
|
89 |
+
|
90 |
+
def forward(self, x: torch.Tensor):
|
91 |
+
identity = x
|
92 |
+
|
93 |
+
out = self.relu(self.bn1(self.conv1(x)))
|
94 |
+
out = self.relu(self.bn2(self.conv2(out)))
|
95 |
+
out = self.avgpool(out)
|
96 |
+
out = self.bn3(self.conv3(out))
|
97 |
+
|
98 |
+
if self.downsample is not None:
|
99 |
+
identity = self.downsample(x)
|
100 |
+
|
101 |
+
out += identity
|
102 |
+
out = self.relu(out)
|
103 |
+
return out
|
104 |
+
|
105 |
+
|
106 |
+
class AttentionPool2d(nn.Module):
|
107 |
+
def __init__(
|
108 |
+
self, spacial_dim: int, embed_dim: int, num_heads: int, output_dim: int = None
|
109 |
+
):
|
110 |
+
super().__init__()
|
111 |
+
self.positional_embedding = nn.Parameter(
|
112 |
+
torch.randn(spacial_dim**2 + 1, embed_dim) / embed_dim**0.5
|
113 |
+
)
|
114 |
+
self.k_proj = nn.Linear(embed_dim, embed_dim)
|
115 |
+
self.q_proj = nn.Linear(embed_dim, embed_dim)
|
116 |
+
self.v_proj = nn.Linear(embed_dim, embed_dim)
|
117 |
+
self.c_proj = nn.Linear(embed_dim, output_dim or embed_dim)
|
118 |
+
self.num_heads = num_heads
|
119 |
+
|
120 |
+
def forward(self, x):
|
121 |
+
x = x.reshape(x.shape[0], x.shape[1], x.shape[2] * x.shape[3]).permute(
|
122 |
+
2, 0, 1
|
123 |
+
) # NCHW -> (HW)NC
|
124 |
+
x = torch.cat([x.mean(dim=0, keepdim=True), x], dim=0) # (HW+1)NC
|
125 |
+
x = x + self.positional_embedding[:, None, :].to(x.dtype) # (HW+1)NC
|
126 |
+
x, _ = F.multi_head_attention_forward(
|
127 |
+
query=x,
|
128 |
+
key=x,
|
129 |
+
value=x,
|
130 |
+
embed_dim_to_check=x.shape[-1],
|
131 |
+
num_heads=self.num_heads,
|
132 |
+
q_proj_weight=self.q_proj.weight,
|
133 |
+
k_proj_weight=self.k_proj.weight,
|
134 |
+
v_proj_weight=self.v_proj.weight,
|
135 |
+
in_proj_weight=None,
|
136 |
+
in_proj_bias=torch.cat(
|
137 |
+
[self.q_proj.bias, self.k_proj.bias, self.v_proj.bias]
|
138 |
+
),
|
139 |
+
bias_k=None,
|
140 |
+
bias_v=None,
|
141 |
+
add_zero_attn=False,
|
142 |
+
dropout_p=0,
|
143 |
+
out_proj_weight=self.c_proj.weight,
|
144 |
+
out_proj_bias=self.c_proj.bias,
|
145 |
+
use_separate_proj_weight=True,
|
146 |
+
training=self.training,
|
147 |
+
need_weights=False,
|
148 |
+
)
|
149 |
+
|
150 |
+
return x[0]
|
151 |
+
|
152 |
+
|
153 |
+
class ModifiedResNet(nn.Module):
|
154 |
+
"""
|
155 |
+
A ResNet class that is similar to torchvision's but contains the following changes:
|
156 |
+
- There are now 3 "stem" convolutions as opposed to 1, with an average pool instead of a max pool.
|
157 |
+
- Performs anti-aliasing strided convolutions, where an avgpool is prepended to convolutions with stride > 1
|
158 |
+
- The final pooling layer is a QKV attention instead of an average pool
|
159 |
+
"""
|
160 |
+
|
161 |
+
def __init__(self, layers, output_dim, heads, image_size=224, width=64):
|
162 |
+
super().__init__()
|
163 |
+
self.output_dim = output_dim
|
164 |
+
self.image_size = image_size
|
165 |
+
|
166 |
+
# the 3-layer stem
|
167 |
+
self.conv1 = nn.Conv2d(
|
168 |
+
3, width // 2, kernel_size=3, stride=2, padding=1, bias=False
|
169 |
+
)
|
170 |
+
self.bn1 = nn.BatchNorm2d(width // 2)
|
171 |
+
self.conv2 = nn.Conv2d(
|
172 |
+
width // 2, width // 2, kernel_size=3, padding=1, bias=False
|
173 |
+
)
|
174 |
+
self.bn2 = nn.BatchNorm2d(width // 2)
|
175 |
+
self.conv3 = nn.Conv2d(width // 2, width, kernel_size=3, padding=1, bias=False)
|
176 |
+
self.bn3 = nn.BatchNorm2d(width)
|
177 |
+
self.avgpool = nn.AvgPool2d(2)
|
178 |
+
self.relu = nn.ReLU(inplace=True)
|
179 |
+
|
180 |
+
# residual layers
|
181 |
+
self._inplanes = width # this is a *mutable* variable used during construction
|
182 |
+
self.layer1 = self._make_layer(width, layers[0])
|
183 |
+
self.layer2 = self._make_layer(width * 2, layers[1], stride=2)
|
184 |
+
self.layer3 = self._make_layer(width * 4, layers[2], stride=2)
|
185 |
+
self.layer4 = self._make_layer(width * 8, layers[3], stride=2)
|
186 |
+
|
187 |
+
embed_dim = width * 32 # the ResNet feature dimension
|
188 |
+
self.attnpool = AttentionPool2d(image_size // 32, embed_dim, heads, output_dim)
|
189 |
+
|
190 |
+
self.init_parameters()
|
191 |
+
|
192 |
+
def _make_layer(self, planes, blocks, stride=1):
|
193 |
+
layers = [Bottleneck(self._inplanes, planes, stride)]
|
194 |
+
|
195 |
+
self._inplanes = planes * Bottleneck.expansion
|
196 |
+
for _ in range(1, blocks):
|
197 |
+
layers.append(Bottleneck(self._inplanes, planes))
|
198 |
+
|
199 |
+
return nn.Sequential(*layers)
|
200 |
+
|
201 |
+
def init_parameters(self):
|
202 |
+
if self.attnpool is not None:
|
203 |
+
std = self.attnpool.c_proj.in_features**-0.5
|
204 |
+
nn.init.normal_(self.attnpool.q_proj.weight, std=std)
|
205 |
+
nn.init.normal_(self.attnpool.k_proj.weight, std=std)
|
206 |
+
nn.init.normal_(self.attnpool.v_proj.weight, std=std)
|
207 |
+
nn.init.normal_(self.attnpool.c_proj.weight, std=std)
|
208 |
+
|
209 |
+
for resnet_block in [self.layer1, self.layer2, self.layer3, self.layer4]:
|
210 |
+
for name, param in resnet_block.named_parameters():
|
211 |
+
if name.endswith("bn3.weight"):
|
212 |
+
nn.init.zeros_(param)
|
213 |
+
|
214 |
+
def lock(self, unlocked_groups=0, freeze_bn_stats=False):
|
215 |
+
assert (
|
216 |
+
unlocked_groups == 0
|
217 |
+
), "partial locking not currently supported for this model"
|
218 |
+
for param in self.parameters():
|
219 |
+
param.requires_grad = False
|
220 |
+
if freeze_bn_stats:
|
221 |
+
freeze_batch_norm_2d(self)
|
222 |
+
|
223 |
+
def stem(self, x):
|
224 |
+
for conv, bn in [
|
225 |
+
(self.conv1, self.bn1),
|
226 |
+
(self.conv2, self.bn2),
|
227 |
+
(self.conv3, self.bn3),
|
228 |
+
]:
|
229 |
+
x = self.relu(bn(conv(x)))
|
230 |
+
x = self.avgpool(x)
|
231 |
+
return x
|
232 |
+
|
233 |
+
def forward(self, x):
|
234 |
+
x = self.stem(x)
|
235 |
+
x = self.layer1(x)
|
236 |
+
x = self.layer2(x)
|
237 |
+
x = self.layer3(x)
|
238 |
+
x = self.layer4(x)
|
239 |
+
x = self.attnpool(x)
|
240 |
+
|
241 |
+
return x
|
242 |
+
|
243 |
+
|
244 |
+
class LayerNorm(nn.LayerNorm):
|
245 |
+
"""Subclass torch's LayerNorm to handle fp16."""
|
246 |
+
|
247 |
+
def forward(self, x: torch.Tensor):
|
248 |
+
orig_type = x.dtype
|
249 |
+
x = F.layer_norm(x, self.normalized_shape, self.weight, self.bias, self.eps)
|
250 |
+
return x.to(orig_type)
|
251 |
+
|
252 |
+
|
253 |
+
class QuickGELU(nn.Module):
|
254 |
+
# NOTE This is slower than nn.GELU or nn.SiLU and uses more GPU memory
|
255 |
+
def forward(self, x: torch.Tensor):
|
256 |
+
return x * torch.sigmoid(1.702 * x)
|
257 |
+
|
258 |
+
|
259 |
+
class ResidualAttentionBlock(nn.Module):
|
260 |
+
def __init__(self, d_model: int, n_head: int, act_layer: Callable = nn.GELU):
|
261 |
+
super().__init__()
|
262 |
+
|
263 |
+
self.attn = nn.MultiheadAttention(d_model, n_head)
|
264 |
+
self.ln_1 = LayerNorm(d_model)
|
265 |
+
self.mlp = nn.Sequential(
|
266 |
+
OrderedDict(
|
267 |
+
[
|
268 |
+
("c_fc", nn.Linear(d_model, d_model * 4)),
|
269 |
+
("gelu", act_layer()),
|
270 |
+
("c_proj", nn.Linear(d_model * 4, d_model)),
|
271 |
+
]
|
272 |
+
)
|
273 |
+
)
|
274 |
+
self.ln_2 = LayerNorm(d_model)
|
275 |
+
|
276 |
+
def attention(self, x: torch.Tensor, attn_mask: Optional[torch.Tensor] = None):
|
277 |
+
return self.attn(x, x, x, need_weights=False, attn_mask=attn_mask)[0]
|
278 |
+
|
279 |
+
def forward(self, x: torch.Tensor, attn_mask: Optional[torch.Tensor] = None):
|
280 |
+
x = x + self.attention(self.ln_1(x), attn_mask=attn_mask)
|
281 |
+
x = x + self.mlp(self.ln_2(x))
|
282 |
+
return x
|
283 |
+
|
284 |
+
|
285 |
+
class Transformer(nn.Module):
|
286 |
+
def __init__(
|
287 |
+
self, width: int, layers: int, heads: int, act_layer: Callable = nn.GELU
|
288 |
+
):
|
289 |
+
super().__init__()
|
290 |
+
self.width = width
|
291 |
+
self.layers = layers
|
292 |
+
self.resblocks = nn.ModuleList(
|
293 |
+
[
|
294 |
+
ResidualAttentionBlock(width, heads, act_layer=act_layer)
|
295 |
+
for _ in range(layers)
|
296 |
+
]
|
297 |
+
)
|
298 |
+
|
299 |
+
def forward(self, x: torch.Tensor, attn_mask: Optional[torch.Tensor] = None):
|
300 |
+
for r in self.resblocks:
|
301 |
+
x = r(x, attn_mask=attn_mask)
|
302 |
+
return x
|
303 |
+
|
304 |
+
|
305 |
+
class VisualTransformer(nn.Module):
|
306 |
+
def __init__(
|
307 |
+
self,
|
308 |
+
image_size: int,
|
309 |
+
patch_size: int,
|
310 |
+
width: int,
|
311 |
+
layers: int,
|
312 |
+
heads: int,
|
313 |
+
output_dim: int,
|
314 |
+
act_layer: Callable = nn.GELU,
|
315 |
+
):
|
316 |
+
super().__init__()
|
317 |
+
self.image_size = image_size
|
318 |
+
self.output_dim = output_dim
|
319 |
+
self.conv1 = nn.Conv2d(
|
320 |
+
in_channels=3,
|
321 |
+
out_channels=width,
|
322 |
+
kernel_size=patch_size,
|
323 |
+
stride=patch_size,
|
324 |
+
bias=False,
|
325 |
+
)
|
326 |
+
|
327 |
+
scale = width**-0.5
|
328 |
+
self.class_embedding = nn.Parameter(scale * torch.randn(width))
|
329 |
+
self.positional_embedding = nn.Parameter(
|
330 |
+
scale * torch.randn((image_size // patch_size) ** 2 + 1, width)
|
331 |
+
)
|
332 |
+
self.ln_pre = LayerNorm(width)
|
333 |
+
|
334 |
+
self.text_branch = Transformer(width, layers, heads, act_layer=act_layer)
|
335 |
+
|
336 |
+
self.ln_post = LayerNorm(width)
|
337 |
+
self.proj = nn.Parameter(scale * torch.randn(width, output_dim))
|
338 |
+
|
339 |
+
def lock(self, unlocked_groups=0, freeze_bn_stats=False):
|
340 |
+
assert (
|
341 |
+
unlocked_groups == 0
|
342 |
+
), "partial locking not currently supported for this model"
|
343 |
+
for param in self.parameters():
|
344 |
+
param.requires_grad = False
|
345 |
+
|
346 |
+
def forward(self, x: torch.Tensor):
|
347 |
+
x = self.conv1(x) # shape = [*, width, grid, grid]
|
348 |
+
x = x.reshape(x.shape[0], x.shape[1], -1) # shape = [*, width, grid ** 2]
|
349 |
+
x = x.permute(0, 2, 1) # shape = [*, grid ** 2, width]
|
350 |
+
x = torch.cat(
|
351 |
+
[
|
352 |
+
self.class_embedding.to(x.dtype)
|
353 |
+
+ torch.zeros(
|
354 |
+
x.shape[0], 1, x.shape[-1], dtype=x.dtype, device=x.device
|
355 |
+
),
|
356 |
+
x,
|
357 |
+
],
|
358 |
+
dim=1,
|
359 |
+
) # shape = [*, grid ** 2 + 1, width]
|
360 |
+
x = x + self.positional_embedding.to(x.dtype)
|
361 |
+
x = self.ln_pre(x)
|
362 |
+
|
363 |
+
x = x.permute(1, 0, 2) # NLD -> LND
|
364 |
+
x = self.text_branch(x)
|
365 |
+
x = x.permute(1, 0, 2) # LND -> NLD
|
366 |
+
|
367 |
+
x = self.ln_post(x[:, 0, :])
|
368 |
+
|
369 |
+
if self.proj is not None:
|
370 |
+
x = x @ self.proj
|
371 |
+
|
372 |
+
return x
|
373 |
+
|
374 |
+
|
375 |
+
@dataclass
|
376 |
+
class CLAPVisionCfg:
|
377 |
+
layers: Union[Tuple[int, int, int, int], int] = 12
|
378 |
+
width: int = 768
|
379 |
+
patch_size: int = 16
|
380 |
+
image_size: Union[Tuple[int, int], int] = 224
|
381 |
+
timm_model_name: str = (
|
382 |
+
None # a valid model name overrides layers, width, patch_size
|
383 |
+
)
|
384 |
+
timm_model_pretrained: bool = (
|
385 |
+
False # use (imagenet) pretrained weights for named model
|
386 |
+
)
|
387 |
+
timm_pool: str = (
|
388 |
+
"avg" # feature pooling for timm model ('abs_attn', 'rot_attn', 'avg', '')
|
389 |
+
)
|
390 |
+
timm_proj: str = (
|
391 |
+
"linear" # linear projection for timm model output ('linear', 'mlp', '')
|
392 |
+
)
|
393 |
+
|
394 |
+
|
395 |
+
# Audio Config Class
|
396 |
+
@dataclass
|
397 |
+
class CLAPAudioCfp:
|
398 |
+
model_type: str = "PANN"
|
399 |
+
model_name: str = "Cnn14"
|
400 |
+
sample_rate: int = 48000
|
401 |
+
# Param
|
402 |
+
audio_length: int = 1024
|
403 |
+
window_size: int = 1024
|
404 |
+
hop_size: int = 1024
|
405 |
+
fmin: int = 50
|
406 |
+
fmax: int = 14000
|
407 |
+
class_num: int = 527
|
408 |
+
mel_bins: int = 64
|
409 |
+
clip_samples: int = 480000
|
410 |
+
|
411 |
+
|
412 |
+
@dataclass
|
413 |
+
class CLAPTextCfg:
|
414 |
+
context_length: int
|
415 |
+
vocab_size: int
|
416 |
+
width: int
|
417 |
+
heads: int
|
418 |
+
layers: int
|
419 |
+
model_type: str
|
420 |
+
|
421 |
+
|
422 |
+
class CLAP(nn.Module):
|
423 |
+
def __init__(
|
424 |
+
self,
|
425 |
+
embed_dim: int,
|
426 |
+
audio_cfg: CLAPAudioCfp,
|
427 |
+
text_cfg: CLAPTextCfg,
|
428 |
+
quick_gelu: bool = False,
|
429 |
+
enable_fusion: bool = False,
|
430 |
+
fusion_type: str = "None",
|
431 |
+
joint_embed_shape: int = 512,
|
432 |
+
mlp_act: str = "relu",
|
433 |
+
):
|
434 |
+
super().__init__()
|
435 |
+
if isinstance(audio_cfg, dict):
|
436 |
+
audio_cfg = CLAPAudioCfp(**audio_cfg)
|
437 |
+
if isinstance(text_cfg, dict):
|
438 |
+
text_cfg = CLAPTextCfg(**text_cfg)
|
439 |
+
|
440 |
+
self.audio_cfg = audio_cfg
|
441 |
+
self.text_cfg = text_cfg
|
442 |
+
self.enable_fusion = enable_fusion
|
443 |
+
self.fusion_type = fusion_type
|
444 |
+
self.joint_embed_shape = joint_embed_shape
|
445 |
+
self.mlp_act = mlp_act
|
446 |
+
|
447 |
+
self.context_length = text_cfg.context_length
|
448 |
+
|
449 |
+
# OpenAI models are pretrained w/ QuickGELU but native nn.GELU is both faster and more
|
450 |
+
# memory efficient in recent PyTorch releases (>= 1.10).
|
451 |
+
# NOTE: timm models always use native GELU regardless of quick_gelu flag.
|
452 |
+
act_layer = QuickGELU if quick_gelu else nn.GELU
|
453 |
+
|
454 |
+
if mlp_act == "relu":
|
455 |
+
mlp_act_layer = nn.ReLU()
|
456 |
+
elif mlp_act == "gelu":
|
457 |
+
mlp_act_layer = nn.GELU()
|
458 |
+
else:
|
459 |
+
raise NotImplementedError
|
460 |
+
|
461 |
+
# audio branch
|
462 |
+
# audio branch parameters
|
463 |
+
if audio_cfg.model_type == "PANN":
|
464 |
+
self.audio_branch = create_pann_model(audio_cfg, enable_fusion, fusion_type)
|
465 |
+
elif audio_cfg.model_type == "HTSAT":
|
466 |
+
self.audio_branch = create_htsat_model(
|
467 |
+
audio_cfg, enable_fusion, fusion_type
|
468 |
+
)
|
469 |
+
else:
|
470 |
+
logging.error(f"Model config for {audio_cfg.model_type} not found")
|
471 |
+
raise RuntimeError(f"Model config for {audio_cfg.model_type} not found.")
|
472 |
+
|
473 |
+
# text branch
|
474 |
+
# text branch parameters
|
475 |
+
if text_cfg.model_type == "transformer":
|
476 |
+
self.text_branch = Transformer(
|
477 |
+
width=text_cfg.width,
|
478 |
+
layers=text_cfg.layers,
|
479 |
+
heads=text_cfg.heads,
|
480 |
+
act_layer=act_layer,
|
481 |
+
)
|
482 |
+
self.vocab_size = text_cfg.vocab_size
|
483 |
+
self.token_embedding = nn.Embedding(text_cfg.vocab_size, text_cfg.width)
|
484 |
+
self.positional_embedding = nn.Parameter(
|
485 |
+
torch.empty(self.context_length, text_cfg.width)
|
486 |
+
)
|
487 |
+
self.ln_final = LayerNorm(text_cfg.width)
|
488 |
+
self.text_transform = MLPLayers(
|
489 |
+
units=[
|
490 |
+
self.joint_embed_shape,
|
491 |
+
self.joint_embed_shape,
|
492 |
+
self.joint_embed_shape,
|
493 |
+
],
|
494 |
+
dropout=0.1,
|
495 |
+
)
|
496 |
+
self.text_projection = nn.Sequential(
|
497 |
+
nn.Linear(text_cfg.width, self.joint_embed_shape),
|
498 |
+
mlp_act_layer,
|
499 |
+
nn.Linear(self.joint_embed_shape, self.joint_embed_shape),
|
500 |
+
)
|
501 |
+
elif text_cfg.model_type == "bert":
|
502 |
+
self.text_branch = BertModel.from_pretrained("bert-base-uncased")
|
503 |
+
self.text_transform = MLPLayers(
|
504 |
+
units=[
|
505 |
+
self.joint_embed_shape,
|
506 |
+
self.joint_embed_shape,
|
507 |
+
self.joint_embed_shape,
|
508 |
+
],
|
509 |
+
dropout=0.1,
|
510 |
+
)
|
511 |
+
self.text_projection = nn.Sequential(
|
512 |
+
nn.Linear(768, self.joint_embed_shape),
|
513 |
+
mlp_act_layer,
|
514 |
+
nn.Linear(self.joint_embed_shape, self.joint_embed_shape),
|
515 |
+
)
|
516 |
+
elif text_cfg.model_type == "roberta":
|
517 |
+
self.text_branch = RobertaModel.from_pretrained("roberta-base")
|
518 |
+
self.text_transform = MLPLayers(
|
519 |
+
units=[
|
520 |
+
self.joint_embed_shape,
|
521 |
+
self.joint_embed_shape,
|
522 |
+
self.joint_embed_shape,
|
523 |
+
],
|
524 |
+
dropout=0.1,
|
525 |
+
)
|
526 |
+
self.text_projection = nn.Sequential(
|
527 |
+
nn.Linear(768, self.joint_embed_shape),
|
528 |
+
mlp_act_layer,
|
529 |
+
nn.Linear(self.joint_embed_shape, self.joint_embed_shape),
|
530 |
+
)
|
531 |
+
elif text_cfg.model_type == "bart":
|
532 |
+
self.text_branch = BartModel.from_pretrained("facebook/bart-base")
|
533 |
+
self.text_transform = MLPLayers(
|
534 |
+
units=[
|
535 |
+
self.joint_embed_shape,
|
536 |
+
self.joint_embed_shape,
|
537 |
+
self.joint_embed_shape,
|
538 |
+
],
|
539 |
+
dropout=0.1,
|
540 |
+
)
|
541 |
+
self.text_projection = nn.Sequential(
|
542 |
+
nn.Linear(768, self.joint_embed_shape),
|
543 |
+
mlp_act_layer,
|
544 |
+
nn.Linear(self.joint_embed_shape, self.joint_embed_shape),
|
545 |
+
)
|
546 |
+
else:
|
547 |
+
logging.error(f"Model config for {text_cfg.model_type} not found")
|
548 |
+
raise RuntimeError(f"Model config for {text_cfg.model_type} not found.")
|
549 |
+
self.text_branch_type = text_cfg.model_type
|
550 |
+
# text branch parameters
|
551 |
+
|
552 |
+
# audio branch parameters
|
553 |
+
self.audio_transform = MLPLayers(
|
554 |
+
units=[
|
555 |
+
self.joint_embed_shape,
|
556 |
+
self.joint_embed_shape,
|
557 |
+
self.joint_embed_shape,
|
558 |
+
],
|
559 |
+
dropout=0.1,
|
560 |
+
)
|
561 |
+
|
562 |
+
# below here is text branch parameters
|
563 |
+
|
564 |
+
# ============================================================================================================
|
565 |
+
self.audio_projection = nn.Sequential(
|
566 |
+
nn.Linear(embed_dim, self.joint_embed_shape),
|
567 |
+
mlp_act_layer,
|
568 |
+
nn.Linear(self.joint_embed_shape, self.joint_embed_shape),
|
569 |
+
)
|
570 |
+
|
571 |
+
self.logit_scale_a = nn.Parameter(torch.ones([]) * np.log(1 / 0.07))
|
572 |
+
self.logit_scale_t = nn.Parameter(torch.ones([]) * np.log(1 / 0.07))
|
573 |
+
self.register_buffer("attn_mask", self.build_attention_mask(), persistent=False)
|
574 |
+
|
575 |
+
self.init_text_branch_parameters()
|
576 |
+
|
577 |
+
def init_text_branch_parameters(self):
|
578 |
+
if self.text_branch_type == "transformer":
|
579 |
+
nn.init.normal_(self.token_embedding.weight, std=0.02)
|
580 |
+
nn.init.normal_(self.positional_embedding, std=0.01)
|
581 |
+
proj_std = (self.text_branch.width**-0.5) * (
|
582 |
+
(2 * self.text_branch.layers) ** -0.5
|
583 |
+
)
|
584 |
+
attn_std = self.text_branch.width**-0.5
|
585 |
+
fc_std = (2 * self.text_branch.width) ** -0.5
|
586 |
+
for block in self.text_branch.resblocks:
|
587 |
+
nn.init.normal_(block.attn.in_proj_weight, std=attn_std)
|
588 |
+
nn.init.normal_(block.attn.out_proj.weight, std=proj_std)
|
589 |
+
nn.init.normal_(block.mlp.c_fc.weight, std=fc_std)
|
590 |
+
nn.init.normal_(block.mlp.c_proj.weight, std=proj_std)
|
591 |
+
if self.text_branch_type == "bert" or self.text_branch_type == "roberta":
|
592 |
+
width = self.text_branch.embeddings.word_embeddings.weight.shape[-1]
|
593 |
+
elif self.text_branch_type == "bart":
|
594 |
+
width = self.text_branch.shared.weight.shape[-1]
|
595 |
+
else:
|
596 |
+
width = self.text_branch.width
|
597 |
+
nn.init.constant_(self.logit_scale_a, np.log(1 / 0.07))
|
598 |
+
nn.init.constant_(self.logit_scale_t, np.log(1 / 0.07))
|
599 |
+
|
600 |
+
# deprecated
|
601 |
+
# if hasattr(self.visual, 'init_parameters'):
|
602 |
+
# self.visual.init_parameters()
|
603 |
+
|
604 |
+
# if self.text_projection is not None:
|
605 |
+
# nn.init.normal_(self.text_projection, std=width**-0.5)
|
606 |
+
|
607 |
+
def build_attention_mask(self):
|
608 |
+
# lazily create causal attention mask, with full attention between the vision tokens
|
609 |
+
# pytorch uses additive attention mask; fill with -inf
|
610 |
+
mask = torch.empty(self.context_length, self.context_length)
|
611 |
+
mask.fill_(float("-inf"))
|
612 |
+
mask.triu_(1) # zero out the lower diagonal
|
613 |
+
return mask
|
614 |
+
|
615 |
+
def encode_audio(self, audio, device):
|
616 |
+
return self.audio_branch(
|
617 |
+
audio, mixup_lambda=None, device=device
|
618 |
+
) # mix lambda needs to add
|
619 |
+
|
620 |
+
# def list_of_dict_of_tensor2dict_of_tensor(self, x, device):
|
621 |
+
# tmp = {}
|
622 |
+
# for k in x[0].keys():
|
623 |
+
# tmp[k] = []
|
624 |
+
# for i in range(len(x)):
|
625 |
+
# tmp[k].append(x[i][k][:77])
|
626 |
+
# for k in x[0].keys():
|
627 |
+
# tmp[k] = torch.tensor(tmp[k]).to(device=device, non_blocking=True)
|
628 |
+
# return tmp
|
629 |
+
|
630 |
+
def encode_text(self, text, device):
|
631 |
+
if self.text_branch_type == "transformer":
|
632 |
+
text = text.to(device=device, non_blocking=True)
|
633 |
+
x = self.token_embedding(text) # [batch_size, n_ctx, d_model]
|
634 |
+
|
635 |
+
x = x + self.positional_embedding
|
636 |
+
x = x.permute(1, 0, 2) # NLD -> LND
|
637 |
+
x = self.text_branch(x, attn_mask=self.attn_mask)
|
638 |
+
x = x.permute(1, 0, 2) # LND -> NLD
|
639 |
+
x = self.ln_final(x)
|
640 |
+
|
641 |
+
# x.shape = [batch_size, n_ctx, transformer.width]
|
642 |
+
# take features from the eot embedding (eot_token is the highest number in each sequence)
|
643 |
+
x = self.text_projection(x[torch.arange(x.shape[0]), text.argmax(dim=-1)])
|
644 |
+
elif self.text_branch_type == "bert":
|
645 |
+
# text = self.list_of_dict_of_tensor2dict_of_tensor(text, device)
|
646 |
+
# text = BatchEncoding(text)
|
647 |
+
x = self.text_branch(
|
648 |
+
input_ids=text["input_ids"].to(device=device, non_blocking=True),
|
649 |
+
attention_mask=text["attention_mask"].to(
|
650 |
+
device=device, non_blocking=True
|
651 |
+
),
|
652 |
+
token_type_ids=text["token_type_ids"].to(
|
653 |
+
device=device, non_blocking=True
|
654 |
+
),
|
655 |
+
)["pooler_output"]
|
656 |
+
x = self.text_projection(x)
|
657 |
+
elif self.text_branch_type == "roberta":
|
658 |
+
x = self.text_branch(
|
659 |
+
input_ids=text["input_ids"].to(device=device, non_blocking=True),
|
660 |
+
attention_mask=text["attention_mask"].to(
|
661 |
+
device=device, non_blocking=True
|
662 |
+
),
|
663 |
+
)["pooler_output"]
|
664 |
+
x = self.text_projection(x)
|
665 |
+
elif self.text_branch_type == "bart":
|
666 |
+
x = torch.mean(
|
667 |
+
self.text_branch(
|
668 |
+
input_ids=text["input_ids"].to(device=device, non_blocking=True),
|
669 |
+
attention_mask=text["attention_mask"].to(
|
670 |
+
device=device, non_blocking=True
|
671 |
+
),
|
672 |
+
)["encoder_last_hidden_state"],
|
673 |
+
axis=1,
|
674 |
+
)
|
675 |
+
x = self.text_projection(x)
|
676 |
+
else:
|
677 |
+
logging.error(f"Model type {self.text_branch_type} not found")
|
678 |
+
raise RuntimeError(f"Model type {self.text_branch_type} not found.")
|
679 |
+
return x
|
680 |
+
|
681 |
+
def forward(self, audio, text, device=None):
|
682 |
+
"""Forward audio and text into the CLAP
|
683 |
+
|
684 |
+
Parameters
|
685 |
+
----------
|
686 |
+
audio: torch.Tensor (batch_size, audio_length)
|
687 |
+
the time-domain audio input / the batch of mel_spec and longer list.
|
688 |
+
text: torch.Tensor () // need to add
|
689 |
+
the text token input
|
690 |
+
"""
|
691 |
+
if device is None:
|
692 |
+
if audio is not None:
|
693 |
+
device = audio.device
|
694 |
+
elif text is not None:
|
695 |
+
device = text.device
|
696 |
+
if audio is None and text is None:
|
697 |
+
# a hack to get the logit scale
|
698 |
+
return self.logit_scale_a.exp(), self.logit_scale_t.exp()
|
699 |
+
elif audio is None:
|
700 |
+
return self.encode_text(text, device=device)
|
701 |
+
elif text is None:
|
702 |
+
return self.audio_projection(
|
703 |
+
self.encode_audio(audio, device=device)["embedding"]
|
704 |
+
)
|
705 |
+
audio_features = self.audio_projection(
|
706 |
+
self.encode_audio(audio, device=device)["embedding"]
|
707 |
+
)
|
708 |
+
audio_features = F.normalize(audio_features, dim=-1)
|
709 |
+
|
710 |
+
text_features = self.encode_text(text, device=device)
|
711 |
+
# print("text_features", text_features)
|
712 |
+
# print("text_features.shape", text_features.shape)
|
713 |
+
# print("text_features.type", type(text_features))
|
714 |
+
text_features = F.normalize(text_features, dim=-1)
|
715 |
+
|
716 |
+
audio_features_mlp = self.audio_transform(audio_features)
|
717 |
+
text_features_mlp = self.text_transform(text_features)
|
718 |
+
# Four outputs: audio features (basic & MLP), text features (basic & MLP)
|
719 |
+
return (
|
720 |
+
audio_features,
|
721 |
+
text_features,
|
722 |
+
audio_features_mlp,
|
723 |
+
text_features_mlp,
|
724 |
+
self.logit_scale_a.exp(),
|
725 |
+
self.logit_scale_t.exp(),
|
726 |
+
)
|
727 |
+
|
728 |
+
def get_logit_scale(self):
|
729 |
+
return self.logit_scale_a.exp(), self.logit_scale_t.exp()
|
730 |
+
|
731 |
+
def get_text_embedding(self, data):
|
732 |
+
"""Get the text embedding from the model
|
733 |
+
|
734 |
+
Parameters
|
735 |
+
----------
|
736 |
+
data: torch.Tensor
|
737 |
+
a tensor of text embedding
|
738 |
+
|
739 |
+
Returns
|
740 |
+
----------
|
741 |
+
text_embed: torch.Tensor
|
742 |
+
a tensor of text_embeds (N, D)
|
743 |
+
|
744 |
+
"""
|
745 |
+
device = next(self.parameters()).device
|
746 |
+
for k in data:
|
747 |
+
data[k] = data[k].to(device)
|
748 |
+
if len(data[k].size()) < 2:
|
749 |
+
data[k] = data[k].unsqueeze(0)
|
750 |
+
text_embeds = self.encode_text(data, device=device)
|
751 |
+
text_embeds = F.normalize(text_embeds, dim=-1)
|
752 |
+
|
753 |
+
return text_embeds
|
754 |
+
|
755 |
+
def get_audio_embedding(self, data):
|
756 |
+
"""Get the audio embedding from the model
|
757 |
+
|
758 |
+
Parameters
|
759 |
+
----------
|
760 |
+
data: a list of dict
|
761 |
+
the audio input dict list from 'get_audio_feature' method
|
762 |
+
|
763 |
+
Returns
|
764 |
+
----------
|
765 |
+
audio_embed: torch.Tensor
|
766 |
+
a tensor of audio_embeds (N, D)
|
767 |
+
|
768 |
+
"""
|
769 |
+
device = next(self.parameters()).device
|
770 |
+
input_dict = {}
|
771 |
+
keys = data[0].keys()
|
772 |
+
for k in keys:
|
773 |
+
input_dict[k] = torch.cat([d[k].unsqueeze(0) for d in data], dim=0).to(
|
774 |
+
device
|
775 |
+
)
|
776 |
+
|
777 |
+
audio_embeds = self.audio_projection(
|
778 |
+
self.encode_audio(input_dict, device=device)["embedding"]
|
779 |
+
)
|
780 |
+
audio_embeds = F.normalize(audio_embeds, dim=-1)
|
781 |
+
|
782 |
+
return audio_embeds
|
783 |
+
|
784 |
+
def audio_infer(self, audio, hopsize=None, device=None):
|
785 |
+
"""Forward one audio and produce the audio embedding
|
786 |
+
|
787 |
+
Parameters
|
788 |
+
----------
|
789 |
+
audio: (audio_length)
|
790 |
+
the time-domain audio input, notice that it must be only one input
|
791 |
+
hopsize: int
|
792 |
+
the overlap hopsize as the sliding window
|
793 |
+
|
794 |
+
Returns
|
795 |
+
----------
|
796 |
+
output_dict: {
|
797 |
+
key: [n, (embedding_shape)] if "HTS-AT"
|
798 |
+
or
|
799 |
+
key: [(embedding_shape)] if "PANN"
|
800 |
+
}
|
801 |
+
the list of key values of the audio branch
|
802 |
+
|
803 |
+
"""
|
804 |
+
|
805 |
+
assert not self.training, "the inference mode must be run at eval stage"
|
806 |
+
output_dict = {}
|
807 |
+
# PANN
|
808 |
+
if self.audio_cfg.model_type == "PANN":
|
809 |
+
audio_input = audio.unsqueeze(dim=0)
|
810 |
+
output_dict[key] = self.encode_audio(audio_input, device=device)[
|
811 |
+
key
|
812 |
+
].squeeze(dim=0)
|
813 |
+
elif self.audio_cfg.model_type == "HTSAT":
|
814 |
+
# repeat
|
815 |
+
audio_len = len(audio)
|
816 |
+
k = self.audio_cfg.clip_samples // audio_len
|
817 |
+
if k > 1:
|
818 |
+
audio = audio.repeat(k)
|
819 |
+
audio_len = len(audio)
|
820 |
+
|
821 |
+
if hopsize is None:
|
822 |
+
hopsize = min(hopsize, audio_len)
|
823 |
+
|
824 |
+
if audio_len > self.audio_cfg.clip_samples:
|
825 |
+
audio_input = [
|
826 |
+
audio[pos : pos + self.audio_cfg.clip_samples].clone()
|
827 |
+
for pos in range(
|
828 |
+
0, audio_len - self.audio_cfg.clip_samples, hopsize
|
829 |
+
)
|
830 |
+
]
|
831 |
+
audio_input.append(audio[-self.audio_cfg.clip_samples :].clone())
|
832 |
+
audio_input = torch.stack(audio_input)
|
833 |
+
output_dict[key] = self.encode_audio(audio_input, device=device)[key]
|
834 |
+
else:
|
835 |
+
audio_input = audio.unsqueeze(dim=0)
|
836 |
+
output_dict[key] = self.encode_audio(audio_input, device=device)[
|
837 |
+
key
|
838 |
+
].squeeze(dim=0)
|
839 |
+
|
840 |
+
return output_dict
|
841 |
+
|
842 |
+
|
843 |
+
def convert_weights_to_fp16(model: nn.Module):
|
844 |
+
"""Convert applicable model parameters to fp16"""
|
845 |
+
|
846 |
+
def _convert_weights_to_fp16(l):
|
847 |
+
if isinstance(l, (nn.Conv1d, nn.Conv2d, nn.Linear)):
|
848 |
+
l.weight.data = l.weight.data.half()
|
849 |
+
if l.bias is not None:
|
850 |
+
l.bias.data = l.bias.data.half()
|
851 |
+
|
852 |
+
if isinstance(l, nn.MultiheadAttention):
|
853 |
+
for attr in [
|
854 |
+
*[f"{s}_proj_weight" for s in ["in", "q", "k", "v"]],
|
855 |
+
"in_proj_bias",
|
856 |
+
"bias_k",
|
857 |
+
"bias_v",
|
858 |
+
]:
|
859 |
+
tensor = getattr(l, attr)
|
860 |
+
if tensor is not None:
|
861 |
+
tensor.data = tensor.data.half()
|
862 |
+
|
863 |
+
for name in ["text_projection", "proj"]:
|
864 |
+
if hasattr(l, name):
|
865 |
+
attr = getattr(l, name)
|
866 |
+
if attr is not None:
|
867 |
+
attr.data = attr.data.half()
|
868 |
+
|
869 |
+
model.apply(_convert_weights_to_fp16)
|
870 |
+
|
871 |
+
|
872 |
+
# Ignore the state dict of the vision part
|
873 |
+
def build_model_from_openai_state_dict(
|
874 |
+
state_dict: dict, model_cfg, enable_fusion: bool = False, fusion_type: str = "None"
|
875 |
+
):
|
876 |
+
|
877 |
+
embed_dim = model_cfg["embed_dim"]
|
878 |
+
audio_cfg = model_cfg["audio_cfg"]
|
879 |
+
text_cfg = model_cfg["text_cfg"]
|
880 |
+
context_length = state_dict["positional_embedding"].shape[0]
|
881 |
+
vocab_size = state_dict["token_embedding.weight"].shape[0]
|
882 |
+
transformer_width = state_dict["ln_final.weight"].shape[0]
|
883 |
+
transformer_heads = transformer_width // 64
|
884 |
+
transformer_layers = len(
|
885 |
+
set(
|
886 |
+
k.split(".")[2]
|
887 |
+
for k in state_dict
|
888 |
+
if k.startswith(f"transformer.resblocks")
|
889 |
+
)
|
890 |
+
)
|
891 |
+
|
892 |
+
audio_cfg = CLAPAudioCfp(**audio_cfg)
|
893 |
+
text_cfg = CLAPTextCfg(**text_cfg)
|
894 |
+
|
895 |
+
model = CLAP(
|
896 |
+
embed_dim,
|
897 |
+
audio_cfg=audio_cfg,
|
898 |
+
text_cfg=text_cfg,
|
899 |
+
quick_gelu=True, # OpenAI models were trained with QuickGELU
|
900 |
+
enable_fusion=enable_fusion,
|
901 |
+
fusion_type=fusion_type,
|
902 |
+
)
|
903 |
+
state_dict["logit_scale_a"] = state_dict["logit_scale"]
|
904 |
+
state_dict["logit_scale_t"] = state_dict["logit_scale"]
|
905 |
+
pop_keys = list(state_dict.keys())[::]
|
906 |
+
# pop the visual branch saved weights
|
907 |
+
for key in pop_keys:
|
908 |
+
if key.startswith("visual."):
|
909 |
+
state_dict.pop(key, None)
|
910 |
+
|
911 |
+
for key in ["logit_scale", "input_resolution", "context_length", "vocab_size"]:
|
912 |
+
state_dict.pop(key, None)
|
913 |
+
|
914 |
+
# not use fp16
|
915 |
+
# convert_weights_to_fp16(model)
|
916 |
+
model.load_state_dict(state_dict, strict=False)
|
917 |
+
return model.eval()
|
918 |
+
|
919 |
+
|
920 |
+
def trace_model(model, batch_size=256, device=torch.device("cpu")):
|
921 |
+
model.eval()
|
922 |
+
audio_length = model.audio_cfg.audio_length
|
923 |
+
example_audio = torch.ones((batch_size, audio_length), device=device)
|
924 |
+
example_text = torch.zeros(
|
925 |
+
(batch_size, model.context_length), dtype=torch.int, device=device
|
926 |
+
)
|
927 |
+
model = torch.jit.trace_module(
|
928 |
+
model,
|
929 |
+
inputs=dict(
|
930 |
+
forward=(example_audio, example_text),
|
931 |
+
encode_text=(example_text,),
|
932 |
+
encode_image=(example_audio,),
|
933 |
+
),
|
934 |
+
)
|
935 |
+
model.audio_cfg.audio_length = audio_length # Question: what does this do?
|
936 |
+
return model
|
src/audioldm/clap/open_clip/model_configs/HTSAT-base.json
ADDED
@@ -0,0 +1,23 @@
|
|
|
|
|
|
|
|
|
|
|
|
|
|
|
|
|
|
|
|
|
|
|
|
|
|
|
|
|
|
|
|
|
|
|
|
|
|
|
|
|
|
|
|
|
|
|
|
|
1 |
+
{
|
2 |
+
"embed_dim": 1024,
|
3 |
+
"audio_cfg": {
|
4 |
+
"audio_length": 1024,
|
5 |
+
"clip_samples": 480000,
|
6 |
+
"mel_bins": 64,
|
7 |
+
"sample_rate": 48000,
|
8 |
+
"window_size": 1024,
|
9 |
+
"hop_size": 480,
|
10 |
+
"fmin": 50,
|
11 |
+
"fmax": 14000,
|
12 |
+
"class_num": 527,
|
13 |
+
"model_type": "HTSAT",
|
14 |
+
"model_name": "base"
|
15 |
+
},
|
16 |
+
"text_cfg": {
|
17 |
+
"context_length": 77,
|
18 |
+
"vocab_size": 49408,
|
19 |
+
"width": 512,
|
20 |
+
"heads": 8,
|
21 |
+
"layers": 12
|
22 |
+
}
|
23 |
+
}
|
src/audioldm/clap/open_clip/model_configs/HTSAT-large.json
ADDED
@@ -0,0 +1,23 @@
|
|
|
|
|
|
|
|
|
|
|
|
|
|
|
|
|
|
|
|
|
|
|
|
|
|
|
|
|
|
|
|
|
|
|
|
|
|
|
|
|
|
|
|
|
|
|
|
|
1 |
+
{
|
2 |
+
"embed_dim": 2048,
|
3 |
+
"audio_cfg": {
|
4 |
+
"audio_length": 1024,
|
5 |
+
"clip_samples": 480000,
|
6 |
+
"mel_bins": 64,
|
7 |
+
"sample_rate": 48000,
|
8 |
+
"window_size": 1024,
|
9 |
+
"hop_size": 480,
|
10 |
+
"fmin": 50,
|
11 |
+
"fmax": 14000,
|
12 |
+
"class_num": 527,
|
13 |
+
"model_type": "HTSAT",
|
14 |
+
"model_name": "large"
|
15 |
+
},
|
16 |
+
"text_cfg": {
|
17 |
+
"context_length": 77,
|
18 |
+
"vocab_size": 49408,
|
19 |
+
"width": 512,
|
20 |
+
"heads": 8,
|
21 |
+
"layers": 12
|
22 |
+
}
|
23 |
+
}
|
src/audioldm/clap/open_clip/model_configs/HTSAT-tiny-win-1536.json
ADDED
@@ -0,0 +1,23 @@
|
|
|
|
|
|
|
|
|
|
|
|
|
|
|
|
|
|
|
|
|
|
|
|
|
|
|
|
|
|
|
|
|
|
|
|
|
|
|
|
|
|
|
|
|
|
|
|
|
1 |
+
{
|
2 |
+
"embed_dim": 768,
|
3 |
+
"audio_cfg": {
|
4 |
+
"audio_length": 1024,
|
5 |
+
"clip_samples": 480000,
|
6 |
+
"mel_bins": 64,
|
7 |
+
"sample_rate": 48000,
|
8 |
+
"window_size": 1536,
|
9 |
+
"hop_size": 480,
|
10 |
+
"fmin": 50,
|
11 |
+
"fmax": 14000,
|
12 |
+
"class_num": 527,
|
13 |
+
"model_type": "HTSAT",
|
14 |
+
"model_name": "tiny"
|
15 |
+
},
|
16 |
+
"text_cfg": {
|
17 |
+
"context_length": 77,
|
18 |
+
"vocab_size": 49408,
|
19 |
+
"width": 512,
|
20 |
+
"heads": 8,
|
21 |
+
"layers": 12
|
22 |
+
}
|
23 |
+
}
|
src/audioldm/clap/open_clip/model_configs/HTSAT-tiny.json
ADDED
@@ -0,0 +1,23 @@
|
|
|
|
|
|
|
|
|
|
|
|
|
|
|
|
|
|
|
|
|
|
|
|
|
|
|
|
|
|
|
|
|
|
|
|
|
|
|
|
|
|
|
|
|
|
|
|
|
1 |
+
{
|
2 |
+
"embed_dim": 768,
|
3 |
+
"audio_cfg": {
|
4 |
+
"audio_length": 1024,
|
5 |
+
"clip_samples": 480000,
|
6 |
+
"mel_bins": 64,
|
7 |
+
"sample_rate": 48000,
|
8 |
+
"window_size": 1024,
|
9 |
+
"hop_size": 480,
|
10 |
+
"fmin": 50,
|
11 |
+
"fmax": 14000,
|
12 |
+
"class_num": 527,
|
13 |
+
"model_type": "HTSAT",
|
14 |
+
"model_name": "tiny"
|
15 |
+
},
|
16 |
+
"text_cfg": {
|
17 |
+
"context_length": 77,
|
18 |
+
"vocab_size": 49408,
|
19 |
+
"width": 512,
|
20 |
+
"heads": 8,
|
21 |
+
"layers": 12
|
22 |
+
}
|
23 |
+
}
|
src/audioldm/clap/open_clip/model_configs/PANN-10.json
ADDED
@@ -0,0 +1,23 @@
|
|
|
|
|
|
|
|
|
|
|
|
|
|
|
|
|
|
|
|
|
|
|
|
|
|
|
|
|
|
|
|
|
|
|
|
|
|
|
|
|
|
|
|
|
|
|
|
|
1 |
+
{
|
2 |
+
"embed_dim": 1024,
|
3 |
+
"audio_cfg": {
|
4 |
+
"audio_length": 1024,
|
5 |
+
"clip_samples": 480000,
|
6 |
+
"mel_bins": 64,
|
7 |
+
"sample_rate": 48000,
|
8 |
+
"window_size": 1024,
|
9 |
+
"hop_size": 480,
|
10 |
+
"fmin": 50,
|
11 |
+
"fmax": 14000,
|
12 |
+
"class_num": 527,
|
13 |
+
"model_type": "PANN",
|
14 |
+
"model_name": "Cnn10"
|
15 |
+
},
|
16 |
+
"text_cfg": {
|
17 |
+
"context_length": 77,
|
18 |
+
"vocab_size": 49408,
|
19 |
+
"width": 512,
|
20 |
+
"heads": 8,
|
21 |
+
"layers": 12
|
22 |
+
}
|
23 |
+
}
|